The power of daydreams: 4 studies on the surprising science of mind-wandering
[ted id=1607 width=560 height=315]
What makes us happy? It’s one of the most complicated puzzles of human existence — and one that, so far, 87 speakers have explored in TEDTalks .
In today’s talk , Matt Killingsworth (who studied under Dan Gilbert at Harvard) shares a novel approach to the study of happiness — an app, Track Your Happiness , which allows people to chart their feelings on a moment-by-moment basis. As they go about their day, app users get random pings, asking them to share their current activity and note their mood. When Killingsworth gave this talk at TEDxCambridge in 2011, the app had collected data from more than 15,000 people in 80 countries, representing a wide range of ages, education levels and occupations. In this talk, Killingsworth reveals a very surprising finding: that mind-wandering appears to factor heavily into this happiness equation.
“As human beings, we have this unique ability to have our minds stray,” says Killingsworth on the TEDx stage . “This ability to focus our attention on something other than the present is amazing — it allows us to learn and plan and reason.”
While most people think of mind-wandering as a lifting escape from daily drudgery, the Track Your Happiness data shows that this may not the case. In fact, mind-wandering appears to be correlated with unhappiness . When people were mind-wandering, they reported feeling happy only 56% of the time. Meanwhile, when they were focused on the present moment, they reported feeling happy 66% of the time. This effect was true regardless of the activity the person was doing — be it waiting in a traffic jam or eating a delicious dinner. (Read Killingsworth’s study, published in the journal Science in 2010 , to see a breakdown of mind-wandering rates by activity.)
According to Killingsworth’s data, people mind-wander most when in the shower and least when they are having sex. But, still, mind-wandering is a constant. Overall, people mind-wander 47% of the time. Perhaps not such a good thing if it relates to unhappiness,
To hear more about mind-wandering — and about the importance of studying happiness in general — watch Killingsworth’s talk . And after the jump, read several more fascinating studies on the psychology of mind-wandering — some of which will make you feel better about your daydreaming.
A relationship to working memory Mind-wandering might make us feel less content, but it could also have a functional purpose. A recent study published in the journal Psychological Science suggests that mind-wandering might be a sign of a high capacity working memory — in other words, the ability to think about multiple things at once. Researchers asked study participants to press a button and, as they went, checked in to see if their minds were wandering. After the task was complete, researchers gave participants a measure of their working memory. Interestingly, those who were found to be frequent mind-wanderers during the first task showed a greater capacity of working memory. Researcher Jonathan Smallwood of the Max Planck Institute for Human Cognitive and Brain Science explains, “Our results suggest that the sorts of planning that people do quite often in daily life — when they’re on the bus, when they’re cycling to work, when they’re in the shower — are probably supported by working memory. Their brains are trying to allocate resources to the most pressing problems.”
A key to memory formation Mind-wandering might also play a vital function in helping us form memories. New York University neuroscientist Arielle Tambini looked at memory consolidation in this study published in the journal Neuron in 2010 . Participants in the study were asked to look at pairs of images and, in between, were instructed to take a break to think about anything they wanted. Using fMRI, the researchers looked at the activity in the hippocampus cortical regions while they did both. The study showed that these two areas of the brain appear to work together — and that the greater the levels of brain activity in both areas, the stronger the subjects’ recall of the image pairing was. Explains Lila Davichi, who oversaw the study , “Your brain is working for you when you’re resting, so rest is important for memory and cognitive function. This is something we don’t appreciate much, especially when today’s information technologies keep us working round-the-clock … Taking a coffee break after class can actually help you retain that information you just learned.”
A creative boost As the cliché goes, the best ideas usually come when you are least expecting them. A recent study published in the journal Psychological Science gives a clue as to why. A research team led by Benjamin Baird and Jonathan Schooler of the University of California at Santa Barbara asked participants to take “unusual uses” tests — brainstorming alternate ways to use an everyday object like a toothpick for two minutes. Study participants did two of these sessions, and then were given a 12-minute break, during which they were asked to rest, perform a demanding memory exercise or do a reaction time activity designed to maximize their mind-wandering. After the break, they did four more unusual uses tests — two of them repeats. While all of the groups performed comparably on the two new unusual uses lists, the group that had performed the mind-wandering tasks performed 41% better then the other groups on the unusual uses lists they were repeating. “The implication is that mind-wandering was only helpful for problems that were already being mentally chewed on. It didn’t seem to lead to a general increase in creative problem-solving ability,” says Baird .
- Subscribe to TED Blog by email

Comments (69)
Pingback: Tracking Mind-Wandering – Resonance
Pingback: 4 studies on the surprising science of mind-wandering | TED Blog | Miquel Blanch
Pingback: 4 Tips For Better Daydreaming / R.S. Mollison-Read
Pingback: TED Talks Pinned: Speaking of HapPINness | The English Learners' Blog
Pingback: momentary happiness please… Ted Talk | Hunt FOR Truth on wordpress
Pingback: On the science of Mind-wandering | Learning Sciences of Change
Pingback: Poetics Serendipity | Margo Roby: Wordgathering
Pingback: Matt Killingsworthy:想要快樂嗎?專注於當下吧! | TEDxTaipei
Pingback: Issue 27 – Happy Thanksgiving! — TLN
Pingback: Abundance. | Cindy Mantai Writing & Editing
Thank you for visiting nature.com. You are using a browser version with limited support for CSS. To obtain the best experience, we recommend you use a more up to date browser (or turn off compatibility mode in Internet Explorer). In the meantime, to ensure continued support, we are displaying the site without styles and JavaScript.
- View all journals
- My Account Login
- Explore content
- About the journal
- Publish with us
- Sign up for alerts
- Open access
- Published: 11 May 2022
On the relationship between mind wandering and mindfulness
- Angelo Belardi 1 ,
- Leila Chaieb 2 ,
- Alodie Rey-Mermet 1 ,
- Florian Mormann 2 ,
- Nicolas Rothen 1 ,
- Juergen Fell 2 &
- Thomas P. Reber 1 , 2
Scientific Reports volume 12 , Article number: 7755 ( 2022 ) Cite this article
4766 Accesses
7 Citations
16 Altmetric
Metrics details
Mind wandering (MW) and mindfulness have both been reported to be vital moderators of psychological wellbeing. Here, we aim to examine how closely associated these phenomena are and evaluate the psychometrics of measures often used to quantify them. We investigated two samples, one consisting of German-speaking unpaid participants (GUP, n \(=\) 313) and one of English-speaking paid participants (EPP, n \(=\) 228) recruited through MTurk.com. In an online experiment, we collected data using the Mindful Attention Awareness Scale (MAAS) and the sustained attention to response task (SART) during which self-reports of MW and meta-awareness of MW were recorded using experience sampling (ES) probes. Internal consistency of the MAAS was high (Cronbachs \(\alpha\) of 0.96 in EPP and 0.88 in GUP). Split-half reliability for SART measures and self-reported MW was overall good with the exception of SART measures focusing on Nogo trials, and those restricted to SART trials preceding ES in a 10 s time window. We found a moderate negative association between trait mindfulness and MW as measured with ES probes in GUP, but not in EPP. Our results suggest that MW and mindfulness are on opposite sides of a spectrum of how attention is focused on the present moment and the task at hand.
Similar content being viewed by others
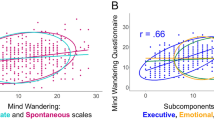
Executive failure hypothesis explains the trait-level association between motivation and mind wandering
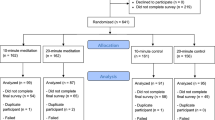
The effect of ten versus twenty minutes of mindfulness meditation on state mindfulness and affect
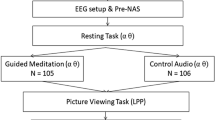
An electrophysiological investigation on the emotion regulatory mechanisms of brief open monitoring meditation in novice non-meditators
Introduction.
Waking experience can be described as a stream of thoughts, perceptions, and emotions that come in and out of the focus of our conscious awareness. Mind wandering (MW) refers to our thoughts becoming decoupled from an ongoing task and coupled to thoughts and feelings not being subject to the task at hand or our surroundings 1 . In comparison, mindfulness refers to the mental act of intentionally resting the focus of awareness on a particular subject of experience in the present moment without judgment 2 . These constructs appear to emphasise aspects which lie on opposite sides of a spectrum of how intentional, focused, and self-aware one is regarding the thoughts and perceptions that make up one’s conscious experience 3 .
In light of these conceptual considerations, it seems surprising that statistical associations between measures of MW and mindfulness are rather low 3 , 4 , 5 . One possible explanation for this may be the low reliability of the psychometric tools used to measure these constructs. Another possibility could be that meta-awareness of MW 6 , 7 , i.e., awareness of the fact that ones contents of consciousness is decoupling from an ongoing task, moderates the relationship between mindfulness and MW. To investigate these questions, we first assessed the psychometrics of a well-established mindfulness questionnaire and self-report measures of MW and meta-awareness thereof in a large sample from an online study. Then we estimated the associations between measures of MW, mindfulness, and meta-awareness.
Evidence for the importance of both MW and mindfulness for psychological wellbeing has been reported numerous times in the literature. Increased propensity to MW was associated with reduced affect 8 and in its extreme form MW can result in persistent negative and repetitive thoughts leading to rumination. Such rumination is at the heart of neurocognitive models of depression 9 , 10 , 11 . Furthermore, distraction due to MW can potentially cause physical harm e.g. when driving 12 , operating heavy machinery 13 , or when working as a medical professional 14 . Excessive MW may also interfere with career goals by affecting work and educational performance 15 . While a majority of studies focus on negative consequences, MW may also facilitate future planning, goal setting, and aid creative problem solving 16 , 17 , 18 . For example, Medea and colleagues 18 found that self-generated cognition during an episode of MW may allow the development of more concrete personal goals.
In contrast, mindfulness has been associated predominantly with an increased feeling of wellbeing. The concept of mindfulness has its origins in eastern philosophy and is closely linked to processes of awareness and attention. Mindfulness describes a state in which a person willingly chooses the focus of conscious experience and takes constant notice of their contents of consciousness. Practicing to achieve this mindful state has been a central tenet of traditional Buddhist meditation, and has been introduced in western cultures as a secular form of mental practice and flavours in psychotherapy, such as e.g., the mindfulness-based stress reduction (MBSR) program or acceptance and commitment therapy 2 , 19 , 20 .
A widely used task to experimentally elicit MW is the sustained attention to response task (SART) 21 . Participants view, for example, a stream of numbers from 0 to 9 appearing in a random sequence and at a constant rate. The participants’ task is to press a button in response to all non-target digits (Go trials) except for one – the target, where they are required to withhold their button press (i.e., the Nogo trial, such as the number 7). Several dependent variables have been used in the SART, such as the performance of the task (i.e., the error rate on Go trials and Nogo trials), the mean reaction time (RT) in Go trials and the variance of these RTs, as well as scores combining performance and RT (e.g. a skills index, calculated as accuracy/RT) 22 . Variants of the task include querying participants intermittently in defined intervals as to whether their mind was ‘on task’ or ‘off task’ using experience sampling (ES) probes to measure MW. Furthermore, meta-awareness of MW is queried after ES of MW in some studies immediately after ‘off task’ responses 23 , 24 . In addition to the self-reports from ES, low performance 25 , 26 , 27 as well as long and widely dispersed RTs 16 in the SART are considered evidence for low sustained attention and potentially for MW. Several versions of the paradigm combining ES probes and SART have been used in previous research. For example, some studies restricted performance and RT analyses to short time windows immediately preceding appearance of the ES probe 16 , 27 . Other studies varied SART difficulty by either adding auditory noise 28 , by making the number stream predictable 29 , or by increasing the inter-stimulus interval (ISI) 30 . Taken together, there is a variety of ways in which the SART is used to elicit and assess MW.
Tools to measure mindfulness, on the other hand, consist predominantly of self-report questionnaires. One of the most commonly used questionnaires is the Mindful Attention Awareness Scale (MAAS) 31 . Previous assessments of the MAAS found that it has a single factor structure and overall robust reliability (Cronbach’s \(\alpha\) between 0.8 and 0.87) 31 . External validity was evaluated with numerous questionnaires assessing a variety of related constructs such as everyday attention, personality traits and anxiety 31 , 32 . Because of the high importance of this questionnaire in mindfulness research, we explored the possibility of shorter versions of the MAAS, based on only 5 and 3 items, which would be quicker to implement in future research.
Despite the close conceptual relationship between MW and mindfulness, estimates of the strength of their association have been surprisingly low 3 , 4 , 5 . Furthermore, none of these previous studies reported an estimate of reliability for ES of MW, making the interpretation of this association difficult. See Table 1 for a detailed summary of previous findings. Together, there is only weak evidence to suggest that a direct measure of MW such as ES during the SART correlates with MAAS scores. Moreover, when these associations were reported, they were moderate at best.
Additional evidence for the relationship comes from a related line of research that investigates whether mindfulness training impacts direct and indirect measures of MW (for a review, see 33 ). Such intervention studies found that the practice of mindfulness usually improved SART performance 3 , 34 , 76 , 36 , 37 , 38 and reduced the frequency of self-reported MW in some cases 36 , 39 but not in others 34 , 38 . Moreover, one study reported higher MAAS scores after mindfulness training 35 . Similarly to the findings of those correlation studies reported before, in these mindfulness training studies the associations between the direct MW measure and mindfulness is not as strong as one might expect.
One possible explanation of low associations between ES of MW and MAAS scores could be that queryi ng participants for whether they were on or off task alone conflates over two forms of MW that are opposingly linked to mindfulness, namely MW with and without meta-awareness 6 . This hypothesis has been put forward by Smallwood and Schooler 7 , and initial empirical evidence for the importance of considering meta-awareness was gathered by the same authors in an ensuing study 9 . Here, ‘zone outs’ (MW without awareness) were linked to higher inhibition errors in an ongoing task while ‘tune outs’ (MW with awareness) were not. How these ‘zone out’ and ‘tune out’ propensities are linked to trait mindfulness, however, seems unclear in the previous literature. Deng et al. 4 found no significant relationship between either the ‘zone out’ or the ’tune out’ rate with trait mindfulness as measured by the MAAS. A more recent study 5 found both rates to be negatively associated with MAAS scores. Together there is inconsistent evidence on the role of meta-awareness as potential mediator between MW and trait mindfulness. Another possible explanation for low correlations between SART, ES, and MAAS is insufficient reliability of measures derived from these instruments. Reliability is an often overlooked quality metric in cognitive tasks while it is routinely reported for questionnaires 40 . Reliability estimates are important as they determine an upper limit of how large correlations between two measures can be. For all the individual measures for mindfulness and MW discussed above, robust psychometric properties have been reported before, though rarely combined and sometimes in small samples: MAAS 31 , 32 , specific SART measures with and without ES for MW 41 , 35 , 36 , 37 , 45 . Table 1 lists all referenced studies that measured the MAAS and/or the SART with or without ES of MW. The table depicts sample sizes, reliability estimates and estimates of association. Most importantly, this table shows that none of the previous studies employed all three measures (MAAS, SART, and ES of MW) and reported both, reliabilities of all measures as well as correlations between all of them. The present study aims to fill this gap and offers data from two new large samples.
Overall the aim of this study is to assess the psychometric quality of several measures for MW and mindfulness from the SART, MAAS, ES of MW and ES of meta-awareness. In a second step, we want to gain an estimate for the statistical association between these constructs. We combined ES of MW during the SART with an established measure of mindfulness in an online study in two large samples collected in an online experiment and by doing so add psychometric estimates for these measures gained in an online study and assessed together.
We recruited two samples of participants for a German and an English version of the experiment. In our first recruitment phase we targeted German-speaking participants through the participant pool of our institution, made up of students and volunteers from the public. Throughout the study, we refer to this sample as German-speaking unpaid participants (GUP). In a second phase, we recruited and paid participants predominantly through Amazon Mechanical Turk (AMT, mturk.com) for an English version of the experiment. We refer to this sample as English-speaking paid participants (EPP). All participants first answered a questionnaire on demographics and the MAAS, then they performed a 20 min version of the SART during which ES probes of MW and meta-awareness wee obtained (see “ Methods ” section).
Sample differences
We initially planned to report our findings as one sample, since the online experiment was identical except for the language. However, after finding significant differences between our two samples in the SART and ES data, we decided post-hoc to report all findings separately for GUP and EPP (see Table 2 for sample differences between all main measures). Most strikingly, EPP reported significantly less than half as often to be ‘off task’ than the GUP \((\hbox {t}(519.01) = -10.06\) , p < .001, d \(=\) 0.81, \(M_E{}_P{}_P = 0.09\) , \(M_G{}_U{}_P = 0.25\) ). This indicates much lower variance in ES data in the EPP. There were also significant differences on all measures derived from the SART directly (RT, accuracy) albeit in a lower magnitude (see Table 2 ).
Factorial structure and reliability of the MAAS
We first checked the correlation matrices of the individual items on the questionnaire and the total score, separately for each of the two samples. In the GUP sample, item 6 had low item-to-total correlation (r \(=\) 0.05) and correlations below r \(=\) 0.2 with most other items. For that reason, we excluded item 6 from further analyses for the GUP. Thus, our total MAAS score for the EPP contained all 15 initial items, while the score of the GUP contained only 14 items.
We then conducted an exploratory factor analysis (EFA) for the MAAS responses for each of the two samples (factor loadings for one-factor EFAs are presented in Table 3 ). Figure 1 depicts scree plots for the EPP and GUP; these plots suggest that a single latent factor drives responses in the MAAS. Further EFAs also revealed that two-factor models only explain little additional variance (EPP: 3% and GUP: 5%), in comparison to that explained by one-factor models (EPP: 63% and GUP: 36%). However, the Kaiser rule (selecting the factors with an eigenvalue above 1; indicated by the dotted line in the scree plots) is also in accordance with a two-factor solution in our GUP.
The model fit statistics from confirmatory factor analyses (CFA) were estimated using the Comparative Fit Index (CFI), the Tucker Lewis Index (TLI), and the Root mean square error approximation (RMSEA). We compared the values against common standards for an acceptable fit (CFI/TLI > 0.9, RMSEA < 0.06) 52 . For one-factor models, the fits are acceptably high in the EPP (CFI \(=\) 0.954, TLI \(=\) 0.946). The fits were poorer, however, for the GUP (CFI \(=\) 0.858, TLI \(=\) 0.832). The RMSEA, which is an absolute fit statistic, indicates a poor approximate fit for both models, in the EPP (RMSEA \(=\) 0.08) and GUP (RMSEA \(=\) 0.096). However, the use of a fixed threshold for the RMSEA is questionable 53 , 54 . The full fit statistics of these two models and of an alternative two-factor model for the GUP can be found in the supplementary materials at https://osf.io/8kg6z . Together, EFA and CFA are mostly consistent with the notion of one single factor driving responses to the MAAS, even though some fit statistics for the CFA were below the threshold for an acceptable fit.
Reliabilities of the MAAS score (mean of individual items) were overall high. For the full MAAS the standardized Cronbach’s \(\alpha\) was 0.88 in the GUP sample and 0.96 in the EPP. We created and then investigated shorter versions of the questionnaire consisting of the three or five items with the highest loadings in the EFAs. In the EPP these items were 7, 8, 10, 1, 11, and in the GUP items 14, 8, 9, 10, 7, in order of decreasing loading (see also Table 3 ). We refer to these shortened scales as the MAAS-5 and MAAS-3. The Cronbach’s \(\alpha\) s of the scales are given in Table 4 and further descriptives of the scores are available in the supplementary materials (Table S7 ). Correlations between short and full MAAS scores were reasonably high (between r = 0.79 and r = 0.97, see full correlation matrices in the supplementary materials (Figs. S9 and S10 ).
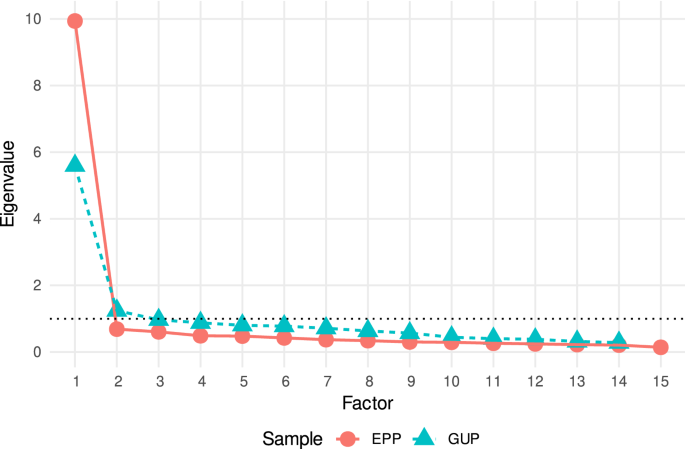
Scree plot for MAAS for EPP and GUP samples. This figure was created using R (v. 4.02) 55 with package ‘ggplot2’ (v. 3.3.5) 56 .
Reliability of MW measures taken from the SART and ES
Estimates of reliability of the measures derived from the SART and ES probes are presented in Table 4 . They are split-half reliabilities derived using a permutation-based approach with 5000 random splits 40 , 57 . For further descriptives of the measures, see Table S7 in the supplementary materials. From the SART, we report these measures: accuracy, the mean (M) and standard deviation (SD) of RTs during all trials and also in only those trials preceding the ES probes within a 10-s time window, a measure used in MW neuroimaging studies 27 . SART values are reported separately for correct Go trials and incorrect Nogo trials. From ES probes, we report the proportion of all ES probes in which participants answered that they were off-task (Attention Off) and the proportion of meta-awareness probes in which participants answered that they were unaware that their attention was off task (Meta-Awareness Off). The sample sizes for the meta-awareness probes were smaller, because they exclude participants who reported that they were always on task. Split-half reliabilities for measures from Go trials in the SART and for ES probes are generally high. Reliabilities for Nogo trials were markedly lower, and were further reduced when restricting the analyses to the 10-s time windows immediately preceding ES probes. It is noteworthy that the sample sizes varied for these different measures due to the structure of the data and restrictions for the split-half calculations: Each participant needed at least four valid data points for the split-half procedure, as each split required two data points to calculate a mean or standard deviation. Furthermore, only 10.6% of all trials were Nogo trials and participants only reacted to 15.2% of Nogo trials, making Nogo trials with participant reaction somewhat scarce.
Estimates of association between the MAAS, SART, and ES
In a next step, we assessed the hypothesized negative association of MW with mindfulness. To this aim, we correlated measures derived from the SART and ES with the MAAS (Fig. 2 ). For the link between the direct measure of MW and mindfulness, we found ES probes (Attention Off) were moderately negatively associated with the MAAS in GUP ( \(\hbox {r} = -.29\) , \(p< 0.001\) ) but not in EPP (r \(=\) 0.04, \(p > 0.1\) ). Between indirect measures of MW and mindfulness, there was no indication for an association between the SART and the MAAS in GUP. In EPP, however, there were small correlations between MAAS total score and SD of RTs in the Go trials during the 10 s window before ES probes ( \(\hbox {r} = -.23\) , \(p < 0.05\) ), between MAAS total score and accuracy in all Nogo trials ( \(\hbox {r} = .13\) , \(p < 0.05\) ), and a medium association between MAAS total score and accuracy of Nogo trials in the 10 s window before ES probes ( \(\hbox {r} = -.43\) , \(p < 0.01\) ). The pattern is mostly consistent with the idea of a negative association of MW and mindfulness. There was no association between meta-awareness probes and MAAS scores in both samples. All pairwise correlations for both samples are available in Tables S1 and S2 in the supplementary materials at https://osf.io/8kg6z .
To check whether these correlations might have been heavily influenced by outliers or non-normally distributed data, we additionally bootstrapped the correlation coefficients and 95% confidence intervals (CIs) for these pairwise correlations (1000 iterations, 100 random participants sampled in each). In addition, we compared the Pearson product-moment correlations to Spearman rank correlations. These analyses showed a similar pattern of results from the Pearson correlations reported above in the GUP, but in the EPP the three reported associations with ES probes were not significant in the Spearman correlations. This further indicates the different answer patterns in self-reported MW between our two samples. The detailed results of these additional versions of the correlations are available in Tables S3 – S6 in the supplementary materials.
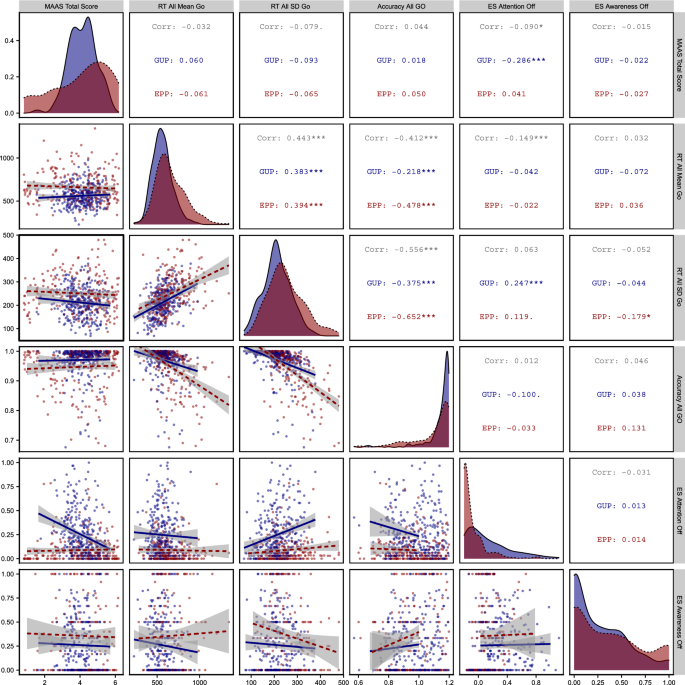
Pairwise Pearson correlations for MAAS, SART, and ES measures. Correlation coefficients are reported for whole sample (‘Corr’), and for EPP and GUP samples separately. Individual plots below the diagonal are scatter plots with regression lines for the two variables intersecting at this cell, those on the diagonal show density distribution plots for the two samples. Significance markers: . \(=\) \(p< 0.1\) , * \(p< 0.05\) , ** \(p< 0.01\) , *** \(p< 0.001\) . This figure was created using R (v. 4.02) 55 with packages ‘ggplot2’ (v. 3.3.5) 56 and ‘GGally’ (v. 2.1.2) 58 .
This study entailed between-subject manipulations hypothesized to affect MW that are out of the scope of the current work. Briefly, we investigated whether exposing participants to auditory stimuli (5 Hz monaural or binaural auditory beats, silence, 440 Hz sine tone) could reduce their propensity to MW. Since such a finding has been reported earlier, in particular for participants exhibiting high proportions of MW 24 , we experimentally manipulated the occurrence of MW in three different ways. First, we varied the inter-stimulus-interval (1 vs. 2 s). Second, we implemented the stimuli in the SART predictably or unpredictably. Third, a creative problem-solving task was executed for a second time after the SART, and participants were either informed before the SART about the second execution or they were not informed.
These between-subject manipulations may have affected our estimates of associations between MW and mindfulness. To investigate this possibility, we first calculated ANOVAs with the experiment’s main manipulations (and all pairwise interactions) as predictors and measures from SART and ES as outcome variables. We then added the MAAS score as covariate to these, to create a set of comparable ANCOVAs. To evaluate whether our associations were affected by the experimental manipulations, we then checked two things. First, we compared the effect sizes ( \(\eta ^2\) ) of the total MAAS score in these ANCOVAs with the coefficient of determination ( \(r^2\) ) between the MAAS score and SART and ES measures. Second, we calculated model comparisons between the ANOVAs and ANCOVAs using likelihood-ratio tests (Table 5 ).
The effect sizes were for most combinations very similar in the correlations and the ANCOVAs. In all but one case, adding the MAAS score as covariate did not significantly improve the model fit. Only in the ES MW variable in GUP did adding the MAAS score as covariate significantly improve the model fit. There the estimate of association between ES MW and the MAAS score slightly increased when accounting for experimental manipulations. This result provides confirmatory evidence that MAAS and ES MW are weakly negatively associated in the GUP sample.
We examined the psychometrics of MW, meta-awareness of MW, and trait mindfulness, as well as the associations between these constructs. Overall, we found reasonably good psychometrics of all measures, and evidence that MW and trait mindfulness are indeed moderately negatively correlated. This association was not moderated by meta-awareness of MW. Neither the psychometrics nor moderating effects of meta-awareness can therefore readily explain that associations between MW and mindfulness are of a rather low magnitude.
In keeping with previous studies, we found overall good psychometric properties and evidence mostly consistent with a single-factor structure for the MAAS questionnaire. Our estimates of reliability of the MAAS were slightly higher than those reported in earlier studies, in both the EPP and GUP. For the English MAAS, the original publication reported internal consistencies in the range of [0.8, 0.87] 31 , and a further study reported 0.89 48 , but this value was 0.96 in our EPP. For the German MAAS, a Cronbach’s \(\alpha\) of 0.83 was reported in the initial publication on the psychometric properties of the questionnaire 49 , while the value in our GUP was 0.88. Very high internal consistencies might indicate redundancy in a questionnaire, suggesting some items are superfluous and can be removed, which would lead to a more efficient assessment 59 . Results on the proposed shorter versions of the MAAS (MAAS-5 and MAAS-3) outlined in this study support this notion and may provide researchers with tools to optimize data collection.
One peculiarity we observed in the MAAS data for the GUP was item 6 ( “I forget a person’s name almost as soon as I’ve been told it for the first time.” 31 ), which correlated very poorly with all other items and the total score. Interestingly, the authors of the German MAAS also observed complications with this item but decided to include it to ensure international comparability 49 . Specifically, they found an item-to-total correlation of r \(=\) 0.18 for item 6 while the next-lowest correlation was for item 1 (r \(=\) 0.26) and those for all other items ranged from 0.33 to 0.65 We did not observe, however, such a low item-to-total correlation of item 6 in EPP. Nevertheless, we assume that cultural differences or mere issues related to translation cannot account for low item-to-total correlation for this item, as it was also observed in a study with English-speaking participants from New Zealand 50 . Moreover, item 6 was also one of the most poorly correlated items in the original English article detailing the MAAS 31 . We suggest item 6 may only occasionally be problematic as its meaning is ambiguous, and can be understood in two different ways. First, it could—probably as intended by the authors of the scale—measure attention usually directed to a person introducing themselves, or it can be understood as asking for self-report on one’s long-term memory abilities, which is arguably an altogether different trait than mindfulness.
While reliability is routinely reported for questionnaires such as the MAAS, they are less common for cognitive behavioral measures, e.g. for the MW measures derived from the SART and ES 40 . Still, earlier studies generally reported high reliabilities also for the SART: e.g. between 0.83 and 0.89 for overall accuracy in the SART 42 , 44 , between 0.92 and 0.98 for SDs of RT 44 , 45 , and even as high as 0.94 to 0.98 for the accuracy of Nogo trials 41 (see Table 1 ). Some of these studies, however, used a shorter stimulus-onset asynchrony (SOA) and much smaller sample sizes (13 42 and 12 41 participants). Also, earlier studies reporting SART reliabilities were usually laboratory studies with more controlled environments. These factors might have led to even higher reliabilty estimates for measures derived from Nogo trials. Our study adds further reasonably high reliabilities with alphas ranging from 0.84 to 0.99, on measures derived from the Go trials of the SART. In contrast to previous studies, reliability estimates for measures derived from Nogo trials were markedly lower (between 0.24 and 0.71) in our samples. These were probably low in our study due to only a small fraction of the SART trials that can be used to derive these measures as we increased the SOA from the original version in order to foster MW. Overall reliabilities are further reduced when restricting the analyses to a short time window preceding ES probes. Filtering the usable trials to a specific time window seems predominately appropriate for neuroimaging studies looking to isolate brain activity patterns of MW, which is where this analysis strategy originated 27 . Researchers focusing on Nogo trials and segmenting the data accordingly, should therefore take care to ensure that the number of trials analyzed remains reasonably large, and bear in mind that reliability of measures derived with these strategies is likely limited. Our reliability estimates for the ES MW probes during the SART (0.91 in GUP and 0.89 in EPP) were within the range of what earlier studies reported (e.g., 0.89 43 and 0.93 45 ). Together with the reliability estimates of the MAAS, our study demonstrates that high reliabilities of the MAAS, SART, and ES for MW can also be obtained in an online study setting.
Our results also stress notions of caution related to recruiting participants via crowdsourcing platforms such as—as in our case—Amazon Mechanical Turk (AMT, mturk.com). We noticed that the two samples behaved differently in the SART and ES, in that AMT participants (the EPP) were less likely to respond that their attention had been ‘off task’ but at the same time showed lower accuracy rates and slower, more varied RTs during the SART. This is likely to have also affected the estimate of association between self-reports of MW in ES probes and the MAAS score. A significant correlation was found in GUP, but not in EPP. The absence of a significant correlation could be due to lower variance in the ES probes of EPP versus GUP. We suggest the different patterns of results relating to the ES probes is not simply due to cultural or language differences, but rather due to differences in motivation to participate. Requesters at AMT are allowed to withhold payment if they are not satisfied with the performance of the participant. It thus seems reasonable to assume that some participants recruited through AMT reported being on task even when they were not. Our data underlies arguments made earlier that caution is warranted when recruiting via AMT and similar platforms, especially when using measures that are susceptible to the issues discussed above 60 , 61 . It might help to explicitly ensure participants that they will experience no disadvantages when they report being off task.
Our results support the hypothesis of a negative link between trait mindfulness and MW. Associations, however, were scattered over different measures and differed between our two samples: There was a moderate correlation of the MAAS with the self-report measure of MW (ES probes during the SART) in one of our samples (GUP) and with SART SDs of RTs and SART accuracy in the SART in the other sample (EPP). Low and absent associations between MW and mindfulness cannot be explained by low reliabilities of the measures we used, as reliabilities were generally satisfyingly high, with the exception of measures derived from SART Nogo trials. With that in mind, the associations based on measures with high realiabilities are only two: that between MAAS total score and ES MW in the GUP, and between MAAS total score and SDs of RTs in SART Go trials during the 10 s window before ES probes in the EPP. One potential explanation for finding the clear association between MAAS and ES MW only in the GUP might be a lack of variance in the EPP data as mentioned above. The lack of variance was due to a large proportion of participants who answered that they were rarely or never ‘off task’ during the experiment.
Despite good psychometrics of our measures, the link between trait mindfulness and MW was only moderate. A further explanation for rather low associations could be that meta-awareness of MW moderates the hypothesized associations. Our finding that meta-awareness of MW is not linked to mindfulness goes against such a hypothesis and some empirical evidence 7 , 23 . However, our results are in accordance with more recent papers that also do not find a moderating effect of meta-awareness on the association between MW and mindfulness 4 , 5 . Nayda et al. 5 reported negative associations between both, the propensity to ‘tune out’ (meta-aware MW) with mindfulness, and the propensity to ‘zone out’ (meta-unaware MW). An earlier publication by Deng et al. 4 found insignificant correlations between trait MW and both ‘zone out’ and ‘tune out’ propensities. It seems noteworthy that both correlations of the Deng et al. 4 study are in the same range and direction as in Nayda et al. 5 but do not reach statistical significance likely due to the low sample size (N \(=\) 23). A potential caveat here is that these rates are calculated using the total of MW probes, rather than the total of meta-awareness probes only. These estimates are therefore biased in that the sum of the ‘tune out’ and ‘zone out’ rates is perfectly inverse proportional to the ‘on-task’ rate. In our analyses, we calculated the meta-awareness rate as proportion of the total of meta-awareness probes instead of the total of MW probes. We found no significant correlation between meta-awareness of MW and mindfulness. Thus, further research seems needed to isolate a potentially moderating effect of meta-awareness on the correlation between MW and mindfulness.
A further reason for low associations between MW and mindfulness could result from the difference in the trait versus transient nature of the constructs. Mindfulness is conceived and measured as a general personality trait. However, MW is a much more transient and fluctuating phenomenon during an ongoing and often boring task. Moreover, boredom itself may explain the low associations between MW and mindfulness. In MW research, the SART is often chosen as an ongoing task, because it is boring and therefore is thought to facilitate MW. The notion that boredom is an enabling factor for MW is supported by two findings. First, boredom has been shown to correlate with attentional lapses as measured with the SART 62 . Second, positive correlations between boredom and MW have been recently reported 63 . In contrast, when participants respond to the mindfulness questions of the MAAS, it is unclear to what extent participants consider boring ongoing tasks (e.g., “I rush through activities without being really attentive to them.” see Table 3 for the complete list of items of the MAAS). Therefore, while boredom seems a relevant aspect of MW when measured with the SART, this is not assessed with the MAAS. Together, this emphasizes the necessity of investigating the role of boredom in the relation between MW and mindfulness in future studies.
One may argue that a further reason for low associations between MW and trait mindfulness could be that the on-task state is more heterogeneous than previously thought. Heterogeneous on-task states were identified by assessing ongoing thought with multidimensional experience sampling (MDES), i.e., extending ES with several questions inquiring about the thoughts’ content and nature 64 . Principal component analysis (PCA) of MDES data revealed several components taxing into the on-task state, which were associated with distinct neural correlates 65 , 59 , 60 , 68 . One component was related to self-focused off-task thoughts while another component indicated detailed task focus. This task-focused component was common in cognitively demanding tasks like tasks measuring working memory, task switching, and gambling. However, it was less observed in low-demand tasks like the SART, where self-focused off-task thoughts prevail 64 . Together, these studies suggest that being more mindful might be linked to how people engage with tasks, perhaps by doing so in a more focused way. The possibility of multiple on-task states may therefore, contribute to the relatively low estimate of the association between mindfulness and ongoing thought.
Finally, low associations between MW and mindfulness could be due to insufficient validity, rather than reliability of the measures we used. While our current study focuses on reliability others have focused on issues related to validity, especially concerning the questionnaires to measure mindfulness 69 . On the one hand, the MAAS in particular has been shown to correlate reasonably well with other questionnaires measuring mindfulness such as the Five Facet Mindfulness Questionnaire (FFMQ) 70 . Further evidence for converging validity with, e.g., positive affect or attention, as well as evidence for discriminant validity, e.g., with anxiety and rumination, has been found in studies reporting correlations with MAAS scores 31 , 32 . On the other hand, questionnaires rely on introspective capabilities and may be subject to bias. A recent study by Isbel et al. 70 questioned especially the discriminant validity of the MAAS and the FFMQ as these measures increased following both a mindfulness training intervention and a control training intervention not aimed at mindfulness. Rather, objective accuracy of breath counting has been found to respond selectively to the mindfulness training intervention 70 . A potential reason why the breath counting task responded selectively to the mindfulness training is that mindfulness training itself often consists of exercises to guide one’s attention specifically on the breath. It is hence a rather near transfer from mindfulness training to an increase in accuracy in breath counting. Nevertheless, more research exploring the practical validity of mindfulness measures is required.
Recent methodological developments in MW research highlight limitations in our findings and offer advice for future research. Contemporary studies of ongoing thought that utilized MDES show that different tasks used in MW research elicit several distinct thought patterns to varying degrees 64 , 67 . Our study is consequently limited by the fact that we only used the SART to investigate the association between individual variation in mindfulness and MW instead of several tasks. The SART also has the limitation that it does not lead to much detailed task focus and tends to stimulate self-focused MW 64 . Due to that, it is unclear whether our findings generalize to other tasks or whether they are specific to the SART and thus to those types of ongoing thoughts more likely to be evoked by the SART like self-focused MW.
Besides the heterogeneity of ongoing thoughts, the relationship between MW and mindfulness is likely modulated by various other factors. A recent study has highlighted MW as a complex phenomenon that warrants a multi-faceted approach that includes a) dispositional traits, like conscientiousness, agreeableness, or mindfulness, b) contextual factors, like motivation or alertness, and c) cognitive abilities, like working memory capacity 71 . If the relationship between MW and mindfulness is embedded within such a multi-faceted approach, the association between these two factors might be diluted by other potential confounding factors that were not accounted for. In this regard, future research will benefit from assessing MW and mindfulness with a broad set of tools including MDES and multiple tasks with variable demands that elicit different patterns of ongoing thoughts.
Participants
A total of 715 participants performed or started our online experiment between October 2019 and January 2021. We excluded participants from the data analysis for these reasons and in this order: Repeated participation (n \(=\) 11), incomplete data due to technical issues (n \(=\) 1), incomplete or delayed participation in the experiment (time in experiment < 23 min or > 120 min [n \(=\) 59]), low number of correct SART trials (proportion of correct Go trials < 2/3 [n \(=\) 51], or proportion of correct Nogo trials < 1/2 [n \(=\) 22]), and outliers who took a long time to answer the ES probes (n \(=\) 30). For this last point we established a cutoff based on the interquartile range (IQR) due to the highly skewed distribution of these values. Cutoff was the 75th percentile plus three times the IQR. We based our data analyses on a total sample of 541, separated into 313 GUP (aged between 16 and 85, M \(=\) 38.78, SD \(=\) 12.95) and 228 EPP (aged between 19 and 68, M \(=\) 34.27, SD \(=\) 11.39). Further demographic characteristics are given in Table 6 .
We recruited participants for two different language versions of the experiment through various routes. The GUP (n \(=\) 313) consists of: (a) 97 participants recruited by the students of two classes in the autumn 2019 and spring 2020 semesters at UniDistance Suisse; (b) 200 students and members of the public interested in participating in experimental research from our institute’s pool of research participants; and (c) 16 participants who followed links in an information email to university employees and on different websites. The EPP (n − 228) contains: (a) 217 participants recruited through AMT, (b) 10 who were PhD students at the Department of Epileptology at the University of Bonn, and (c) 1 who followed a link from an external website.
Those recruited through AMT were paid USD 3.50 when they had completed the whole experiment. Students in the Bachelor’s program in Psychology at the UniDistance Suisse received course credits for their participation. Other participants received no compensation. All participants gave informed consent by reading information provided online and then checking off tick boxes in an online form before they proceeded to the experiment. The study was carried out following all the relevant guidelines and regulations. The study and its compliance with relevant guidelines and regulations was approved by the ethical review committee of the Faculty of Psychology at UniDistance Suisse ( https://distanceuniversity.ch/research/ethics-commission/ ). In particular, all procedures are in accordance with the Declaration of Helsinki.
The data reported here was collected in a study also investigating the effects of experimental manipulations on MW. Participants performed the SART with intermittent ES probes to directly obtain self-reports of episodes of MW. These experimental manipulations are outside the scope of the current work as they focus on potential effects of auditory beat stimulation on MW 24 , 72 and will be reported elsewhere. Briefly, experimental manipulations were performed in a \(4\times 2\times 2\times 2\) between-subjects design and included the independent variables Auditory Beat Stimulation (5 Hz binaural, 5 Hz monaural; 440 Hz pure tone; no sound), SART ISI (1 or 2 s), Predictability of the Number Sequence in the SART (random or ascending), and Expectancy of an ensuing creativity task (expected or unexpected). Dependent variables are RTs and Accuracy during the SART and ES MW probes. It was for the purpose of this study, that we collected data using the MAAS.
Instruments
To assess trait mindfulness we applied the MAAS, a 15-item questionnaire that determines attention to the present in everyday experiences 31 . For the German version of the experiment, we used the validated German translation available from the Leibniz Institute for Psychology Information (ZPID) 73 .
To measure MW indirectly through lapses in sustained attention in a deliberately monotonous task, we used the SART 21 . The SART is a Go/No-go task that uses digits as stimuli which are presented individually on screen with a fixation cross shown between stimuli. Participants are asked to react to all digits (Go trials) except for the number 7 (Nogo trials). We adapted the original SART with the intention to make it more monotonous, in order to elicit more MW. Specifically, we displayed each stimulus longer (2 s instead of 250 ms), had a longer ISI (1 or 2 s instead of 900 ms), and used a fixed font size (instead of randomly varying font sizes) to present our stimuli 21 .
We assessed self-reported MW using ES probes during the SART. In intervals between 25 and 35 s, participants were asked: “Immediately before this question appeared, was your attention focused ON the task or OFF task?” with a dichotomous forced-choice answer. When “OFF task” was selected, a second question appeared: “Were you aware that your attention was OFF task?” with a dichotomous forced choice answer again (yes or no). There was no time limit to answer these probes.
To further increase MW by adding a cognitive distraction during the SART and to assess particpants’ creativity, we implemented a short task for divergent thinking based on the alternative/unusual uses concept originally introduced by Guilford 74 . In this unusual uses task (UUT), participants were given 20 s to find alternative uses for a brick, with the original use described as “building houses”. Participants entered their answer in a large text field and were asked to enter one answer per line.
We implemented the MAAS and SART with ES as an online experiment using the JavaScript-based online experiment builder “lab.js” ( https://lab.js.org 75 . Participants were required to wear headphones during the experiment. We included a headphone test before the SART to ensure participants had correctly placed the headphones and could listen to the stimulation. Runnable files and code for both language versions of the experiment can be found in the supplementary materials at https://osf.io/8kg6z .
The online experiment started with information about the experiment, data processing, and informed consent request. This was followed by a short demographic questionnaire, the MAAS, the headphone test, the UUT, and 20 min of the SART. After the SART, a summary page informed the participants about their performance and a debriefing page gave further background information about the study.
Data processing, analysis and creation of figures and tables were done in R (v 4.0.2) 55 , using the following packages in addition to base R: ‘tidyverse’ 76 for various data wrangling and processing tasks and for data visualizations via ‘ggplot2’ 56 , ‘GGally’ 58 for data visualizations, ‘e1071’ 77 for kurtosis and skewness calculations, ‘lubridate’ 78 for handling of date and time data, ‘lavaan’ 79 for confirmatory factor analyses, ‘stargazer’ 80 to create and export LaTeX tables, ‘splithalf’ 57 for permutation-based split-half calculatio ns.
Data availability
The datasets generated and analysed for the current study are available in the Open Science Framework (OSF) repository, https://osf.io/wg9q5 . Tables, figures, and other supplementary materials specifically for this publication are available in a different repository at OSF, https://osf.io/8kg6z .
Smallwood, J. & Schooler, J. W. The science of mind wandering: Empirically navigating the stream of consciousness. Annu. Rev. Psychol. 66 , 487–518. https://doi.org/10.1146/annurev-psych-010814-015331 (2015).
Article PubMed Google Scholar
Kabat-Zinn, J. Full Catastrophe Living: Using the Wisdom of Your Body and Mind to Face Stress, Pain, and Illness (Batam Books Trade Paperbacks, 2013).
Mrazek, M. D., Smallwood, J. & Schooler, J. W. Mindfulness and mind-wandering: Finding convergence through opposing constructs. Emotion 12 , 442–448. https://doi.org/10.1037/a0026678 (2012).
Deng, Y.-Q., Li, S. & Tang, Y.-Y. The relationship between wandering mind, depression and mindfulness. Mindfulness 5 , 124–128. https://doi.org/10.1007/s12671-012-0157-7 (2014).
Article Google Scholar
Nayda, D. M. & Takarangi, M. K. The cost of being absent: Is meta-awareness of mind-wandering related to depression symptom severity, rumination tendencies and trauma intrusions? J. Affect. Disord. 292 , 131–138. https://doi.org/10.1016/j.jad.2021.05.053 (2021).
Dunne, J. D., Thompson, E. & Schooler, J. Mindful meta-awareness: Sustained and non-propositional. Curr. Opin. Psychol. 28 , 307–311. https://doi.org/10.1016/j.copsyc.2019.07.003 (2019).
Smallwood, J. & Schooler, J. W. The restless mind. Psychol. Bull. 132 , 946–958. https://doi.org/10.1037/0033-2909.132.6.946 (2006).
Killingsworth, M. A. & Gilbert, D. T. A wandering mind is an unhappy mind. Science 330 , 932–932. https://doi.org/10.1126/science.1192439 (2010).
Article ADS CAS PubMed Google Scholar
Smallwood, J., O’Connor, R. C., Sudbery, M. V. & Obonsawin, M. Mind-wandering and dysphoria. Cogn. Emot. 21 , 816–842. https://doi.org/10.1080/02699930600911531 (2007).
Malhi, G. S. et al. Mood disorders: Neurocognitive models. Bipolar Disord. 17 , 3–20. https://doi.org/10.1111/bdi.12353 (2015).
Article ADS MathSciNet PubMed Google Scholar
Fell, J. Is the hippocampus a potential target for the modulation of mind wandering in major depression? Front. Psychiatry 9 , 10. https://doi.org/10.3389/fpsyt.2018.00363 (2018).
Yanko, M. R. & Spalek, T. M. Driving with the wandering mind: The effect that mind-wandering has on driving performance. Hum. Factors 56 , 260–269. https://doi.org/10.1177/0018720813495280 (2014).
Di Nocera, F. et al. Attentional control in accidents involving agricultural tractor operators. Ergon. Des. Q. Hum. Factors Appl. 26 , 17–23. https://doi.org/10.1177/1064804617737444 (2018).
Smallwood, J., Mrazek, M. D. & Schooler, J. W. Medicine for the wandering mind: mind wandering in medical practice. Med. Educ. 45 , 1072–1080. https://doi.org/10.1111/j.1365-2923.2011.04074.x (2011).
Smallwood, J., Fishman, D. J. & Schooler, J. W. Counting the cost of an absent mind: Mind wandering as an underrecognized influence on educational performance. Psychon. Bull. Rev. 14 , 230–236. https://doi.org/10.3758/BF03194057 (2007).
Leszczynski, M. et al. Mind wandering simultaneously prolongs reactions and promotes creative incubation. Sci. Rep. 7 , 10197. https://doi.org/10.1038/s41598-017-10616-3 (2017).
Article ADS CAS PubMed PubMed Central Google Scholar
Mooneyham, B. W. & Schooler, J. W. The costs and benefits of mind-wandering: A review. Can. J. Exp. Psychol. 67 , 11–18. https://doi.org/10.1037/a0031569 (2013).
Medea, B. et al. How do we decide what to do? Resting-state connectivity patterns and components of self-generated thought linked to the development of more concrete personal goals. Exp. Brain Res. 236 , 2469–2481. https://doi.org/10.1007/s00221-016-4729-y (2018).
Hayes, S. C., Levin, M. E., Plumb-Vilardaga, J., Villatte, J. L. & Pistorello, J. Acceptance and commitment therapy and contextual behavioral science: Examining the progress of a distinctive model of behavioral and cognitive therapy. Behav. Ther. 44 , 180–198. https://doi.org/10.1016/j.beth.2009.08.002 (2013).
Gu, J., Strauss, C., Bond, R. & Cavanagh, K. How do mindfulness-based cognitive therapy and mindfulness-based stress reduction improve mental health and wellbeing? A systematic review and meta-analysis of mediation studies. Clin. Psychol. Rev. 37 , 1–12. https://doi.org/10.1016/j.cpr.2015.01.006 (2015).
Article CAS PubMed Google Scholar
Robertson, I. H., Manly, T., Andrade, J., Baddeley, B. T. & Yiend, J. ‘Oops!’: Performance correlates of everyday attentional failures in traumatic brain injured and normal subjects. Neuropsychologia 35 , 747–758. https://doi.org/10.1016/S0028-3932(97)00015-8 (1997).
Jonker, T. R., Seli, P., Cheyne, J. A. & Smilek, D. Performance reactivity in a continuous-performance task: Implications for understanding post-error behavior. Conscious. Cogn. 22 , 1468–1476. https://doi.org/10.1016/j.concog.2013.10.005 (2013).
Smallwood, J., McSpadden, M. & Schooler, J. W. The lights are on but no one’s home: Meta-awareness and the decoupling of attention when the mind wanders. Psychon. Bull. Rev. 14 , 527–533. https://doi.org/10.3758/BF03194102 (2007).
Chaieb, L., Derner, M., Leszczyński, M. & Fell, J. Modulation of mind wandering using auditory beat stimulation: A pilot study. J. Cogn. Enhanc. 4 , 40–48. https://doi.org/10.1007/s41465-019-00137-4 (2019).
Manly, T., Robertson, I. H., Galloway, M. & Hawkins, K. The absent mind: Further investigations of sustained attention to response. Neuropsychologia 37 , 661–670. https://doi.org/10.1016/S0028-3932(98)00127-4 (1999).
Smallwood, J. et al. Subjective experience and the attentional lapse: Task engagement and disengagement during sustained attention. Conscious. Cogn. 13 , 657–690. https://doi.org/10.1016/j.concog.2004.06.003 (2004).
Christoff, K., Gordon, A. M., Smallwood, J., Smith, R. & Schooler, J. W. Experience sampling during fMRI reveals default network and executive system contributions to mind wandering. In Proceedings of the National Academy of Sciences , Vol. 106 8719–8724, https://doi.org/10.1073/pnas.0900234106 (2009).
Smucny, J., Rojas, D. C., Eichman, L. C. & Tregellas, J. R. Neuronal effects of auditory distraction on visual attention. Brain Cogn. 81 , 263–270. https://doi.org/10.1016/j.bandc.2012.11.008 (2013).
Article PubMed PubMed Central Google Scholar
Seli, P., Risko, E. F. & Smilek, D. On the necessity of distinguishing between unintentional and intentional mind wandering. Psychol. Sci. 27 , 685–691. https://doi.org/10.1177/0956797616634068 (2016).
Birnie, L. H. W., Smallwood, J., Reay, J. & Riby, L. M. Glucose and the wandering mind: Not paying attention or simply out of fuel? Psychopharmacology 232 , 2903–2910. https://doi.org/10.1007/s00213-015-3926-x (2015).
Brown, K. W. & Ryan, R. M. The benefits of being present: Mindfulness and its role in psychological well-being. J. Pers. Soc. Psychol. 84 , 27. https://doi.org/10.1037/0022-3514.84.4.822 (2003).
Park, T., Reilly-Spong, M. & Gross, C. R. Mindfulness: A systematic review of instruments to measure an emergent patient-reported outcome (PRO). Qual. Life Res. 22 , 2639–2659. https://doi.org/10.1007/s11136-013-0395-8 (2013).
Feruglio, S., Matiz, A., Pagnoni, G., Fabbro, F. & Crescentini, C. The Impact of Mindfulness Meditation on the Wandering Mind: a Systematic Review. Neurosci. Biobehav. Rev. 131 , 313–330. https://doi.org/10.1016/j.neubiorev.2021.09.032 (2021).
Giannandrea, A. et al. Effects of the Mindfulness-Based Stress Reduction Program on Mind Wandering and Dispositional Mindfulness Facets. Mindfulness . 10 (1), 185–195. https://doi.org/10.1007/s12671-018-1070-5 (2019).
Bennike, I. H., Wieghorst, A. & Kirk, U. Online-based Mindfulness Training Reduces Behavioral Markers of Mind Wandering. J. Cogn. Enhanc. 1 (2), 172–181. https://doi.org/10.1007/s41465-017-0020-9 (2017).
Morrison, A. B., Goolsarran, M., Rogers, S. L. & Jha, A. P. Taming a wandering attention: Short-form mindfulness training in student cohorts. Front. Hum. Neurosci. 7 , 897. https://doi.org/10.3389/fnhum.2013.00897 (2014).
Rahl, H. A., Lindsay, E. K., Pacilio, L. E., Brown, K. W. & Creswell, J. D. Brief mindfulness meditation training reduces mind wandering: The critical role of acceptance. Emotion 17 (2), 224–230. https://doi.org/10.1037/emo0000250 (2017).
Cantone, D., Feruglio, S., Crescentini, C., Cinot, S. & Matiz, A. A multilevel approach to explore the wandering mind and its connections with mindfulness and personality. Behav. Sci. 11 (9), 125. https://doi.org/10.3390/bs11090125 (2021).
Mrazek, M. D., Franklin, M. S., Phillips, D. T., Baird, B. & Schooler, J. W. Mindfulness Training Improves Working Memory Capacity and GRE Performance While Reducing Mind Wandering. Psychol. Sci. 24 (5), 776–781. https://doi.org/10.1177/0956797612459659 (2013).
Parsons, S., Kruijt, A.-W. & Fox, E. Psychological science needs a standard practice of reporting the reliability of cognitive-behavioral measurements. Adv. Methods Pract. Psychol. Sci. 2 , 378–395. https://doi.org/10.1177/2515245919879695 (2019).
Sofuoglu, M., Waters, A. J., Mooney, M. & Kosten, T. Riluzole and d-amphetamine interactions in humans. Prog. Neuropsychopharmacol. Biol. Psychiatry 32 , 16–22. https://doi.org/10.1016/j.pnpbp.2007.05.003 (2008).
O’Connell, R. G. et al. Two types of action error: Electrophysiological evidence for separable inhibitory and sustained attention neural mechanisms producing error on go/no-go tasks. J. Cogn. Neurosci. 21 , 93–104. https://doi.org/10.1162/jocn.2009.21008 (2009).
McVay, J. C. & Kane, M. J. Conducting the train of thought: Working memory capacity, goal neglect, and mind wandering in an executive-control task. J. Exp. Psychol. Learn. Mem. Cogn. 35 , 196. https://doi.org/10.1037/a0014104 (2009).
Unsworth, N. & McMillan, B. D. Similarities and differences between mind-wandering and external distraction: A latent variable analysis of lapses of attention and their relation to cognitive abilities. Acta Physiol. (Oxf.) 150 , 14–25. https://doi.org/10.1016/j.actpsy.2014.04.001 (2014).
Google Scholar
Kane, M. J. et al. Individual differences in the executive control of attention, memory, and thought, and their associations with schizotypy. J. Exp. Psychol. Gen. 145 , 1017. https://doi.apa.org/doi/10.1037/xge0000184 (2016).
Smilek, D., Carriere, J. S. A. & Cheyne, J. A. Failures of sustained attention in life, lab, and brain: Ecological validity of the SART. Neuropsychologia 48 , 2564–2570. https://doi.org/10.1016/j.neuropsychologia.2010.05.002 (2010).
Cheyne, J. A., Carriere, J. S. & Smilek, D. Absent-mindedness: Lapses of conscious awareness and everyday cognitive failures. Conscious. Cogn. 15 , 578–592. https://doi.org/10.1016/j.concog.2005.11.009 (2006).
MacKillop, J. & Anderson, E. J. Further psychometric validation of the mindful attention awareness scale (MAAS). J. Psychopathol. Behav. Assess. 29 , 289–293. https://doi.org/10.1007/s10862-007-9045-1 (2007).
Michalak, J., Heidenreich, T., Ströhle, G. & Nachtigall, C. Die deutsche Version der Mindful Attention and Awareness Scale (MAAS) Psychometrische Befunde zu einem Achtsamkeitsfragebogen. Z. Klin. Psychol. Psychother. 37 , 200–208. https://doi.org/10.1026/1616-3443.37.3.200 (2008).
Medvedev, O. N. et al. Measuring trait mindfulness: How to improve the precision of the mindful attention awareness scale using a Rasch model. Mindfulness 7 , 384–395. https://doi.org/10.1007/s12671-015-0454-z (2016).
Lewis-Beck, M., Bryman, A. & Futing Liao, T. The SAGE encyclopedia of social science research. Methods . https://doi.org/10.4135/9781412950589NV-0 (2004).
Hooper, D., Coughlan, J. & Mullen, M. R. Structural equation modelling: Guidelines for determining model fit. Electron. J. Bus. Res. Methods 6 , 53–60. https://doi.org/10.21427/D79B73 (2008)
Kline, R. B. Principles and Practice of Structural Equation Modeling (Guilford publications, 2015).
Chen F, Curran, P. J., Bollen, K. A., Kirby, J. & Paxton, P. An empirical evaluation of the use of fixed cutoff points in RMSEA test statistic in structural equation models. Sociol. Methods Res. 36 , 462–494, https://doi.org/10.1177/0049124108314720 (2008).
Article MathSciNet Google Scholar
R Core Team. R: A Language and Environment for Statistical Computing (R Foundation for Statistical Computing, Vienna, 2020).
Wickham, H. ggplot2: Elegant Graphics for Data Analysis (Springer, New York, 2016).
Book Google Scholar
Parsons, S. splithalf—robust estimates of split half reliability. https://doi.org/10.6084/m9.figshare.5559175.v5 (2020).
Schloerke, B. et al. GGally: Extension to ’ggplot2’ . R package version 2.1.2. https://CRAN.R-project.org/package=GGally (2021).
Tavakol, M. & Dennick, R. Making sense of Cronbach’s alpha. Int. J. Med. Educ. 2 , 53–55. https://doi.org/10.5116/ijme.4dfb.8dfd (2011).
Ward, P., Clark, T., Zabriskie, R. & Morris, T. Paper/pencil versus online data collection: An exploratory study. J. Leis. Res. 46 , 84–105. https://doi.org/10.1080/00222216.2014.11950314 (2014).
Chmielewski, M. & Kucker, S. C. An MTurk crisis? Shifts in data quality and the impact on study results. Social Psychological and Personality Science 11 , 464–473. https://doi.org/10.1177/1948550619875149 (2020).
Malkovsky, E., Merrifield, C., Goldberg, Y. & Danckert, J. Exploring the relationship between boredom and sustained attention. Exp. Brain Res. 221 , 59–67. https://doi.org/10.1007/s00221-012-3147-z (2012).
Martarelli, C. S., Bertrams, A. & Wolff, W. A personality trait-based network of boredom, spontaneous and deliberate mind-wandering. Assessment 28 , 1915–1931. https://doi.org/10.1177/1073191120936336 (2021).
Konu, D. et al. Exploring patterns of ongoing thought under naturalistic and conventional task-based conditions. Conscious. Cogn. 93 , 103139. https://doi.org/10.1016/j.concog.2021.103139 (2021).
Sormaz, M. et al. Default mode network can support the level of detail in experience during active task states. Proc. Natl. Acad. Sci. 115 , E11198–E11198. https://doi.org/10.1073/pnas.1817966115 (2018).
Article CAS Google Scholar
Konu, D. et al. A role for the ventromedial prefrontal cortex in self-generated episodic social cognition. Neuroimage 218 , 116977. https://doi.org/10.1016/j.neuroimage.2020.116977 (2020).
Smallwood, J. et al. The neural correlates of ongoing conscious thought. iScience 24 , 102132. https://doi.org/10.1016/j.isci.2021.102132 (2021).
Article ADS PubMed PubMed Central Google Scholar
Turnbull, A. et al. Left dorsolateral prefrontal cortex supports context-dependent prioritisation of off-task thought. Nat. Commun. 10 , 3816. https://doi.org/10.1038/s41467-019-11764-y (2019).
Grossman, P. On measuring mindfulness in psychosomatic and psychological research. J. Psychosom. Res. 64 , 405–408. https://doi.org/10.1016/j.jpsychores.2008.02.001 (2008).
Isbel, B., Stefanidis, K. & Summers, M. J. Assessing mindfulness: Experimental support for the discriminant validity of breath counting as a measure of mindfulness but not self-report questionnaires. Psychol. Assess. 32 , 1184–1190. https://doi.org/10.1037/pas0000957 (2020).
Robison, M. K., Miller, A. L. & Unsworth, N. A multi-faceted approach to understanding individual differences in mind-wandering. Cognition 198 , 104078. https://doi.org/10.1016/j.cognition.2019.104078 (2020).
Chaieb, L., Wilpert, E. C., Reber, T. P. & Fell, J. Auditory beat stimulation and its effects on cognition and mood states . https://doi.org/10.3389/fpsyt.2015.00070 (2015).
Michalak, J., Heidenreich, T., Ströhle, G. & Nachtigall, C. MAAS: Mindful attention and awareness scale—deutsche version [Verfahrensdokumentation aus PSYNDEX Tests-Nr. 9006040 und Fragebogen]. In Leibniz-Zentrum für Psychologische Information und Dokumentation (ZPID), Elektronisches Testarchiv , https://doi.org/10.23668/psycharchives.393 (Trier: ZPID, 2011).
Runco, M. A. & Acar, S. Divergent thinking as an indicator of creative potential. Creat. Res. J. 24 , 66–75. https://doi.org/10.1080/10400419.2012.652929 (2012).
Henninger, F., Shevchenko, Y., Mertens, U., Kieslich, P. J. & Hilbig, B. E. lab.js—a free, open, online experiment builder. https://doi.org/10.5281/zenodo.3767907 (2020).
Wickham, H. et al. Welcome to the tidyverse. J. Open Source Softw. 4 , 1686. https://doi.org/10.21105/joss.01686 (2019).
Article ADS Google Scholar
Meyer, D., Dimitriadou, E., Hornik, K., Weingessel, A. & Leisch, F. e1071: Misc Functions of the Department of Statistics, Probability Theory Group (Formerly: E1071), TU Wien. R package version 1.7-4. https://CRAN.R-project.org/package=e1071 (2020).
Grolemund, G. & Wickham, H. Dates and times made easy with lubridate. J. Stat. Softw. 40 , 1–25. https://doi.org/10.18637/jss.v040.i03 (2011).
Rosseel, Y. lavaan: An R package for structural equation modeling. J. Stat. Softw. 48 , 1–36. https://doi.org/10.18637/jss.v048.i02 (2012).
Hlavac, M. Stargazer: Well-Formatted Regression and Summary Statistics Tables. R package version 5.2.2. https://CRAN.R-project.org/package=stargazer (2018).
Download references
Acknowledgements
The authors would like to thank all students of the following two classes at the UniDistance Suisse, who recruited participants for the experiment: “Methoden III: Experimentelle Übungen” during the fall semester 2019, “Wissenschaftliches Arbeiten” during the spring semester 2020.
Author information
Authors and affiliations.
Faculty of Psychology, UniDistance Suisse, 3900, Brig, Switzerland
Angelo Belardi, Alodie Rey-Mermet, Nicolas Rothen & Thomas P. Reber
Department of Epileptology, University of Bonn, 53127, Bonn, Germany
Leila Chaieb, Florian Mormann, Juergen Fell & Thomas P. Reber
You can also search for this author in PubMed Google Scholar
Contributions
Using the CRediT contributor roles taxonomy (casrai.org/credit/). A.B.: Conceptualization, Data curation, Formal analysis, Investigation, Methodology, Project administration, Software, Validation, Visualization, Writing—original draft, Writing—review & editing. L.C.: Conceptualization, Resources, Writing—review & editing. A.R-M.: Conceptualization, Writing—review & editing. F.M.: Resources, Writing—review & editing. N.R.: Resources, Writing—review & editing. J.F.: Conceptualization, Writing—review & editing. T.P.R.: Conceptualization, Resources, Formal analysis, Investigation, Methodology, Project administration, Software, Validation, Supervision, Writing—original draft, Writing—review & editing.
Corresponding author
Correspondence to Angelo Belardi .
Ethics declarations
Competing interests.
The authors declare no competing interests.
Additional information
Publisher's note.
Springer Nature remains neutral with regard to jurisdictional claims in published maps and institutional affiliations.
Supplementary Information
Supplementary information., rights and permissions.
Open Access This article is licensed under a Creative Commons Attribution 4.0 International License, which permits use, sharing, adaptation, distribution and reproduction in any medium or format, as long as you give appropriate credit to the original author(s) and the source, provide a link to the Creative Commons licence, and indicate if changes were made. The images or other third party material in this article are included in the article's Creative Commons licence, unless indicated otherwise in a credit line to the material. If material is not included in the article's Creative Commons licence and your intended use is not permitted by statutory regulation or exceeds the permitted use, you will need to obtain permission directly from the copyright holder. To view a copy of this licence, visit http://creativecommons.org/licenses/by/4.0/ .
Reprints and permissions
About this article
Cite this article.
Belardi, A., Chaieb, L., Rey-Mermet, A. et al. On the relationship between mind wandering and mindfulness. Sci Rep 12 , 7755 (2022). https://doi.org/10.1038/s41598-022-11594-x
Download citation
Received : 07 September 2021
Accepted : 26 April 2022
Published : 11 May 2022
DOI : https://doi.org/10.1038/s41598-022-11594-x
Share this article
Anyone you share the following link with will be able to read this content:
Sorry, a shareable link is not currently available for this article.
Provided by the Springer Nature SharedIt content-sharing initiative
This article is cited by
Effects of 5 hz auditory beat stimulation on mind wandering and sustained attention in an online experiment.
- Angelo Belardi
- Leila Chaieb
- Thomas P. Reber
Journal of Cognitive Enhancement (2024)
Time, valence, and imagination: a comparative study of thoughts in restricted and unrestricted mind wandering
- Halleyson Li
- Thomas Hills
Psychological Research (2024)
By submitting a comment you agree to abide by our Terms and Community Guidelines . If you find something abusive or that does not comply with our terms or guidelines please flag it as inappropriate.
Quick links
- Explore articles by subject
- Guide to authors
- Editorial policies
Sign up for the Nature Briefing newsletter — what matters in science, free to your inbox daily.

Daydreaming and Concentration: What the Science Says
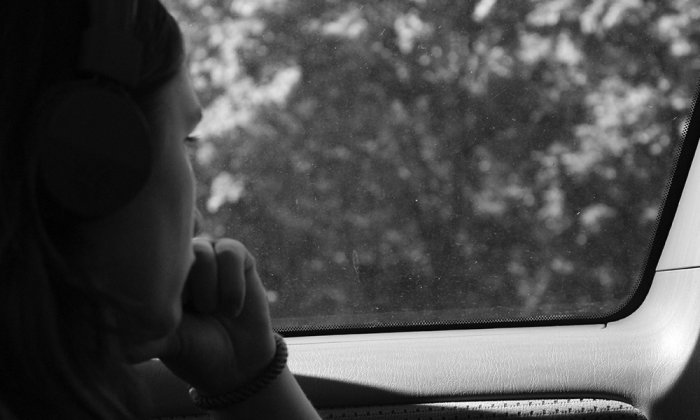
You’re probably familiar with the phenomenon of suddenly catching yourself daydreaming while reading. Your eyes travel back and forth across the text, but the information isn’t being processed. Instead, you’re thinking about the vacation you have planned or the argument you thankfully resolved yesterday. Before you know it, you’ve reached the bottom of the page, but you have no idea what you have just read.
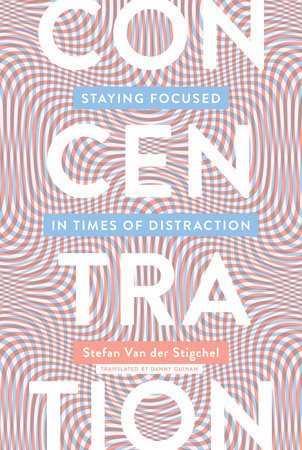
When you are daydreaming (or mind-wandering , as it is more accurately referred to within scientific circles), memories that you thought were lost forever can come to the surface again, or you may suddenly find yourself realizing that you have forgotten someone’s birthday — the kinds of things that don’t happen when you are deep in concentration. The neural activity that can be observed when you are daydreaming is very similar to that found in the “default network,” a network of regions in the brain that are active during periods of rest. This is a brain state in which you are not actively performing any task; in other words, when your working memory is empty.
We actually spend more of our waking hours daydreaming than you might think — as much as half our day, according to at least one study . About a decade ago, scientists at Harvard developed an app that asks test subjects what they are doing at any given moment during the day (a method called experience sampling ) and to report their level of happiness at that particular moment. The scientists are still collecting additional data, but they have already compiled a massive amount of it — approximately 250,000 measurements from more than 2,000 individuals were recorded in that first study alone.
Their findings showed that the participants spent 47 percent of their day daydreaming instead of working on the task they were supposed to be carrying out. When the test subjects indicated that they were thinking of something other than their intended task, they were asked whether they were thinking happy, neutral, or unpleasant thoughts. When the study was published in Science magazine, the headline, surprisingly enough, was, “A Wandering Mind Is an Unhappy Mind.” The participants indicated that they were significantly unhappier when they were daydreaming than when they were performing a certain activity.
It is quite possible that we are a lot happier when we are fully immersed in our work than when we are daydreaming.
These results have interesting consequences for people who, in their pursuit of the perfect life, believe that never having to work a single day again would make them happy. Although this is very much open to interpretation — happiness is a relative concept and one that is notoriously difficult to measure , and the sample taken was not entirely representative of the population as a whole — it is quite possible that we are a lot happier when we are fully immersed in our work than when we are daydreaming. This idea can also be found in many forms of relaxation therapy in which people are advised to focus on the activity currently occupying their mind (so that they can “be in the moment”), and the Harvard study is often cited in support of such techniques. As the scientists themselves so succinctly concluded, “A human mind is a wandering mind, and a wandering mind is an unhappy mind.”
There are significant differences between individuals when it comes to how much time we spend daydreaming. We are all familiar with the image of a child staring out of a classroom window into the distance. You may even be a dreamer yourself. In which case you may not be surprised to learn that daydreaming can have a negative effect on your ability to perform a specific task. It may come as a surprise, however, that on average people who are inclined to daydream a lot have a lower working memory capacity and score worse on IQ tests . That said, we must remember that we are talking about a correlation here and it does not necessarily mean that daydreaming leads to a lower level of intelligence, although there is a strong relationship between the two. And that makes sense — you need a good working memory to be able to maintain your concentration, after all. Given the fact that you suppress your default network when you are concentrating, this automatically means that your default network becomes activated again when you suffer a lapse in concentration, and then you start daydreaming. You could say that daydreaming is actually concentration gone wrong.
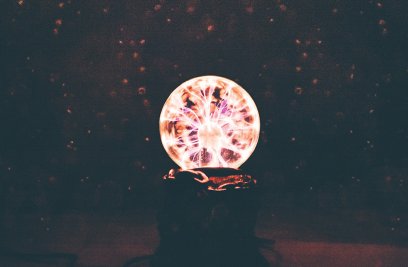
In another excellent daydreaming experiment , test subjects were asked at random moments while performing a task whether their attention was actually focused on the task or whether their mind was somewhere else entirely. The results showed, perhaps unsurprisingly, that the periods of reduced attention were the periods during which the most mistakes were made, but they also offered a possible route out of the daydreaming maze: the prospect of a reward. When test subjects knew that they would be given a reward if their performance was good, not only did their performance improve, but they were also less inclined to daydream. So the next time you want to concentrate intensively on your work and avoid daydreaming, you could try promising yourself a reward.
So is it all bad news when it comes to daydreaming? Well, some scientists believe that daydreaming also serves important functions. For example, when you are less preoccupied with the world around you, it is easier to focus on yourself and make plans for the future. When people are asked what their daydreams are about, many say that they often concern personal matters, otherwise known as autobiographical planning . These kinds of thoughts can serve important functions for our well-being, especially when the task on which you are supposed to be focusing is not especially important or does not require all that much attention.
When test subjects knew that they would be given a reward if their performance was good, not only did their performance improve, but they were also less inclined to daydream.
Another benefit of daydreaming is that it can make a mind-numbing task more enjoyable. In one study, a team of cognitive neuroscientists found that after test subjects had been asked to perform a very tedious task for 45 minutes, they reported feeling less happy than they did beforehand. However, the drop in the level of happiness was less pronounced among those test subjects who reported having daydreamed during the task. A potential solution for boredom is often included on the list of possible functions of daydreaming. You could regard the brain as a machine that always has to be doing something. So when you’re killing time, you might allow your thoughts to whisk you away briefly to some imaginary future.
One of the most important possible functions often attributed to daydreaming is the stimulation of creativity, thinking up new ideas and taking the time to solve complicated problems — the power of the unconscious. Books about concentration and creativity often advise readers to daydream and let ideas appear of their own accord. The theory goes that when you are daydreaming, your unconscious mind goes about solving your problems for you. In fact, the argument goes, it would be better to leave this kind of thing to your unconscious mind altogether.
But this claim is one worth investigating. There is no doubt that taking a break during work so that you can come back later and tackle a problem with a clear mind is a good idea. That much is intuitive. But can unconscious processes really solve problems for you or unleash your creativity? This argument is based primarily on the findings of the psychologist Ap Dijksterhuis, who has written a number of influential scientific books and articles on this subject. His findings are surprising, given what we already know about the human brain. The functions of calculation and reasoning are the domain of the working memory and they require concentration. The working memory is the place where all of the information in the brain is brought together and where the tools we need to be able to consider that information are located. This is also the information of which we are conscious, and on the basis of these definitions there cannot be any such thing as “unconscious thinking.” After all, that would mean that we also have an “unconscious” working memory that is just as powerful as our conscious working memory. In recent years, the findings of Dijksterhuis and his colleagues have come under increasing scrutiny . It turns out there is no clear evidence to suggest that the brain can solve problems on its own while you are taking a mental siesta.
However, for many of us, a mental pause can have a positive effect, hence the often-heard suggestion that we should “sleep on it” when faced with making an important decision. Switching your attention to something else can give you the time you need to approach a matter from a different perspective and maybe even reach a different conclusion. After all, the brain can become exhausted from the effort involved in trying to ignore all of the stimuli, both internal and external, with which it is constantly being bombarded. So the next time you need a reset, go outside and let your mind wander. Who knows what might happen in your stream of consciousness.
Stefan Van der Stigchel is Professor of Cognitive Psychology at Utrecht University and the author of “ Concentration: Staying Focused in Times of Distraction ,” from which this article is adapted.
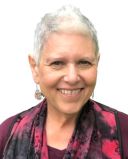
Our Minds Wander at Least 30 Percent of the Time
Mind wandering is more common than we think, and it isn't always a bad thing..
Posted January 10, 2013
You are driving to work, thinking about work, and home and your weekend plans, and before you know it, you have pulled into the parking lot of your office. You are surprised to realize that you don’t even remember the drive. The last thing you really remember is getting into your car.
What happened during the 20 minutes you were driving? You were “ mind wandering .”
How common is mind wandering? — If you ask people that question they estimate that mind wandering happens 10% of the time. But it’s actually much more common. According to Jonathan Schooler of UC, Santa Barbara, your mind is wandering at least 30% of the time when you are doing your normal day-to-day tasks, and in some cases—for example, when driving on an uncrowded highway—it might be as high as 70%.
Mind wandering is our natural state of our brains at rest — Malia Mason recorded peoples’ brain activity and correlated that to self-reports of mind wandering. When people reported that their mind was wandering their brains showed activity in several cortical regions that are the same regions that are active when our brains are “at rest." These areas are always operating in the background. So mind wandering is a natural part of how our brains work.
The multi-tasking mind-wanderer — Mind wandering allows one part of the brain to focus on the task at hand, and another part of the brain to keep a higher goal in mind. Mind wandering might be the closest thing we have to multi-tasking. Multi-tasking doesn’t really exist, but mind wandering does allow you to switch focus from one idea to another, and then back again quickly.
The link between mind wandering and creativity — Researchers at UC, Santa Barbara have shown that people whose mind wanders a lot are more creative and better problem solvers. They are able to work on the task at hand, while simultaneously processing other information and making connections amongst ideas. Specifically, the ability to come in and out of mind wandering at will is very significant, and is the hallmark of the most creative people.
Embrace the wandering mind — Now that you know that minds wander at least a third of the time or more, what can you or should you do about it? Well, you really can't do much to stop it! Mind wandering is not all bad. Since we know that mind wandering is related to creativity, try to change your attitude about it. If someone is sitting at their desk staring into space they might be thinking about their dog, but they might also be doing creative thinking .
Christoff, Kalina, Gordon, A. M., Smallwood, J., Smith, R., and Schooler, J. 2009. “Experience sampling during fMRI reveals default network and executive system contributions to mind wandering.” Proceedings of the National Academy of Sciences 106(21): 8719–24.
Mason, Malia, Michael Norton, John Van Horn, Daniel Wegner, Scott Grafton and C. Neil Macrae. 2007. “Wandering minds: The default network and stimulus-independent thought.” Science . 315 (5810): 393-395. DOI: 10.1126/science.1131295
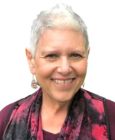
Susan Weinschenk, Ph.D. ,is a behavioral psychologist, author, coach, and consultant in neuropsychology.
- Find a Therapist
- Find a Treatment Center
- Find a Psychiatrist
- Find a Support Group
- Find Online Therapy
- United States
- Brooklyn, NY
- Chicago, IL
- Houston, TX
- Los Angeles, CA
- New York, NY
- Portland, OR
- San Diego, CA
- San Francisco, CA
- Seattle, WA
- Washington, DC
- Asperger's
- Bipolar Disorder
- Chronic Pain
- Eating Disorders
- Passive Aggression
- Personality
- Goal Setting
- Positive Psychology
- Stopping Smoking
- Low Sexual Desire
- Relationships
- Child Development
- Self Tests NEW
- Therapy Center
- Diagnosis Dictionary
- Types of Therapy
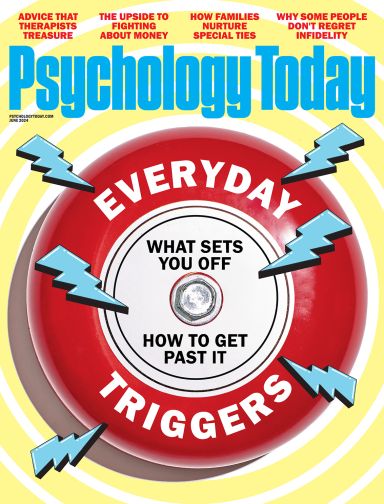
At any moment, someone’s aggravating behavior or our own bad luck can set us off on an emotional spiral that threatens to derail our entire day. Here’s how we can face our triggers with less reactivity so that we can get on with our lives.
- Emotional Intelligence
- Gaslighting
- Affective Forecasting
- Neuroscience
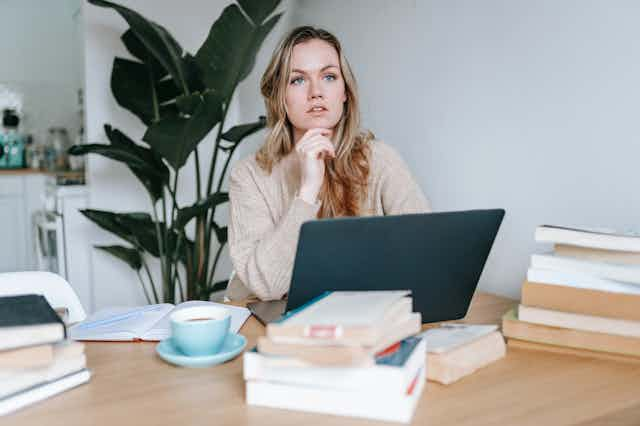
It’s normal for your mind to wander. Here’s how to maximise the benefits
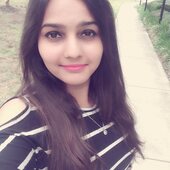
Psychology researcher, Bond University
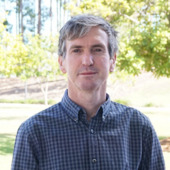
Associate Professor in Psychology, Bond University
Disclosure statement
The authors do not work for, consult, own shares in or receive funding from any company or organisation that would benefit from this article, and have disclosed no relevant affiliations beyond their academic appointment.
Bond University provides funding as a member of The Conversation AU.
View all partners
Have you ever found yourself thinking about loved ones during a boring meeting? Or going over the plot of a movie you recently watched during a drive to the supermarket?
This is the cognitive phenomenon known as “ mind wandering ”. Research suggests it can account for up to 50% of our waking cognition (our mental processes when awake) in both western and non-western societies .
So what can help make this time productive and beneficial?
Mind wandering is not daydreaming
Mind wandering is often used interchangeably with daydreaming. They are both considered types of inattention but are not the same thing.
Mind wandering is related to a primary task, such as reading a book, listening to a lecture, or attending a meeting. The mind withdraws from that task and focuses on internally generated, unrelated thoughts.
On the other hand, daydreaming does not involve a primary, active task. For example, daydreaming would be thinking about an ex-partner while travelling on a bus and gazing out the window. Or lying in bed and thinking about what it might be like to go on a holiday overseas.
If you were driving the bus or making the bed and your thoughts diverted from the primary task, this would be classed as mind wandering.
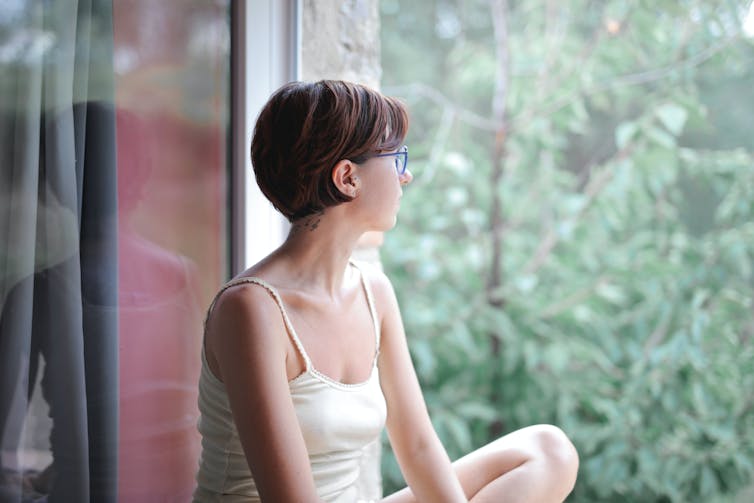
The benefits of mind wandering
Mind wandering is believed to play an important role in generating new ideas , conclusions or insights (also known as “aha! moments”). This is because it can give your mind a break and free it up to think more creatively.
This type of creativity does not always have to be related to creative pursuits (such as writing a song or making an artwork). It could include a new way to approach a university or school assignment or a project at work. Another benefit of mind wandering is relief from boredom, providing the opportunity to mentally retreat from a monotonous task.
For example, someone who does not enjoy washing dishes could think about their upcoming weekend plans while doing the chore. In this instance, mind wandering assists in “passing the time” during an uninteresting task.
Mind wandering also tends to be future-oriented. This can provide an opportunity to reflect upon and plan future goals, big or small. For example, what steps do I need to take to get a job after graduation? Or, what am I going to make for dinner tomorrow?
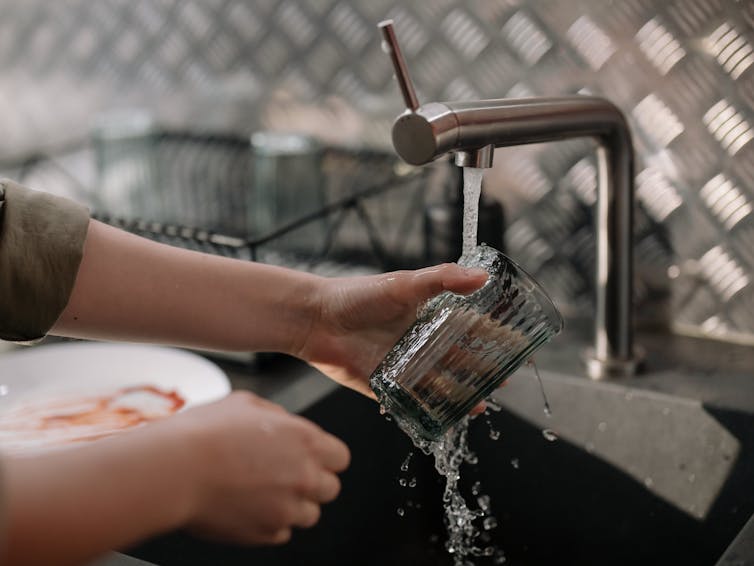
Read more: Alpha, beta, theta: what are brain states and brain waves? And can we control them?
What are the risks?
Mind wandering is not always beneficial, however. It can mean you miss out on crucial information. For example, there could be disruptions in learning if a student engages in mind wandering during a lesson that covers exam details. Or an important building block for learning.
Some tasks also require a lot of concentration in order to be safe. If you’re thinking about a recent argument with a partner while driving, you run the risk of having an accident.
That being said, it can be more difficult for some people to control their mind wandering. For example, mind wandering is more prevalent in people with ADHD.
Read more: How your brain decides what to think
What can you do to maximise the benefits?
There are several things you can do to maximise the benefits of mind wandering.
- be aware : awareness of mind wandering allows you to take note of and make use of any productive thoughts. Alternatively, if it is not a good time to mind wander it can help bring your attention back to the task at hand
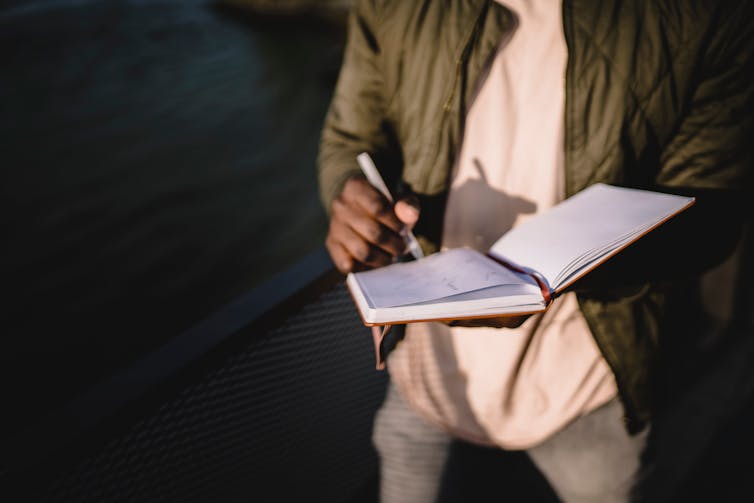
context matters : try to keep mind wandering to non-demanding tasks rather than demanding tasks. Otherwise, mind wandering could be unproductive or unsafe. For example, try think about that big presentation during a car wash rather than when driving to and from the car wash
content matters : if possible, try to keep the content positive. Research has found , keeping your thoughts more positive, specific and concrete (and less about “you”), is associated with better wellbeing. For example, thinking about tasks to meet upcoming work deadlines could be more productive than ruminating about how you felt stressed or failed to meet past deadlines.
- Consciousness
- Daydreaming
- Concentration
- Mind wandering

Social Media Producer

Student Recruitment & Enquiries Officer

Dean (Head of School), Indigenous Knowledges

Senior Research Fellow - Curtin Institute for Energy Transition (CIET)

Laboratory Head - RNA Biology
The costs and benefits of mind-wandering: a review
Affiliation.
- 1 Department of Psychological & Brain Sciences.
- PMID: 23458547
- DOI: 10.1037/a0031569
Substantial evidence suggests that mind-wandering typically occurs at a significant cost to performance. Mind-wandering-related deficits in performance have been observed in many contexts, most notably reading, tests of sustained attention, and tests of aptitude. Mind-wandering has been shown to negatively impact reading comprehension and model building, impair the ability to withhold automatized responses, and disrupt performance on tests of working memory and intelligence. These empirically identified costs of mind-wandering have led to the suggestion that mind-wandering may represent a pure failure of cognitive control and thus pose little benefit. However, emerging evidence suggests that the role of mind-wandering is not entirely pernicious. Recent studies have shown that mind-wandering may play a crucial role in both autobiographical planning and creative problem solving, thus providing at least two possible adaptive functions of the phenomenon. This article reviews these observed costs and possible functions of mind-wandering and identifies important avenues of future inquiry.
Publication types
- Research Support, N.I.H., Extramural
- Research Support, Non-U.S. Gov't
- Research Support, U.S. Gov't, Non-P.H.S.
- Attention / physiology*
- Cost-Benefit Analysis*
- Memory, Short-Term / physiology*
- Thinking / physiology*
Grants and funding
- R305H030235/PHS HHS/United States
Featured Topics
Featured series.
A series of random questions answered by Harvard experts.
Explore the Gazette
Read the latest.

Testing fitness of aging brain

DNR orders for Down syndrome patients far exceeded pandemic norm

Researchers reverse hair loss caused by alopecia
Harvard psychologists Matthew A. Killingsworth (right) and Daniel T. Gilbert (left) used a special “track your happiness” iPhone app to gather research. The results: We spend at least half our time thinking about something other than our immediate surroundings, and most of this daydreaming doesn’t make us happy.
Kris Snibbe/Harvard Staff Photographer
Wandering mind not a happy mind
Steve Bradt
Harvard Staff Writer
About 47% of waking hours spent thinking about what isn’t going on
People spend 46.9 percent of their waking hours thinking about something other than what they’re doing, and this mind-wandering typically makes them unhappy. So says a study that used an iPhone Web app to gather 250,000 data points on subjects’ thoughts, feelings, and actions as they went about their lives.
The research, by psychologists Matthew A. Killingsworth and Daniel T. Gilbert of Harvard University, is described this week in the journal Science .
“A human mind is a wandering mind, and a wandering mind is an unhappy mind,” Killingsworth and Gilbert write. “The ability to think about what is not happening is a cognitive achievement that comes at an emotional cost.”
Unlike other animals, humans spend a lot of time thinking about what isn’t going on around them: contemplating events that happened in the past, might happen in the future, or may never happen at all. Indeed, mind-wandering appears to be the human brain’s default mode of operation.
To track this behavior, Killingsworth developed an iPhone app that contacted 2,250 volunteers at random intervals to ask how happy they were, what they were currently doing, and whether they were thinking about their current activity or about something else that was pleasant, neutral, or unpleasant.
Subjects could choose from 22 general activities, such as walking, eating, shopping, and watching television. On average, respondents reported that their minds were wandering 46.9 percent of time, and no less than 30 percent of the time during every activity except making love.
“Mind-wandering appears ubiquitous across all activities,” says Killingsworth, a doctoral student in psychology at Harvard. “This study shows that our mental lives are pervaded, to a remarkable degree, by the nonpresent.”
Killingsworth and Gilbert, a professor of psychology at Harvard, found that people were happiest when making love, exercising, or engaging in conversation. They were least happy when resting, working, or using a home computer.
“Mind-wandering is an excellent predictor of people’s happiness,” Killingsworth says. “In fact, how often our minds leave the present and where they tend to go is a better predictor of our happiness than the activities in which we are engaged.”
The researchers estimated that only 4.6 percent of a person’s happiness in a given moment was attributable to the specific activity he or she was doing, whereas a person’s mind-wandering status accounted for about 10.8 percent of his or her happiness.
Time-lag analyses conducted by the researchers suggested that their subjects’ mind-wandering was generally the cause, not the consequence, of their unhappiness.
“Many philosophical and religious traditions teach that happiness is to be found by living in the moment, and practitioners are trained to resist mind wandering and to ‘be here now,’” Killingsworth and Gilbert note in Science. “These traditions suggest that a wandering mind is an unhappy mind.”
This new research, the authors say, suggests that these traditions are right.
Killingsworth and Gilbert’s 2,250 subjects in this study ranged in age from 18 to 88, representing a wide range of socioeconomic backgrounds and occupations. Seventy-four percent of study participants were American.
More than 5,000 people are now using the iPhone Web app .
Share this article
You might like.
Most voters back cognitive exams for older politicians. What do they measure?

Co-author sees need for additional research and earlier, deeper conversations around care

Treatment holds promise for painlessly targeting affected areas without weakening immune system
Binge eating appears more widespread, persistent than thought
New research takes broader, deeper look at common, but poorly understood, disorder
From the Lab to the Wild: Examining Generalizability of Video-based Mind Wandering Detection
- Open access
- Published: 17 June 2024
Cite this article
You have full access to this open access article
- Babette Bühler ORCID: orcid.org/0000-0003-1679-4979 1 ,
- Efe Bozkir 2 , 6 ,
- Patricia Goldberg 1 ,
- Ömer Sümer 3 ,
- Sidney D’Mello 4 ,
- Peter Gerjets 5 ,
- Ulrich Trautwein 1 &
- Enkelejda Kasneci 6
61 Accesses
7 Altmetric
Explore all metrics
Student’s shift of attention away from a current learning task to task-unrelated thought, also called mind wandering, occurs about 30% of the time spent on education-related activities. Its frequent occurrence has a negative effect on learning outcomes across learning tasks. Automated detection of mind wandering might offer an opportunity to assess the attentional state continuously and non-intrusively over time and hence enable large-scale research on learning materials and responding to inattention with targeted interventions. To achieve this, an accessible detection approach that performs well for various systems and settings is required. In this work, we explore a new, generalizable approach to video-based mind wandering detection that can be transferred to naturalistic settings across learning tasks. Therefore, we leverage two datasets, consisting of facial videos during reading in the lab (N = 135) and lecture viewing in-the-wild (N = 15). When predicting mind wandering, deep neural networks (DNN) and long short-term memory networks (LSTMs) achieve F \(_{1}\) scores of 0.44 (AUC-PR = 0.40) and 0.459 (AUC-PR = 0.39), above chance level, with latent features based on transfer-learning on the lab data. When exploring generalizability by training on the lab dataset and predicting on the in-the-wild dataset, BiLSTMs on latent features perform comparably to the state-of-the-art with an F \(_{1}\) score of 0.352 (AUC-PR = 0.26). Moreover, we investigate the fairness of predictive models across gender and show based on post-hoc explainability methods that employed latent features mainly encode information on eye and mouth areas. We discuss the benefits of generalizability and possible applications.
Avoid common mistakes on your manuscript.
Introduction
Attention plays a central role in learning and knowledge construction (Levine, 1990 ). However, a recent meta-analysis by Wong et al. ( 2022 ) showed that about 30% of the time learners spend in educational activities, their thoughts are elsewhere. This shift of attention away from the current task to task-unrelated thought is called mind wandering (Smallwood & Schooler, 2006 ). Wong et al. ( 2022 ) further demonstrated that frequent occurrence of task-unrelated thoughts during learning is significantly associated with lower test performance and explains about 7% of the variability in learning outcomes. This negative relationship holds equally for surface and inference-level learning and is consistent across tasks. For instance, mind wandering has shown to have a negative effect on reading comprehension (Smallwood, 2011 ; Feng et al., 2013 ; D’Mello & Mills, 2021 ; Bonifacci et al., 2022 ; Caruso & D’Mello, 2023 ) and lecture retention (Risko et al., 2012 ; Szpunar et al., 2013 ; Hollis & Was, 2016 ; Pan et al., 2020 ).
This evident effect of mind wandering on learning should not be neglected. Therefore, learning environments - physical as well as online - aim to create appealing conditions that allow students to focus their attention on the relevant content and support successful learning. The Covid19 pandemic has greatly accelerated the use (Lemay et al., 2021 ) and development (Dhawan, 2020 ) of online learning tools at all levels of education. These include intelligent tutoring systems (ITSs), massive open online courses (MOOCs), as well as online lecture portals. To support learners in online learning settings, one can either improve the presented learning materials in a way that decreases mind wandering, for instance, by making texts more interesting (Bonifacci et al., 2022 ), or one can try to direct attention back to the learning task, for instance, through targeted interventions (e.g., Mills et al., 2021 ).
One step that is foundational to those two approaches to support learners is the automated detection of mind wandering, as it allows for continuous and unobtrusive measurement of the state of attention over time. It can be used to test and optimize learning materials and to conduct further research on the conditions under which mind wandering occurs and its effects on learning outcomes. At the same time, it offers online learning systems the possibility to implement targeted interventions to respond to the learners’ shift of attention with adaptive and supporting actions. It has been demonstrated that such automated interventions can reduce mind wandering and thus support learning. For example, feedback following eye-tracking-based mind wandering detection mitigated its negative effect on reading comprehension during computerized reading (D’Mello et al., 2017 ; Mills et al., 2021 ). Furthermore, repetition and questioning interventions based on automatically detected mind wandering reduced mind wandering and improved retention of students with low prior knowledge in an ITS in certain cases (Hutt et al., 2021 ).
Mind wandering detectors using supervised machine learning mostly rely on data from modalities such as eye trackers (Hutt et al., 2017 ; Faber & D’Mello, 2018 ; Hutt et al., 2019 ; Faber et al., 2020 ; Zhang et al., 2020 ; Mills et al., 2021 ) or physiological sensors such as EEG (Jin et al., 2019 ; Dong et al., 2021 ). While these modalities provide very useful process information, the use of such sensors requires a well-controlled environment, is quite costly, and is difficult to scale. However, another strand of recent research has shown that mind wandering can also be detected above chance level using video recordings of the face obtained from consumer-grade webcams, such as those found in almost all laptops (Bosch & D’Mello, 2021 ; Lee et al., 2022 ). The use of video recordings enables the detection of mind wandering on a large scale in natural environments where online learning systems are commonly used, such as classrooms or homes.
Video-based mind wandering detectors have the potential to be used in many different systems and environments. Intelligent user interfaces for learning can combine multiple stimuli, such as text and video, and may be used globally, i.e., by culturally diverse target groups. However, to train suited machine-learning models, labeled ground truth data is needed, i.e., collecting learner self-reports makes the whole process time-consuming, effortful, and costly. Thus, approaches that generalize well across different settings, learning tasks, and target groups are required in order to ensure the applicability of such solutions.
To obtain generalizability, facial features rather than gaze features, which are highly predictive but also highly stimulus dependent (Faber et al., 2020 ), are suitable as they can achieve greater transferability between tasks. In this study, we use transfer-learning-based features trained on a dataset of facial expressions in the wild (Mollahosseini et al., 2017 ), i.e., on a highly diverse set of facial images. Previous research has shown that affective features such as facial action units (AUs) and predictions of emotional state are informative for predicting mind wandering (Stewart et al., 2017a ; Bosch & D’Mello, 2021 ). However, this could pose a challenge when thinking about generalizing models across subject groups with different cultural backgrounds, as there is an ongoing scientific debate about the universality of facial expressions of emotion across different cultures (Russell, 1994 ; Ekman, 1994 ; Jack et al., 2012 ). This could have implications for cross-cultural generalizability when using features derived from such classification tasks. This highlights one of the major limitations of previous approaches-namely, the lack of sample diversity and the unexplored effects on algorithms, which may bias results and impact generalizability (Kuvar et al., 2023 ).
The goal of the present work is to examine the generalizability of video-based mind wandering detection. Towards this goal, we investigate whether (1) a new feature set based on transfer learning of facial-expression recognition can be used in combination with temporal models exploiting temporal relationships in video data to improve model performance compared to explicit facial features. Furthermore, (2) we explore the potential of its generalization across two datasets that differ with regard to the environment (lab vs. in the wild), task (reading vs. watching a video lecture), and cultural background of the target groups (American vs. Korean students). We then (3) examine the fairness of our models across genders and (4) use explainable AI tools to investigate the information encoded in latent features. Accurate detection of mind wandering is the first critical step towards large-scale research and adaptive learning technologies that aim to enhance engagement and learning outcomes.
Related Work
The automated detection of mind wandering episodes allows measuring this state non-obtrusively and continuously over time. This is achieved by employing machine learning methods and self-reports from learners as ground truth. Here, self-reports are used because an objective, reliable measurement by neurophysiological or behavioral markers is not possible so far (Smallwood & Schooler, 2015 ). When collecting self-reports with the probe-caught method, subjects are repeatedly interrupted by a probe and explicitly asked about the direction of their attention (Smallwood & Schooler, 2006 ), while in the self-caught method, participants are instructed to report whenever they become aware of their own shift of attention (Schooler et al., 2011 ). Research suggests that both approaches allow for reliable measurement of mind wandering (Schubert et al., 2020 ; Varao-Sousa & Kingstone, 2019 ). Self-reports are associated with physiological signals (Franklin et al., 2013 ; Blanchard et al., 2014 ; Christoff et al., 2009 ) and consistently correlate with objective performance measures (Randall et al., 2014 ), demonstrating predictive validity. Moreover, the datasets on which mind wandering is examined are all very unbalanced with approximately 25-30% mind wandering rates. Therefore, the results of the prediction are reported using the F \(_1\) measure, which represents a harmonized mean of precision (proportion of predicted mind wandering instances that are truly mind wandering) and recall (proportion of true mind wandering instances predicted as mind wandering). The reported improvement over chance level is the proportion of above chance performance of the perfect above chance prediction. For more details on the evaluation metrics, we refer the reader to “ Evaluation Metrics ”.
Most studies on automated mind wandering can be divided into two main strands according to the sensing modalities used for the detection: Eye tracking and physiological measures, and video recordings. Therefore, in the following, we review this research focusing on these areas and describe the novelty of our study.
Eye-tracking and Physiological Sensor-based Approaches
Most research on mind wandering detection has focused on eye movement data obtained by eye trackers. According to the so-called mind-eye link, cognitive processes are reflected in eye movements (Just & Carpenter, 1976 ; Rayner, 1998 ; Reichle et al., 2012 ) thus making eye tracking suitable to identify mind wandering. Global gaze features, such as fixations and saccades, as well as locality features describing the spatial properties of gaze have widely been used in research to estimate attentional states during a variety of learning-related tasks such as reading (Bixler & D’Mello, 2014 ; D’Mello et al., 2016 ; Faber et al., 2018 ; Mills et al., 2021 ), watching video lectures (Hutt et al., 2017 ; Jang et al., 2020 ; Zhang et al., 2020 ) or using an ITS (Hutt et al., 2019 ). Also cross-task prediction of such features was examined (Faber et al., 2020 ; Bixler & D’Mello, 2021 ). Additionally, pupil size and blink rates have been shown to be meaningful features to off-task thought detection (Smilek et al., 2010 ; Brishtel et al., 2020 ).
Further, several physiological sensors are utilized for mind wandering detection. Electrodermal activity (EDA) was used as a standalone modality (Blanchard et al., 2014 ) and in combination with eye tracking (Brishtel et al., 2020 ) for mind wandering detection during reading. Furthermore, an increased heart rate was detected in mind wandering episodes due to greater arousal (Smallwood et al., 2007 ), thus being deployed for automated detection as well (Pham & Wang, 2015 ). Another way of assessing mind wandering is using EEG (Jin et al., 2019 ; Dong et al., 2021 ; Dhindsa et al., 2019 ; Conrad & Newman, 2021 ), which has also been employed for learning related mind wandering during the watching of online lectures (Dhindsa et al., 2019 ; Conrad & Newman, 2021 ).
The collection of data employing eye trackers or physiological sensors requires highly controlled settings (i.e., laboratory). Consequently, most of the studies were conducted in a lab setting, with the exception of Hutt et al. ( 2019 ); who used commercial eye trackers in a classroom setting. Furthermore, respective modalities are often very expensive, which again limits scalability. The alternative of video-based detection is more cost-effective. Consumer-grade webcams can be employed and thus detection can be upscaled easily in naturalistic settings (e.g., at home or in the classroom). Therefore, in this study, we focus on mind wandering detection based only on facial videos.

Video-based Approaches
The first approach to detect mind wandering based on facial videos from webcams was provided back in 2017 by Stewart et al. ( 2017a ). The authors predicted self-reported mind wandering during narrative film watching in a laboratory setting, based on features such as AUs, head pose, face position, face size, and gross body movement. Employing support vector machine (SVM) models, they achieved an F \(_{1}\) score of 0.39, which is an improvement of 13% above chance level, on aggregated features from 45-second windows. In a following study, the potential of cross-task classification in laboratory settings was shown, by predicting mind wandering on a reading task from a model trained on mind wandering from a film watching task and vice versa (Stewart et al., 2017b ). Employing the same feature set as in the previous study and a decision tree based C4.5 classifier, their models generalized well and almost maintained within-dataset prediction performance when training on film watching data and predicting reading data (F \(_{1}\) : 0.407; 21% above chance level) and also after adjusting the classification threshold the other way around (F \(_{1}\) : 0.441; 22% above chance level).
In a laboratory experiment to detect mind wandering during MOOCs, Zhao et al. ( 2017 ) implemented webcam-based gaze estimation and compared it to predictions with specialized eye-tracking data. With probe-caught mind wandering reports as ground truth they concluded that SVM classifiers on both data sources perform equally well with the webcam-based approach achieving an F \(_{1}\) score of 0.405, a 16% above-chance improvement.
Another recent study by Bosch & D’Mello ( 2021 ) examined face-based mind wandering detection in the laboratory during a reading task with self-caught mind wandering reports and in the classroom during the usage of an ITS based on probe-caught mind wandering reports. In addition to AUs, head pose, and body movement, they hand-crafted new features depicting co-occurring AUs, temporal dynamics of AUs, and facial texture. In the classroom setting, those features were extracted in real-time, avoiding the recording of children due to privacy concerns. With SVM and deep neural networks on aggregated features sets over 10-second windows, they achieved F \(_{1}\) scores of 0.478 in the lab and 0.414 in the classroom setting, which represents 25% and 20% above-chance improvements respectively. Although eye-tracking features (Global gaze features F \(_{1}\) : 0.45, 29% above-chance accuracy; Locality gaze features F \(_{1}\) : 0.49, 34% above-chance accuracy) outperform a facial-feature based approach (AUs F \(_{1}\) : 0.31, 10% above-chance accuracy; Co-occurring AUs F \(_{1}\) : 0.3, 9% above-chance accuracy) for mind wandering detection while using an intelligent tutor system in the same classroom setting (Hutt et al., 2019 ), a fusion of both features could increase robustness by accounting for missing values in one of the two modalities.
In a recent paper by Lee et al. ( 2022 ) mind wandering detection based on facial webcam videos during lecture viewing in the wild, for example at home, was examined. Gaze-related features (i.e., speed, dispersion, horizontal movement ratio), Eye Aspect Ratio (EAR) features, head movement, as well as emotion predictions, were extracted from facial videos. They employed eXtreme Gradient Boosting (XGBoost), Deep Neural Network (DNN), and SVM classifiers on different time windows, achieving the best results with XGBoost with an F \(_{1}\) score of 0.36 (15% over chance level improvement) utilizing 10-second windows.
Adopting webcam-based eye tracking for reading tasks, Hutt et al. ( 2023 ) executed two in-the-wild studies: the first recruited participants through a university, and the second utilized Prolific for participant recruitment. They predicted probe-caught mind wandering using global and local gaze features, achieving an F \(_{1}\) -score of 0.25 on a combined dataset (9% above chance). In cross-dataset prediction between two data collections, training on one dataset and predicting on the other, they achieved Kappa values of 0.09 and 0.15, respectively.
The overwhelming majority of these studies, except one cross-task laboratory-based generalization study (Stewart et al., 2017b ) and a very recent webcam-eye-tracking study (Hutt et al., 2023 ), consider the performance of their models only on the labeled data on which they were trained and therefore do not test the generalizability of their models. As mentioned above, collecting labeled data for this specific task is costly and may not be possible for every use case, implying that generalizable models are necessary.
Novelty of this Work
While a large variety of machine learning and computer vision methods are used for feature extraction, they are not directly targeted to generalizability. Hence, there are still unexploited potentials of machine learning techniques, which we investigate in this study. These techniques include utilizing features from pre-trained networks for similar but more general tasks, employing temporal models, and an evaluation of generalizability, which are discussed as follows.
Deep Learning-based Features
To our knowledge, all previous research in this field used explicit features, such as AUs (Stewart et al., 2017a ; Bosch & D’Mello, 2021 ) and gaze features (Lee et al., 2022 ) for predictions. In some cases, additional hand-manufactured features, such as gaze dispersion (Lee et al., 2022 ), have been created. While those appear to be informative in the data at hand, there is a risk that some of those features, especially gaze-related features, might be very stimulus-specific. Gaze in a reading task has a highly specific signature, whose features may be difficult to transfer to different tasks (Bixler & D’Mello, 2021 ; Faber et al., 2020 ). While there have been advances with webcam-based eye tracking in recent years, enabling the detection of fixations of specific areas of interest (AOIs) on the computer screen, there are still severe limitations compared to specialized eye trackers. Especially in terms of mind wandering detection, more fine granular eye movement indicators such as saccade duration are predictive and most likely more generalizable. Those cannot be observed based on consumer-grade webcams at this time, underlining the advantages of facial expression features. Moreover, recent studies have showcased the utility of advanced Facial Emotion Recognition (FER) methods on webcam videos in adjacent domains, particularly for evaluating emotion regulation in remote collaborative learning settings (Nguyen et al., 2022a ; Ngo et al., 2024 ). Further, the creation of hand-manufactured features requires domain knowledge and might be tailored to the setting at hand.
In other image-based classification tasks such as facial expression recognition, deep learning methods have been used successfully (Rawat & Wang, 2017 ; Li & Deng, 2020 ). Due to the limited sample sizes of data for mind wandering detection, we use transfer learning, where the feature extraction part of networks pre-trained on a similar task with available large datasets, is applied to a new problem. Based on the previous successful use of facial expression features as facial texture patches and AUs (Bosch & D’Mello, 2021 ) and emotional state features (Lee et al., 2022 ) for mind wandering detection, we argue that latent features learned by a CNN trained on the related task of FER can be informative to the mind wandering classification problem at hand. Furthermore, as they are trained on an in-the-wild data set, which assures large data variability, and thus utilizing these features may contribute to making the models generalizable to in-the-wild settings, i.e. in terms of image quality. To increase the confidence in these latent features, which cannot be directly interpreted by humans, we use the Explainable AI tool LIME (Ribeiro et al., 2016 ) to illustrate which parts of the face are used for classification and are encoded respectively in our latent representation.
Temporal Models
Previous research indicates that temporal dynamics can be informative for mind wandering detection. Recent studies showed the importance of hand-crafted features such as co-occurring AU pairs and temporal dynamics of AUs (Bosch & D’Mello, 2021 ), as well as body (Stewart et al., 2017a ; Bosch & D’Mello, 2021 ) and head movement (Lee et al., 2022 ), which are explicitly designed to depict these dynamics. If not represented by such manually generated features, they might be blurred by aggregation over time. For this reason, we employ models that are able to take time-series data such as the video data at hand as input and directly learn temporal relations between features. The use of these temporal models allows us to additionally train an end-to-end model, in which we can fine-tune the pre-trained Convolutional Neural Network (CNN) used for frame-wise feature extraction to our specific classification task.
Generalizability
In previous face-based mind wandering detection research, only cross-task prediction in laboratory settings was explored (Stewart et al., 2017b ), but the generalizability of lab settings to other more naturalistic settings is still to be examined. We trained a model on data collected during a reading task in the lab (Bosch & D’Mello, 2021 ) and then applied it to in-the-wild data of students watching a lecture video at home (Lee et al., 2022 ), resulting in a cross-context and cross-task prediction. However, the two data sets differ additionally in the cultural backgrounds of their subjects, as one was collected in the U.S. and the other in Korea. With regard to the discussion about the cross-cultural universality of facial expressions in the literature (Russell, 1994 ; Ekman, 1994 ; Benitez-Garcia et al., 2017 ), this is a further challenge for our model, which is based on such features to generalize across culturally diverse user groups. Although the in-the-wild dataset is relatively modest in size, it represents to our knowledge the only publicly available dataset of its kind, enabling a crucial initial stride towards achieving generalizability in naturalistic environments. For comparability, we used the reading-task data to carry out within-context and within-task evaluation. We further compare the classification results for both across gender to investigate potential biases.
Methodology
In this section, we discuss the details of the employed data, features that are utilized for mind wandering detection, as well as training and inference processes.
We employ two different datasets in our work, one (Bosch & D’Mello, 2021 ) for within-dataset evaluation and the other (Lee et al., 2022 ) for cross-dataset evaluation. Table 1 provides a general overview of the datasets, and they are described as follows.
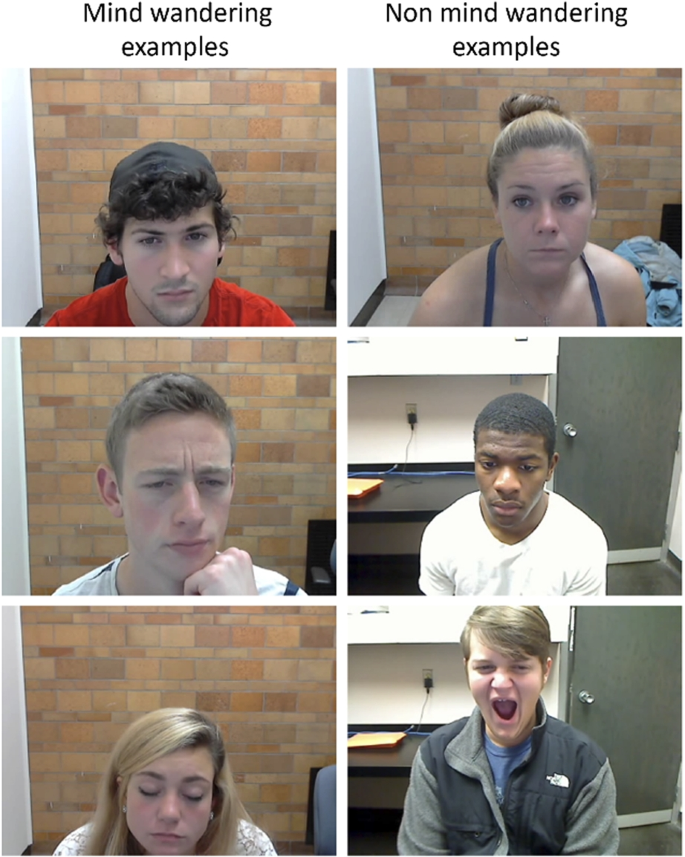
Example images of mind wandering (left) and non mind wandering (right) instances in the lab data (Bosch & D’Mello, 2021 )
Lab Data by Bosch & D’Mello ( 2021 )
This dataset is from a lab study, containing facial videos, recorded using a Logitech C270 webcam, of \(N = 135\) university students from the U.S. reading a scientific text and their self-caught reports on mind wandering. Mind wandering instances and on-task moments of 10 s each were cut from the original videos (Fig. 1 ) (Bosch & D’Mello, 2021 ). The mind wandering instances are the time windows right before a self-report, with a 4 s buffer before the self-report, to ensure the exclusion of the key-press movement. The buffer length was validated in a pilot study. The on-task examples are 10-second clips taken from the time in-between, that do not include a page turn or fall into 30 s before a mind wandering self-report. The resulting dataset contains \(N = 1031\) mind wandering instances and \(N = 2406\) non-mind wandering instances. We use this dataset for training our models in both within- and cross-task evaluation scenarios as it contains more subjects and samples.
In-the-wild Data by Lee et al. ( 2022 )
This open-source dataset available for research purposes contains facial videos of \(N = 15\) university students in Korea and probe-based mind wandering reports. The participants watched a one-hour-long lecture video at home, were probed for mind wandering in 40-second intervals, and were filmed by their webcams. In contrast to previously employed datasets, the data was collected in the wild (i.e., at students’ homes), which is an increasingly realistic learning setting for MOOCs and other online learning tools. In total, it contains \(N = 205\) mind wandering and \(N = 1009\) non-mind wandering instances with 30 FPS. Due to the smaller dataset size, we use this dataset solely for evaluation purposes to detect mind wandering in the wild.
To extract deep learning-based facial expression features, we use a CNN with a ResNet50 (He et al., 2016 ) architecture pre-trained on the AffectNet dataset (Mollahosseini et al., 2017 ) containing 23,901 images classified as belonging to seven discrete facial expressions (neutral, happy, sad, surprise, fear, disgust, anger), as a feature extractor. The process is depicted exemplarily in Fig. 2 . This model achieves an accuracy of \(58\%\) on the AffectNet validation set (see Sümer et al. ( 2021 ) for details on model training). To extract latent features from our video clips, we apply frame-wise face detection by employing RetinaFace (Deng et al., 2020 ) to our videos. In this step, we had to remove 74 instances, 31 of them mind wandering, because no face could be detected across all frames. We pre-process the resulting face images, aligning them based on five facial key points extracted by the face detector, then they are cropped to size \(224 \times 224\) and normalized. We then insert them into the pre-trained ResNet50 model, from which the FER classification layer was removed. The extracted latent feature vector is 2048 digits long and can be fed into a downstream classifier. We provide insights into the most important image areas encoded in the feature vectors by applying the Explainable AI tool LIME (Ribeiro et al., 2016 ) to the feature extraction model.
To compare our latent deep learning features to more explicit features, similar to those used in previous research, we extract AUs, facial landmark locations, head location, pose and rotation, as well as face shape parameters. Additionally, we extract gaze direction vectors for both eyes and gaze angles, as well as 2D and 3D eye region landmarks, consisting of 55 landmark points for each. All features were extracted using the OpenFace toolkit (Baltrušaitis et al., 2016 ) from each video frame respectively.
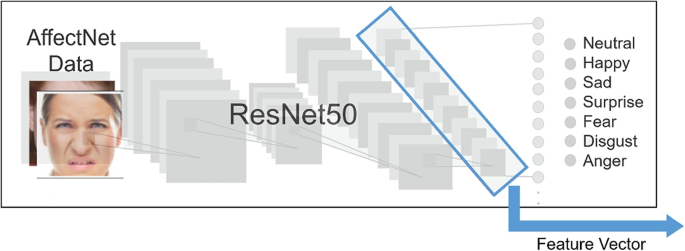
Pre-trained Resnet50 as feature extractor
Training and Inference
In our analysis approach for binary mind wandering classification, we aim to leverage pre-trained features from deep neural networks by employing transfer learning from the related task of facial expression recognition and compare this approach to employing explicit features as AUs and gaze vectors. Further, we examine whether these pre-trained features enable our model to generalize to a new in-the-wild dataset.
Handling Temporal Data
We aim to leverage temporal dynamics information which may be lost by aggregation over time in non-temporal models, by employing recurrent neural network models for supervised classification. In particular, we train long short-term memory (LSTM) (Hochreiter & Schmidhuber, 1997 ) and bidirectional long short-term memory (BiLSTM) (Baldi et al., 1999 ; Schuster & Paliwal, 1997 ) recurrent neural networks. For both models, we employ an architecture consisting of three recurrent layers with 100 neurons respectively, taking frame-wise extracted feature vectors with 125-time steps as input. A dense layer stacked on top, implementing a sigmoid activation function, outputs the binary mind wandering predictions.
The aforementioned self-reports serve as ground truth for our supervised machine learning approach. In order to compare our results in a within-task and within-context evaluation scenario, we employ the exact same person-independent 4-fold validation splits as in (Bosch & D’Mello, 2021 ). For each fold, a model is trained separately and results are averaged over all test folds. To emphasize the minority class mind wandering during training we employ class weighting. Furthermore, we employ early stopping of model training to avoid overfitting with a patience of 5 epochs.
To compare the performance of temporal models with non-temporal models, we additionally train SVMs (Cortes & Vapnik, 1995 ) with a radial basis function (RBF) kernel, which showed to be the best performing models in previous research (Bosch & D’Mello, 2021 ), as well as XGBoost (Chen & Guestrin, 2016 ) models and simple DNNs with one hidden layer (Fig. 3 ). To find the optimal parameter settings for each model, we used person-independent, nested 4-fold cross-validation to apply grid-search hyperparameter tuning. The best performing settings determined in inner 4-fold cross-validation were used for prediction in each outer fold. An overview of the parameters tested can be found in Table 7 in the appendix. To generate suitable input, we aggregate frame-wise extracted explicit features, computing the mean, median, minimum, maximum, and standard deviation values for each feature over the whole clip. For our latent features, we create statistical aggregations of the 2048-numbers long feature vector over all 125 frames. Since this procedure results in a large number of features with a lot of redundancy, we apply mutual information-feature selection, a univariate feature selection method based on the dependency between variables. Based on the training data, the 100 most meaningful features were selected in each fold. To account for the imbalanced data, we employ weighting or up-sampling of the training split using Synthetic Minority Over-sampling Technique (SMOTE) (Chawla et al., 2002 ). We report the best performing combinations of balancing and hyper-parameters.
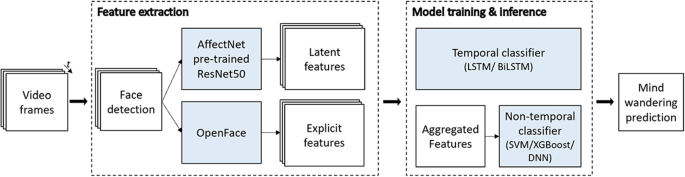
Mind wandering detection pipeline
Fine-tuned CNN-LSTM
Employing temporal models allows us to not only employ the pre-trained AffectNet-CNN as a feature extractor but also train and fine-tune an end-to-end CNN-LSTM model. This allows not only to train the temporal inference and classification parts of the model for our present problem of mind wandering detection but also to adapt the image feature extraction part of the model more precisely to the particularities of our problem. As a first step, the pre-trained ResNet50 without classification layer is included in the LSTM architecture to allow for video input and frame-wise feature extraction. Then, the previously described RNN architectures are stacked on top. In a first training run, the pre-trained weights in the CNN part of the model are frozen and only the top part of the model is allowed to adapt with a learning rate of 0.001. In a second training run, the last convolutional block of the ResNet50 model is set trainable and thus adapted to the mind wandering classification task with a smaller learning rate of 0.00001.
Cross-dataset Prediction
To examine the generalizability of the proposed approach over different settings, tasks, and target groups, we perform cross-dataset prediction employing our new approach. This means the aforementioned in-the-wild data is used to predict mind wandering instances. To this end, we use the video data from our lab reading task and train a model on the entire data. Using this model, we then predict mind wandering instances in the in-the-wild lecture viewing data from (Lee et al., 2022 ). We pre-process the in-the-wild dataset in the same way as the lab data. Based on the provided mind wandering probes, we cut 10-second windows before each probe and extracted all features described in “ Features ”. For temporal models, we downsampled the 300 frames resulting from a higher recording frame rate to 125 frames to match the sequence length.
Evaluation Metrics
Due to the unbalanced nature of both our datasets with lab data having 30% and in-the-wild data having 25% mind wandering instances, reporting the accuracies of mind wandering prediction models could be misleading. A classifier always predicting non-mind wandering would achieve 70-75% accuracy without recognizing a single mind wandering instance. Also commonly used threshold metrics focusing on the minority class as F \(_{1}\) scores are highly influenced by the skew in the data (Jeni et al., 2013 ). Such measures can be driven by high recall at low detection precision. For this reason, we report area under Precision-Recall curve (AUC-PR) values, which show the precision as function of the recall. This rank metric helps to balance precision and recall of the minority class.
Another measure that helps to evaluate the performance, especially when comparing performances of datasets with differing class distributions, is the improvement of the model above chance level, which is calculated as follows:
To allow comparability to previous research, we additionally report the F \(_{1}\) scores for the minority-class mind wandering, which is calculated as follows:
where the Precision (i.e., the proportion of correct mind wandering predictions of all mind wandering predictions) and Recall (i.e., share of correctly predicted mind wandering instances of all mind wandering instances) are defined as:
TP, FP, TN, and FN represent “true positive”, “false positive”, “true negative”, and “false negative”, respectively.
In this section, we report within- and cross-dataset mind wandering detection results and investigate potential model biases by comparing our results across gender and explain the employed latent features.
Mind Wandering Detection
The results for within-task mind wandering detection, training, and predicting on the lab data, are depicted in Table 2 . In the within-task prediction setting, models trained on latent features outperform the random baseline (i.e., \(F_{1} = 0.3\) (Bosch & D’Mello, 2021 ) and \(AUC-PR = 0.3\) ) to a significant extent.
The results are comparable to the state-of-the-art on this data (Bosch & D’Mello, 2021 ), which achieved similar performance between \(F_{1}\) scores of 0.414 and 0.478, mostly with hand-crafted features and SVMs. However, the observed \(F_{1}\) scores are clearly driven by high recall values, while precision values are rather low. While a high recall means that most mind wandering instances are detected, simultaneously low precision also means that many instances are falsely classified as mind wandering. Therefore, we introduce the \(AUC-PR\) score, which is a rank metric that also reflects the ratio of precision and recall. Thus, the above chance level values reported in the table are calculated on the basis of \(AUC-PR\) scores.
The results for cross-task mind wandering detection using in-the-wild data for evaluations are reported in Table 3 . Similar to the within-task setting, our results outperform the random baseline (i.e., \(F_{1} = 0.25\) (Lee et al., 2022 ), \(AUC-PR = 0.17\) ) despite the cross-task prediction and in-the-wild setting. State-of-the-art on this dataset (Lee et al., 2022 ) achieved \(F_{1}\) scores between 0.25 and 0.36 using SVMs, XGBoost, and DNNs for mind wandering prediction in a within-dataset setting. We achieve a very comparable performance to the best performance of the state-of-the-art (Lee et al., 2022 ) despite cross-task evaluation, a culturally different target group, and an in-the-wild setting.
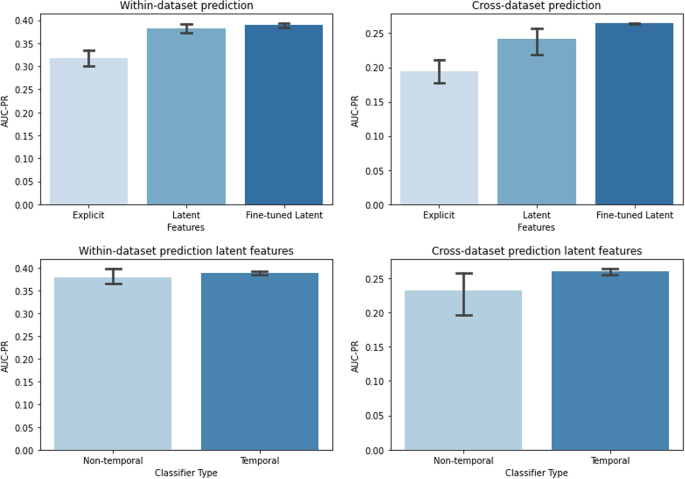
Average Performance by Feature Sets and Classifier Type
A comparison of performance along the employed feature sets over all classifiers and prediction scenarios, depicted in Fig. 4 , indicates that overall the latent features allow a better mind wandering detection. Especially in cross-dataset prediction, the fine-tuning of the latent features in an end-to-end CNN-LSTM leads to further improvement. This could be due to the fact that more data, i.e., the complete lab data set, were available for the training, hence fine-tuning, than in the case of the within the prediction. When employing latent features, the use of temporal (i.e., LSTM, BiLSTM, CNN-LSTM, CNN-BiLSTM) models, allows for a small improvement of prediction performance compared to non-temporal classifiers (i.e., SVM, XGB, DNN) (Fig. 4 , 2nd row), especially in the cross-dataset prediction.
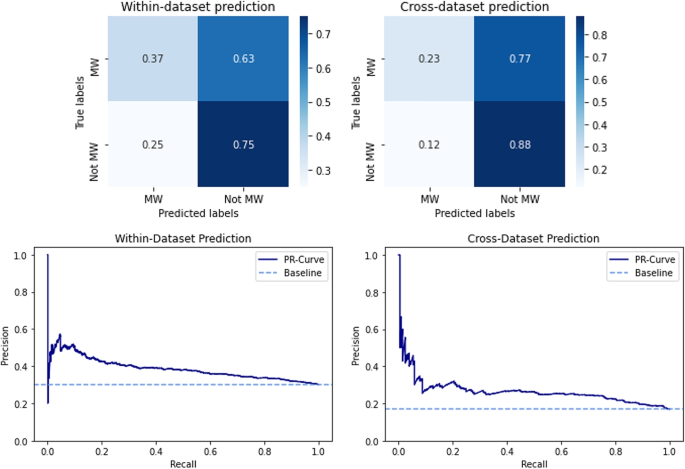
Confusion Matrices and Precision-recall Curves for Within- and Cross-dataset Prediction with CNN-BiLSTM
Figure 5 shows the confusion matrices for within- and cross-dataset mind wandering prediction of the fine-tuned CNN-BiLSTM model, as well as precision-recall curves for both prediction scenarios. The confusion matrices reveal that the models are able to detect 37% and 23% of mind wandering instances in the two scenarios. Depending on the detection use case high recall might be favored over precision, which can be achieved by lowering the prediction threshold.
When aggregating instance-by-instance predictions over time per participant, to assess performance over longer time windows, we obtain a Pearson’s correlation coefficient of 0.578 ( \(P < 0.001\) ) between true and predicted mind wandering proportion per person in the within-dataset prediction. For cross-dataset predictions, we found a correlation of 0.48 ( \(P = 0.07\) ) on a person-level. However, when adapting the prediction threshold from 0.5 to 0.3 we achieve a correlation of 0.8 ( \(P < 0.001\) ). This finding underscores the potential to enhance mind wandering detection by fine-tuning the prediction threshold for specific use cases, particularly in cross-dataset predictions that exhibit varying overall proportions of mind wandering. Consequently, we conducted systematic experimentation to optimize thresholds for enhanced detection accuracy and applicability.
Threshold Optimization
In alignment with previous research (Stewart et al., 2017b ; Faber et al., 2018 ) we employ threshold optimization to further improve the detection of mind wandering instances. Threshold optimization is a technique used to enhance the performance of a classification model by selecting the optimal cutoff value for distinguishing between the two classes. In binary classification, models often output a probability score that indicates the likelihood of an instance belonging to the positive class. The default threshold is typically set at 0.5. However, the default threshold of 0.5 may not always be the best choice for all datasets or objectives, especially in cases where there is class imbalance. Particularly in the cross-dataset scenario, where the two different datasets yielded varying mind wandering rates of 30% and 17%, threshold optimization emerges as a critical approach, enabling the adjustment of classification cutoffs to better reflect the disparate prevalence rates across datasets (Stewart et al., 2017b ).
We systematically tested thresholds from 0.1 to 0.9 in increments of 0.1 for all employed models. Tables 4 and 5 show the results for optimization according to \(F_{1}\) values for within- and cross-dataset predictions. Optimal thresholds are smaller than 0.5 for most classifiers. Those lower thresholds lead to notable improvements in \(F_{1}\) values. Figure 6 shows evaluation metrics by prediction thresholds for CNN-BiLSTM models in both prediction scenarios. We can see that \(F_{1}\) values drop at the 0.3 and 0.4 thresholds, which were identified as optimal for those models.
Comparison across Gender
To detect potential bias in the classification models and provide transparency with regard to the fairness of prediction across gender, we compare the detection performance of mind wandering by gender for the within- and cross-dataset predictions. We compare the results of all models trained on latent features for both prediction scenarios. The lab data employed for within-dataset predictions consists of 40.77% male and 59.23% female participants, with females reporting an overall higher mind wandering rate of 32.64% and males a rate of 26.41%.
The in-the-wild data, serving as an evaluation set for our cross-dataset predictions, contains 53.33% female and 46.67% male participants, reporting mind wandering in 18% and 16% of the mind wandering probes on average, respectively. The prediction results by gender are displayed in Table 6 , with above chance values based on different base rates by gender and dataset. In general, mind wandering instances are predicted more accurately for females than for males. These results are most likely rooted in the imbalance of the underlying datasets, both regarding overall gender rates as well as gender-specific mind wandering rates, leading to overall fewer male mind wandering instances in the data. However, this difference becomes larger when employing temporal models, especially for within-dataset prediction scenarios. We can assume that this is due to the increased number of parameters in the temporal models, requiring large training data. While the overall performance increases, only females seem to benefit from improved detection, as there is more training data available.

Evaluation Metrics by Prediction Thresholds for Within- and Cross-dataset Prediction with CNN-BiLSTM
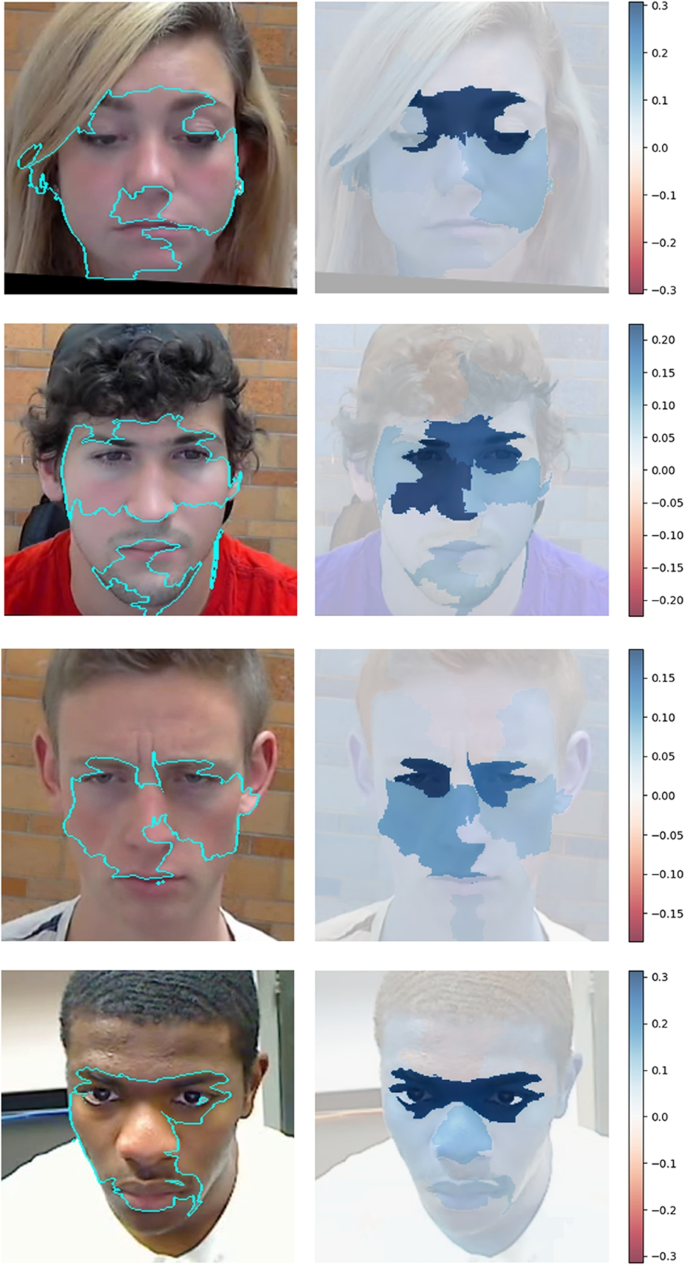
Most important super-pixel boundaries (left) and super-pixel importance heatmaps (right) of pre-trained ResNet50 FER model, calculated with LIME for a true positive, true negative, false positive, and false negative samples in the final mind wandering classification (top to bottom)
Latent Feature Explanation
In order to gain deeper insights into the employed latent features, we apply the explainability algorithm LIME (Ribeiro et al., 2016 ) on our feature extraction CNN model, pre-trained on facial expression recognition task, and fine-tuned to our mind wandering detection task. LIME is an Explainable AI tool that helps to understand the decision-making of an algorithm, as it allows highlighting areas of interest in the image, the so-called super-pixels, that contribute positively or negatively to the model’s prediction. This helps to get an intuition on why the model thinks this image belongs to a certain class and which part of a given image the decision is based on. It is important to take this step to uncover any unintended correlations that the classifier may have learned, such as those resulting from artifacts produced in the data collection process (Ribeiro et al., 2016 ).
In our study, these regions of interest let us draw conclusions about the information encoded in the latent features that are fed into the temporal mind wandering classifier. Therefore, it ensures the quality of feature extraction, by validating that the learned information aligns with established theoretical frameworks. Example images from the lab data including super-pixel boundaries and heatmaps, with dark blue encoding the most important areas, are provided in Fig. 7 . The super-pixel boundaries include the 5 most important features positively contributing to the obtained prediction. The heatmaps depict super-pixels by importance, by coloring the most important areas in dark blue. We see that the CNN part of our classification model, employed for feature extraction, mainly relies on information from the eye and mouth areas of the participants, which are consequently inherently encoded in our feature vectors as well. We included example images based on our mind wandering prediction results including a true positive, true negative, false positive, and a false negative. These results are in line with previous studies suggesting facial textures and AUs are meaningful features for video-based mind wandering detection (Bosch & D’Mello, 2021 ).
Since our analysis is based on video clips, the features shown are extracted from each frame individually and then transferred to the LSTM module as a time series. In this way, temporal dynamics can be displayed. To visualize this process, we have mapped the time course of the most important features for a correctly predicted mind wandering instance in Fig. 8 . Every 25th frame from a 10 s clip is depicted. It is evident that the focused regions undergo minimal changes based on facial expression and eye opening, but the primary focus on the eye region remains consistent.
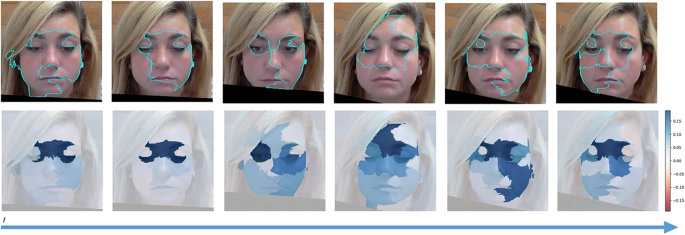
Temporal dynamics of extracted latent feature super pixels. Example depiction of every 25th frame from one correctly predicted mind wandering instance
In this section, we discuss our findings, also focusing on application scenarios and ethical issues.
Main Findings
We showed that features extracted from a pre-trained CNN on the AffectNet facial expression recognition dataset are informative for predicting mind wandering, even to a higher degree when they are utilized with temporal models that use frame-wise extracted features as input. The best results for within-dataset prediction were obtained using deep learning models such as DNN, LSTM, and BiLSTM, which allowed us to detect mind wandering \(\approx 14\%\) above the chance level. This indicates that the latent representations provided by a model based on the recognition of six basic facial expressions of emotion contain information at the same level for the task of mind wandering recognition as a set of explicit out-of-the-box features such as AUs, facial landmarks, and gaze vectors extracted by the OpenFace toolbox. Further, visualizations of areas of interest from the pre-trained FER model, fine-tuned on the mind wandering task showed that mainly eye and mouth areas were encoded by the model and, therefore, most likely encoded in the latent representation. these findings show that the features used represent meaningful information and can increase confidence in the latent representations. The results are comparable to those previously obtained by Bosch & D’Mello ( 2021 ) using a CART fusion of five explicit and hand-crafted feature groups on this data ( \(F_{1}\) = 0.478; \(\approx 25\%\) above chance level). By using transfer learning, the problem of insufficient data to train deep learning for this task can be overcome. Fine-tuning these features in an end-to-end model on the task at hand did slightly improve performance for the respective models but depends strongly on the available amount of data.
When we evaluated these models with a new dataset that differed in the task, setting, and target group, we showed that latent features generalized very well and achieved a prediction performance ( \(\approx 11.4\%\) above chance level) that was comparable to the best performance of a within-task prediction in the state-of-the-art (Lee et al., 2022 ). Also for the cross-dataset setting, the models on the latent features performed better overall than those on the OpenFace features. This indicates that the transfer-learned features generalize better than the explicit out-of-the-box features without manual feature engineering. This may be due to the fact that they are based purely on facial expression, which is less stimulus-specific than, for example, gaze. Beyond the only existing generalization study of video-based mind wandering recognition (Stewart et al., 2017b ), which was based on AUs, we were able to show that latent facial features are also predictive beyond the lab in naturalistic settings, such as the home. These features were trained on an in-the-wild dataset, which might favor the transferability of the features to an in-the-wild setting.
Nevertheless, the generalization results are remarkable because not only the setting and task differ in our cross-dataset prediction, but also the culture of the target group. As mentioned in “ Introduction ”, there is an ongoing debate about the universality of facial expressions of emotion (Russell, 1994 ; Ekman, 1994 ; Jack et al., 2012 ). One position taken is that the facial expressions of the six basic emotions relate to Western interpretations of these, but are not representative for instance, of East Asian culture (Jack et al., 2012 ). While often universality of facial expressions of the six basic emotions is assumed, several studies tackled cross-cultural FER (Dailey et al., 2010 ; Benitez-Garcia et al., 2017 ; Ali et al., 2020 ) and highlighted culture-specific differences in automated detection. The features used in this work are based on a model that was pre-trained on AffectNet data without accounting for culture-specific differences. Nevertheless, they generalize well from U.S. to Korean subjects in detecting mind wandering.
When comparing the prediction performances across gender, for both scenarios, we achieve better classification results for the female group. This difference could partly be the result of a combination of a greater proportion of women in both datasets, and the generally higher mind wandering rate for females in the training sample. However, gender differences are higher for temporal models with a higher number of parameters, in need of large training data. Another challenge is choosing the best prediction model. We demonstrated that adjusting the threshold for decision-making, depending on its application, can improve the effectiveness of these predictions, especially in cross-dataset prediction. However, focusing on F \(_1\) scores can lead to an imbalance between recall and precision of the mind wandering class, therefore we focus on the rank metric AUC-PR. Depending on the application scenario, the need for optimization towards one or the other can be advantageous. While it is important to capture all mind wandering instances when used for research and testing of materials, it may be more useful to have high precision when using interventions not to disturb learners in attentive moments.
Applications
The ability of a machine learning model to generalize is of primary importance in terms of its potential applications. On the one hand, it enables finding the optimal design of learning materials and systems in a way that less mind wandering occurs. On the other hand, automated detection of mind wandering has the potential to help learners in online learning contexts such as ITSs and MOOCs to focus their attention on the task at hand, which often consists of tasks such as the ones we explored in this study: reading or video lecture viewing. This can be done through interventions such as providing feedback, suggesting re-watching or re-reading, asking intermediate questions, or adapting the presented content when a user loses focus. Such interventions can reduce user’s mind wandering (D’Mello et al., 2017 ; Hutt et al., 2021 ; Mills et al., 2021 ) and therefore, based on the observed negative relationship between mind wandering episodes and learning success (Smallwood, 2011 ; Feng et al., 2013 ; Szpunar et al., 2013 ; Hollis & Was, 2016 ), improve learning outcomes. However, since all of these studies examine short-term effects, there is a need to validate the long-term impact of mind wandering interventions.
The automatic detection of learners’ off-task thoughts presented here can be used in future research to provide learners with different types of interventions and feedback. An experimental examination with corresponding questionnaires and knowledge tests could examine whether these are suitable to help learners sustain their attention and whether they actually lead to improved learning success without being distracting or disruptive. While the efficacy of some interventions based on eye tracking has been investigated in both lab and classroom context (D’Mello et al., 2017 ; Hutt et al., 2021 ; Mills et al., 2021 ), a generalizable video-based recognition approach proposed in this study will allow future research to investigate the effectiveness of interventions outside the laboratory in naturalistic settings on a large scale. Furthermore, it will allow to deploy mind wandering detection modules in interfaces used in settings, in which eye tracking is not feasible.
While the current precision in predicting isolated occurrences of mind wandering might evoke uncertainties, our research shows that by consolidating predictions at the participant level correlations with the ground truth, ranging from moderate to high, can be achieved. This underscores that when aggregated over longer periods of time, analogous to the temporal scope utilized in interventions, models can yield improved outcomes. However, given the current performance of state-of-the-art video-based mind wandering prediction and the potential for false positive predictions, it is imperative to consider interventions that do not impede the learning process. Among the viable options, two seem most suitable: Non-interruptive interventions, like follow-up prompts to re-read or re-watch critical parts of learning materials, prioritize fostering self-regulated learning while minimizing disruptions and distractions. By seamlessly integrating these interventions into the learning environment, a more favorable and effective learning experience can be achieved. Furthermore, the implementation of thresholds, based on criteria such as prediction confidence or time durations, ensures the maintenance of intervention quality. This approach mitigates the risk of learner irritation or negative experiences resulting from inadequately controlled interventions. In doing so, even if video-based mind wandering detection is less performant than comparable tasks, such as emotion recognition, its use in online learning systems can still lead to an improvement in attention. It is crucial to determine the required level of precision for targeted interventions to attain their desired effect without impeding learning. In general, the goal should be to support learners in self-regulated learning (Järvelä et al., 2023 ) rather than to monitor or assess them. Therefore, it is important to consider user and data privacy in any potential application.
Ethical Considerations
While the application of video-based mind wandering detection holds promise for supporting learning in various applications, it also raises concerns regarding privacy, fairness, and inclusiveness. Since videos capture the identifiable faces of students during learning tasks, responsible handling of this data becomes imperative. While we work with raw videos, for model development, one of the advantages of our approaches is that for real world applications both explicit and latent features can be extracted on the fly in real time without the need of saving videos in the first place. A similar approach was employed in the classroom study of Bosch & D’Mello ( 2021 ) to preserve privacy. This aspect is very important as particularly vulnerable target groups such as school children (i.e., minors in dependent relationships), as those can also benefit from MOOCs and ITSs that utilize mind wandering detection. The HCI community has been addressing data privacy-related issues in different tasks such as face recognition (Erkin et al., 2009 ), gaze estimation (Bozkir et al., 2020 ), or even in the classroom context (Sümer et al., 2020 ) and similar approaches that preserve privacy should be utilized if one uses raw videos in an end-to-end fashion when mind wandering detection modules are deployed in real-world systems. Another convenient approach would also include utilizing federated learning systems (Li et al., 2020 ) where the training of machine learning models is carried out locally in user devices by keeping the sensitive data away from other parties and only sending the trained models to the computation party for aggregation. In brief, while the performance of mind wandering detection is an important aspect, privacy issues are of paramount importance for real-world use. In any case the process of collecting and analyzing any form of data should be transparent to the user with informed consent and clarity of the purposes the data will be used for (Nguyen et al., 2022b ). Another important aspect of deploying automated mind wandering detection in educational settings is the inclusiveness in data and algorithms (Nguyen et al., 2022b ). To ensure fairness and equality across user groups, it is crucial that these algorithms are trained and tested on diverse, unbiased data. This research underscores the significance of these considerations by aiming to develop a culturally generalizable algorithm and conduct a transparent analysis of gender fairness. While the results are promising with regard to generalization, especially the found gender differences stemming from unbalanced data, highlight the necessity for diverse and representative data that facilitate even better adherence to these principles in future research.
Limitations and Future Work
Despite its novelty, the presented work exhibits several limitations. First of all, we examined cross-setting predictions from the lab dataset to the in-the-wild dataset. This is partly due to the fact that lab-based data collection is more controlled, and therefore, allows for higher data quality. As reported in the original study (Lee et al., 2022 ) of the in-the-wild dataset, a large amount of data had to be excluded during data preparation due to insufficient data quality, for example, due to low luminance. From a practical standpoint, the generalizability from labeled lab data to in-the-wild data is a likely setting for future applications. Related to this point, the present in-the-wild dataset is rather small to train our proposed models on it. The need for a comparatively large labeled dataset to enable us to use the proposed temporal methods is a further limitation of this work. Currently, to our best knowledge, no larger in-the-wild mind wandering dataset is available. Despite the limited size of the in-the-wild dataset, this study examines the potential generalizability of state-of-the art mind wandering detection to diverse datasets. However, it is important to exercise caution when interpreting these findings due to the dataset’s size. Future research should focus on collecting larger datasets to provide a more robust evaluation of the model’s performance and enhance our understanding of its broader applicability. In general, further advances in automated detection of mind wandering should be evaluated based on their generalizability to in-the-wild settings to avoid the optimization of performance on single datasets at the expense of generalizability, as was recently discussed in the related field of affect detection (D’Mello & Booth, 2023 ).
While our cross-dataset prediction examines the models’ ability to generalize two culturally different target groups, the sample of university and college students is somewhat homogeneous regarding other demographics, such as age. Future research should investigate, how well the model can be transferred to other target groups, for example, to school children. Furthermore, differences in predictive power by gender were revealed. A balanced sample by gender, as well as further investigation of gender differences in mind wandering, should be an important consideration for future studies to avoid bias.
In general, the presented results underscore the complexity of the recognition of mind wandering solely through facial video analysis. The approach presented in this paper, alongside previous approaches, can predict off-task thoughts above chance level, for dataset-specific class distributions, albeit without achieving exceptionally high prediction performances. While the achieved prediction accuracy may be considered moderate, and will potentially lead to false positive predictions when employed in educational systems, it is crucial to acknowledge the inherent difficulties associated with accurately identifying such a nuanced cognitive process. A prior study hinting at upper bounds for appearance-based detection showed that human observers only could identify mind wandering episodes to a similar extent ( \(F_{1}\) = 0.406) as machine-learning based detection algorithms on the lab data (Bosch & D’Mello, 2022 ). These results strengthen the assumption that there is a limit to the accuracy with which the covert cognitive state of mind wandering can be detected based only on appearance. However, the required detection accuracy heavily depends on the desired application. Initial studies exploring interventions, like reiterating content, and asking questions, based on similar prediction performances show the potential success of those (D’Mello et al., 2017 ; Mills et al., 2021 ; Hutt et al., 2021 ). Future investigations should deploy the presented automated mind wandering detection approach to deliver interventions while assessing learning outcomes to determine the extent to which interventions improve attention and learning outcomes, whether frequent or incorrectly delivered mind wandering interventions hinder or interfere with learning and whether predictions at the current precision level are sufficient to be integrated into systems. As mentioned above, especially the use of non-intrusive interventions and the implementation of quality thresholds should be considered in the context of moderate prediction accuracies.
Additionally, the limited performance of these elaborate models can be attributed to the size constraints of the employed training dataset. Unfortunately, to our best knowledge, no other video-based mind wandering detection dataset is publicly available at this moment. To further advance the field and improve prediction outcomes, future research should place emphasis on collecting large-scale datasets, particularly in in-the-wild scenarios. By incorporating such diverse and extensive data, researchers can enhance the models’ performance and pave the way for more accurate and robust mind wandering detection systems. A commendable approach was employed by Hutt et al. ( 2023 ), who gathered webcam eye tracking data both in university environments and via the platform Prolific, thereby ensuring the inclusion of diverse target groups and settings. Further potential strategies for enhancing prediction performance may include the implementation of personalization, such as the use of personalized prediction thresholds. This approach could partially compensate for the presumed interpersonal variations. Another way to improve prediction accuracy is to add other signals, like behavioural trace data or physiological signals. In this work, we focused on webcam videos due to the goal of scalability and low-threshold application in naturalistic settings. One potential for future research in this realm could be the further improvement of webcam-based eye tracking, since eye movements have been shown to be highly predictive for mind wandering (Hutt et al., 2023 ; Bixler & D’Mello, 2014 ; D’Mello et al., 2016 ; Faber et al., 2018 ; Mills et al., 2021 ).
In this work, we proposed a novel and generalizable approach for mind wandering detection utilizing facial features based on transfer learning from videos. Our results show the meaningfulness and transferability of those features with within- and cross-dataset prediction tasks on two challenging datasets. In particular, the results of the cross-dataset setting that differed with regard to the task, target group, and environment show the generalizability of our approach, which is key to the deployment of such models in intelligent learning systems to support learners to keep their attention on a given task.
Data Availability
The Lab data by Bosch & D’Mello Baldi et al. ( 1999 ) is not publicly available. The In-the-wild data by Lee et al. Lee et al. ( 2022 ) is available for research purposes by contacting the authors at https://nmsl.kaist.ac.kr/projects/attention/ .
Ali, G., Ali, A., Ali, F., Draz, U., Majeed, F., Yasin, S., & Haider, N. (2020). Artificial neural network based ensemble approach for multicultural facial expressions analysis. IEEE Access, 8 , 134950–134963. https://doi.org/10.1109/ACCESS.2020.3009908
Article Google Scholar
Baldi, P., Brunak, S., Frasconi, P., Soda, G., & Pollastri, G. (1999). Exploiting the past and the future in protein secondary structure prediction. Bioinformatics, 15 (11), 937–946.
Baltrušaitis, T., Robinson, P., Morency, L.-P. (2016). Openface: an open source facial behavior analysis toolkit. 2016 ieee winter conference on applications of computer vision (wacv) (p.1-10).
Benitez-Garcia, G., Nakamura, T., Kaneko, M. (2017). Analysis of in- and out-group differences between western and east-asian facial expression recognition. 2017 fifteenth iapr international conference on machine vision applications (mva) (p.402-405).
Bixler, R., & D’Mello, S.K. (2014). Toward fully automated person-independent detection of mind wandering. International conference on user modeling, adaptation, and personalization (pp. 37–48).
Bixler, R., & D’Mello, S.K. (2021). Crossed eyes: Domain adaptation for gaze-based mind wandering models. Acm symposium on eye tracking research and applications (pp. 1–12).
Blanchard, N., Bixler, R., Joyce, T., D’Mello, S.K. (2014). Automated physiological-based detection of mind wandering during learning. Ş. Trǎuşan-Matu, K.E. Boyer, M. Crosby, and K. Panourgia (Eds.), Intelligent tutoring systems (pp. 55–60). Cham: Springer.
Bonifacci, P., Viroli, C., Vassura, C., Colombini, E., Desideri, L. (2022). The relationship between mind wandering and reading comprehension: A meta-analysis. Psychonomic Bulletin & Review , 1–20,
Bosch, N., & D’Mello, S. K. (2021). Automatic detection of mind wandering from video in the lab and in the classroom. IEEE Transactions on Affective Computing, 12 (4), 974–988. https://doi.org/10.1109/TAFFC.2019.2908837
Bosch, N., & D’Mello, S. K. (2022). Can computers outperform humans in detecting user zone-outs? implications for intelligent interfaces. ACM Transactions on Computer-Human Interaction, 29 (2), 1–33.
Bozkir, E., Ünal, A. B., Akgün, M., Kasneci, E., & Pfeifer, N. (2020). Privacy preserving gaze estimation using synthetic images via a randomized encoding based framework., 21 (1–21), 5. https://doi.org/10.1145/3379156.3391364
Brishtel, I., Khan, A. A., Schmidt, T., Dingler, T., Ishimaru, S., & Dengel, A. (2020). Mind wandering in a multimodal reading setting: Behavior analysis & automatic detection using eye-tracking and an eda sensor. Sensors (Basel, Switzerland), 20 (9), 2546. https://doi.org/10.3390/s20092546
Caruso, M., & D’Mello, S. (2023). Do associations between mind wandering and learning from complex texts vary by assessment depth and time? Lak23: 13th international learning analytics and knowledge conference (pp. 230–239).
Chawla, N. V., Bowyer, K. W., Hall, L. O., & Kegelmeyer, W. P. (2002). SMOTE: Synthetic minority over-sampling technique. Journal of Artificial Intelligence Research, 16 , 321–357. https://doi.org/10.1613/jair.953
Chen, T., & Guestrin, C. (2016). XGBoost: A scalable tree boosting system. Proceedings of the 22nd acm sigkdd international conference on knowledge discovery and data mining (pp. 785–794). New York, NY, USA: ACM.
Christoff, K., Gordon, A. M., Smallwood, J., Smith, R., & Schooler, J. W. (2009). Experience sampling during fmri reveals default network and executive system contributions to mind wandering. Proceedings of the National Academy of Sciences, 106 (21), 8719–8724. https://doi.org/10.1073/pnas.0900234106
Conrad, C., & Newman, A. (2021). Measuring mind wandering during online lectures assessed with eeg. Frontiers in Human Neuroscience, 15 , 697532.
Cortes, C., & Vapnik, V. (1995). Support-vector networks. Machine Learning, 20 (3), 273–297. https://doi.org/10.1007/BF00994018
Dailey, M. N., Joyce, C., Lyons, M. J., Kamachi, M., Ishi, H., Gyoba, J., & Cottrell, G. W. (2010). Evidence and a computational explanation of cultural differences in facial expression recognition. Emotion, 10 (6), 874.
Deng, J., Guo, J., Ververas, E., Kotsia, I., Zafeiriou, S. (2020). Retinaface: Single-shot multi-level face localisation in the wild. Proceedings of the ieee/cvf conference on computer vision and pattern recognition (pp. 5203–5212).
Dhawan, S. (2020). Online learning: A panacea in the time of covid-19 crisis. Journal of educational technology systems, 49 (1), 5–22.
Dhindsa, K., Acai, A., Wagner, N., Bosynak, D., Kelly, S., Bhandari, M., & Sonnadara, R. R. (2019). Individualized pattern recognition for detecting mind wandering from eeg during live lectures. PloS one, 14 (9), e0222276.
D’Mello, S. K., & Booth, B. M. (2023). Affect detection from wearables in the real wild: Fact, fantasy, or somewhere in between? IEEE Intelligent Systems, 38 (1), 76–84.
D’Mello, S.K., Kopp, K., Bixler, R.E., Bosch, N. (2016). Attending to attention: Detecting and combating mind wandering during computerized reading. Proceedings of the 2016 chi conference extended abstracts on human factors in computing systems (pp. 1661–1669).
D’Mello, S.K., Mills, C., Bixler, R., Bosch, N. (2017). Zone out no more: Mitigating mind wandering during computerized reading. International Educational Data Mining Society .
D’Mello, S. K., & Mills, C. S. (2021). Mind wandering during reading: An interdisciplinary and integrative review of psychological, computing, and intervention research and theory. Language and Linguistics Compass, 15 (4), e12412.
Dong, H. W., Mills, C., Knight, R. T., & Kam, J. W. (2021). Detection of mind wandering using eeg: Within and across individuals. Plos one, 16 (5), e0251490.
Ekman, P. (1994). Strong evidence for universals in facial expressions: a reply to russell’s mistaken critique. Psychological Bulletin, 115 , 268–287.
Erkin, Z., Franz, M., Guajardo, J., Katzenbeisser, S., Lagendijk, I., Toft, T. (2009). Privacy-preserving face recognition. Privacy enhancing technologies: 9th international symposium, pets 2009 (pp. 235–253).
Faber, M., Bixler, R., & D’Mello, S. K. (2018). An automated behavioral measure of mind wandering during computerized reading. Behavior Research Methods, 50 (1), 134–150. https://doi.org/10.3758/s13428-017-0857-y
Faber, M., & D’Mello, S. K. (2018). How the stimulus influences mind wandering in semantically rich task contexts. Cognitive Research: Principles and Implications, 3 (1), 35. https://doi.org/10.1186/s41235-018-0129-0
Faber, M., Krasich, K., Bixler, R. E., Brockmole, J. R., & D’Mello, S. K. (2020). The eye-mind wandering link: Identifying gaze indices of mind wandering across tasks. Journal of Experimental Psychology: Human Perception and Performance, 46 (10), 1201–1221. https://doi.org/10.1037/xhp0000743
Feng, S., D’Mello, S. K., & Graesser, A. C. (2013). Mind wandering while reading easy and difficult texts. Psychonomic Bulletin & Review, 20 (3), 586–592. https://doi.org/10.3758/s13423-012-0367-y
Franklin, M. S., Broadway, J. M., Mrazek, M. D., Smallwood, J., & Schooler, J. W. (2013). Window to the wandering mind: Pupillometry of spontaneous thought while reading. Quarterly Journal of Experimental Psychology, 66 (12), 2289–2294. https://doi.org/10.1080/17470218.2013.858170 . (PMID: 24313285).
He, K., Zhang, X., Ren, S., Sun, J. (2016). Deep residual learning for image recognition. Proceedings of the ieee conference on computer vision and pattern recognition (cvpr) (pp. 770–778). IEEE.
Hochreiter, S., & Schmidhuber, J. (1997). Long short-term memory. Neural computation, 9 (8), 1735–1780.
Hollis, R. B., & Was, C. A. (2016). Mind wandering, control failures, and social media distractions in online learning. Learning and Instruction, 42 , 104–112. https://doi.org/10.1016/j.learninstruc.2016.01.007
Hutt, S., Hardey, J., Bixler, R., Stewart, A., Risko, E., D’Mello, S.K. (2017). Gaze-based detection of mind wandering during lecture viewing. International Educational Data Mining Society .
Hutt, S., Krasich, K., Mills, C., Bosch, N., White, S., Brockmole, J. R., & D’Mello, S. K. (2019). Automated gaze-based mind wandering detection during computerized learning in classrooms. User Modeling and User-Adapted Interaction, 29 (4), 821–867. https://doi.org/10.1007/s11257-019-09228-5
Hutt, S., Krasich, K., R. Brockmole, J., K. D’Mello, S.K. (2021). Breaking out of the lab: Mitigating mind wandering with gaze-based attention-aware technology in classrooms. Proceedings of the 2021 chi conference on human factors in computing systems (pp. 1–14).
Hutt, S., Wong, A., Papoutsaki, A., Baker, R.S., Gold, J.I., Mills, C. (2023). Webcam-based eye tracking to detect mind wandering and comprehension errors. Behavior Research Methods , 1–17,
Jack, R. E., Garrod, O. G., Yu, H., Caldara, R., & Schyns, P. G. (2012). Facial expressions of emotion are not culturally universal. Proceedings of the National Academy of Sciences, 109 (19), 7241–7244.
Jang, D., Yang, I., & Kim, S. (2020). Detecting mind-wandering from eye movement and oculomotor data during learning video lecture. Education Sciences, 10 (3), 51. https://doi.org/10.3390/educsci10030051
Järvelä, S., Molenaar, I., & Nguyen, A. (2023). Advancing srl research with artificial intelligence . Elsevier.
Book Google Scholar
Jeni, L.A., Cohn, J.F., De La Torre, F. (2013). Facing imbalanced data–recommendations for the use of performance metrics. 2013 humaine association conference on affective computing and intelligent interaction (p.245-251).
Jin, C. Y., Borst, J. P., & Van Vugt, M. K. (2019). Predicting task-general mind-wandering with eeg. Cognitive, Affective, & Behavioral Neuroscience, 19 (4), 1059–1073.
Just, M. A., & Carpenter, P. A. (1976). Eye fixations and cognitive processes. Cognitive psychology, 8 (4), 441–480.
Kuvar, V., Kam, J.W., Hutt, S., Mills, C. (2023). Detecting when the mind wanders off task in real-time: An overview and systematic review. Proceedings of the 25th international conference on multimodal interaction (pp. 163–173).
Lee, T., Kim, D., Park, S., Kim, D., Lee, S- J. (2022). Predicting mind-wandering with facial videos in online lectures. Proceedings of the ieee/cvf conference on computer vision and pattern recognition (cvpr) workshops (pp. 2104–2113).
Lemay, D. J., Bazelais, P., & Doleck, T. (2021). Transition to online learning during the covid-19 pandemic. Computers in Human Behavior Reports, 4 , 100130.
Levine, M.D. (1990). Keeping a head in school. Educators Publishing Service, Inc., 75 Moulton St., Cambridge, MA 02138-1104.
Li, S., & Deng, W. (2020). Deep facial expression recognition: A survey. IEEE Transactions on Affective Computing, 1–1 ,. https://doi.org/10.1109/TAFFC.2020.2981446
Li, T., Sahu, A. K., Talwalkar, A., & Smith, V. (2020). Federated learning: Challenges, methods, and future directions. IEEE Signal Processing Magazine, 37 (3), 50–60. https://doi.org/10.1109/MSP.2020.2975749
Mills, C., Gregg, J., Bixler, R., & D’Mello, S. K. (2021). Eye-mind reader: An intelligent reading interface that promotes long-term comprehension by detecting and responding to mind wandering. Human-Computer Interaction, 36 (4), 306–332.
Mollahosseini, A., Hasani, B., & Mahoor, M. H. (2017). Affectnet: A database for facial expression, valence, and arousal computing in the wild. IEEE Transactions on Affective Computing, 10 (1), 18–31.
Ngo, D., Nguyen, A., Dang, B., Ngo, H. (2024). Facial expression recognition for examining emotional regulation in synchronous online collaborative learning. International Journal of Artificial Intelligence in Education , 1–20,
Nguyen, A., Hong, Y., Dang, B., Nguyen, P.T.B. (2022). Emotional regulation in synchronous online collaborative learning: A facial expression recognition study. ICIS 2022 Proceedings.
Nguyen, A., Ngo, H.N., Hong, Y., Dang, B., Nguyen, B- P.T. (2022). Ethical principles for artificial intelligence in education. Education and Information Technologies , 1–21,
Pan, S. C., Sana, F., Schmitt, A. G., & Bjork, E. L. (2020). Pretesting reduces mind wandering and enhances learning during online lectures. Journal of Applied Research in Memory and Cognition, 9 (4), 542–554.
Pham, P., & Wang, J. (2015). Attentivelearner: Improving mobile mooc learning via implicit heart rate tracking. C. Conati, N. Heffernan, A. Mitrovic, and M.F. Verdejo (Eds.), Artificial intelligence in education (pp. 367–376). Cham: Springer.
Randall, J. G., Oswald, F. L., & Beier, M. E. (2014). Mind-wandering, cognition, and performance: a theory-driven meta-analysis of attention regulation. Psychological bulletin, 140 (6), 1411.
Rawat, W., & Wang, Z. (2017). Deep convolutional neural networks for image classification: A comprehensive review. Neural computation, 29 (9), 2352–2449.
Article MathSciNet Google Scholar
Rayner, K. (1998). Eye movements in reading and information processing: 20 years of research. Psychological bulletin, 124 (3), 372.
Reichle, E. D., Pollatsek, A., & Rayner, K. (2012). Using ez reader to simulate eye movements in nonreading tasks: A unified framework for understanding the eye-mind link. Psychological review, 119 (1), 155.
Ribeiro, M.T., Singh, S., Guestrin, C. (2016). "why should I trust you?": Explaining the predictions of any classifier. Proceedings of the 22nd ACM SIGKDD international conference on knowledge discovery and data mining, san francisco, ca, usa, august 13-17, 2016 (pp. 1135–1144).
Risko, E. F., Anderson, N., Sarwal, A., Engelhardt, M., & Kingstone, A. (2012). Everyday attention: Variation in mind wandering and memory in a lecture. Applied Cognitive Psychology, 26 (2), 234–242. https://doi.org/10.1002/acp.1814
Russell, J. A. (1994). Is there universal recognition of emotion from facial expression? a review of the cross-cultural studies. Psychological bulletin, 115 (1), 102.
Schooler, J. W., Smallwood, J., Christoff, K., Handy, T. C., Reichle, E. D., & Sayette, M. A. (2011). Meta-awareness, perceptual decoupling and the wandering mind. Trends in Cognitive Sciences, 15 (7), 319–326. https://doi.org/10.1016/j.tics.2011.05.006
Schubert, A- L., Frischkorn, G.T., Rummel, J. (2020). The validity of the online thought-probing procedure of mind wandering is not threatened by variations of probe rate and probe framing. Psychological research, 84 (7), 1846–1856.
Schuster, M., & Paliwal, K. K. (1997). Bidirectional recurrent neural networks. IEEE transactions on Signal Processing, 45 (11), 2673–2681.
Smallwood, J. (2011). Mind-wandering while reading: Attentional decoupling, mindless reading and the cascade model of inattention. Language and Linguistics Compass, 5 (2), 63–77. https://doi.org/10.1111/j.1749-818X.2010.00263.x
Smallwood, J., McSpadden, M., & Schooler, J. W. (2007). The lights are on but no one’s home: meta-awareness and the decoupling of attention when the mind wanders. Psychonomic Bulletin & Review, 14 (3), 527–533. https://doi.org/10.3758/BF03194102
Smallwood, J., & Schooler, J. W. (2006). The restless mind. Psychological Bulletin, 132 (6), 946–958.
Smallwood, J., & Schooler, J. W. (2015). The science of mind wandering: empirically navigating the stream of consciousness. Annual review of psychology, 66 , 487–518. https://doi.org/10.1146/annurev-psych-010814-015331
Smilek, D., Carriere, J. S., & Cheyne, J. A. (2010). Out of mind, out of sight: Eye blinking as indicator and embodiment of mind wandering. Psychological science, 21 (6), 786–789.
Stewart, A., Bosch, N., Chen, H., Donnelly, P., D’Mello, S.K. (2017). Face forward: Detecting mind wandering from video during narrative film comprehension. International conference on artificial intelligence in education (pp. 359–370).
Stewart, A., Bosch, N., D’Mello, S.K. (2017). Generalizability of face-based mind wandering detection across task contexts. International Educational Data Mining Society .
Sümer, Ö., Gerjets, P., Trautwein, U., Kasneci, E. (2020). Automated anonymisation of visual and audio data in classroom studies. The workshops of the thirty-fourth aaai conference on artificial intelligence.
Sümer, Ö., Goldberg, P., D’Mello, S.K., Gerjets, P., Trautwein, U., Kasneci, E. (2021). Multimodal engagement analysis from facial videos in the classroom. IEEE Transactions on Affective Computing .
Szpunar, K. K., Moulton, S. T., & Schacter, D. L. (2013). Mind wandering and education: from the classroom to online learning. Frontiers in Psychology, 4 , 495. https://doi.org/10.3389/fpsyg.2013.00495
Varao-Sousa, T. L., & Kingstone, A. (2019). Are mind wandering rates an artifact of the probe-caught method? using self-caught mind wandering in the classroom to test, and reject, this possibility. Behavior Research Methods, 51 (1), 235–242. https://doi.org/10.3758/s13428-018-1073-0
Wong, A. Y., Smith, S. L., McGrath, C. A., Flynn, L. E., & Mills, C. (2022). Task-unrelated thought during educational activities: A meta-analysis of its occurrence and relationship with learning. Contemporary Educational Psychology, 71 , 102098.
Zhang, H., Miller, K. F., Sun, X., & Cortina, K. S. (2020). Wandering eyes: Eye movements during mind wandering in video lectures. Applied Cognitive Psychology, 34 (2), 449–464. https://doi.org/10.1002/acp.3632
Zhao, Y., Lofi, C., Hauff, C. (2017). Scalable mind-wandering detection for moocs: A webcam-based approach. É. Lavoué, H. Drachsler, K. Verbert, J. Broisin, and M. Pérez-Sanagustín (Eds.), Data driven approaches in digital education (pp. 330–344). Cham: Springer International Publishing.
Download references
Open Access funding enabled and organized by Projekt DEAL. Babette Bühler is a doctoral candidate and supported by the LEAD Graduate School and Research Network, which is funded by the Ministry of Science, Research and the Arts of the state of Baden-Württemberg within the framework of the sustainability funding for the projects of the Excellence Initiative II. This research was partly funded by the Deutsche Forschungsgemeinschaft (DFG, German Research Foundation) under Germany’s Excellence Strategy - EXC number 2064/1 - Project number 390727645. Sidney D’Mello was supported by the National Science Foundation (DRL 1920510). The opinions expressed are those of the authors and do not represent the views of the funding agencies.
Author information
Authors and affiliations.
Hector Research Institute of Education Sciences and Psychology, University of Tübingen, Europastraße 6, Tübingen, 72072, Germany
Babette Bühler, Patricia Goldberg & Ulrich Trautwein
Human-Computer Interaction, University of Tübingen, Sand 14, Tübingen, 72076, Germany
University of Augsburg, Universitätsstraße 6a, Augsburg, 86159, Germany
Institute of Cognitive Science and the Department of Computer Science, University of Colorado, UCB 344/ 1905 Colorado Ave., Boulder, 80309, Colorado, United States
Sidney D’Mello
Leibniz-Institut für Wissensmedien, Schleichstraße 6, Tübingen, 72076, Germany
Peter Gerjets
Human-Centered Technologies for Learning, Technical University Munich, Arcisstraße 21, Munich, 80333, Germany
Efe Bozkir & Enkelejda Kasneci
You can also search for this author in PubMed Google Scholar
Contributions
All authors contributed to the study conception and design. Data analysis was performed by Babette Bühler. The first draft of the manuscript was written by Babette Bühler, Efe Bozkir and all authors commented on previous versions of the manuscript. All authors read and approved the final manuscript.
Corresponding author
Correspondence to Babette Bühler .
Ethics declarations
Competing interests.
The authors have no relevant financial or non-financial interests to disclose.
Additional information
Publisher's note.
Springer Nature remains neutral with regard to jurisdictional claims in published maps and institutional affiliations.
Appendix A: Additional Tables
Rights and permissions.
Open Access This article is licensed under a Creative Commons Attribution 4.0 International License, which permits use, sharing, adaptation, distribution and reproduction in any medium or format, as long as you give appropriate credit to the original author(s) and the source, provide a link to the Creative Commons licence, and indicate if changes were made. The images or other third party material in this article are included in the article’s Creative Commons licence, unless indicated otherwise in a credit line to the material. If material is not included in the article’s Creative Commons licence and your intended use is not permitted by statutory regulation or exceeds the permitted use, you will need to obtain permission directly from the copyright holder. To view a copy of this licence, visit http://creativecommons.org/licenses/by/4.0/ .
Reprints and permissions
About this article
Bühler, B., Bozkir, E., Goldberg, P. et al. From the Lab to the Wild: Examining Generalizability of Video-based Mind Wandering Detection. Int J Artif Intell Educ (2024). https://doi.org/10.1007/s40593-024-00412-2
Download citation
Accepted : 14 May 2024
Published : 17 June 2024
DOI : https://doi.org/10.1007/s40593-024-00412-2
Share this article
Anyone you share the following link with will be able to read this content:
Sorry, a shareable link is not currently available for this article.
Provided by the Springer Nature SharedIt content-sharing initiative
- Mind wandering
- Transfer-learning
- Affective computing
- Educational technology
- Find a journal
- Publish with us
- Track your research
Five mind-blowing facts - New York Central passenger trains
Mind-blowing facts - new york central passenger trains.
We are 50-plus years into the Amtrak era, which began on May 1, 1971. A few Amtrak trains still carry the identity of the conveyances they imitate - California Zephyr, Empire Builder, and Crescent - to mention a few. What lives on today is a contemporary train - traveling a route similar to the original, but not quite - that is a shadow of their forebears. When it comes to American passenger trains, names like the Super Chief, City of New Orleans, or Capitol Limited evoke a hushed reverence and the legend of exceptional service, comfortable accommodations, fine food, and a schedule well maintained.
If these few name trains are the stuff of legend, then the New York Central, its Great Steel Fleet, and trains like the 20th Century Limited are the stuff legends are made of. Many have argued that the 20th Century Limited was the finest passenger train on American rails. Argue how you like and long for the days of yore, but either way come along now for a glimpse behind the legendary standard passenger trains of the New York Central. There is more to the story than meets the eye across all the years. These are five-mind blowing facts about the passenger trains of the New York Central.
No. 1 - The Century by the numbers
The New York Central's top passenger train was the 20th Century Limited . It was an all-Pullman, extra-fare, luxury liner running between New York and Chicago on a 15-hour schedule in 1948. An early-1930 advertisement by the NYC stated that: "The 20th Century Limited long since ceased to be a ‘train.' It is a daily fleet of trains." In the 12 months prior to the ad's appearance, the NYC had dispatched 2,153 trains carrying the 20th Century Limited name. That works out to almost six sections of the train daily. The record day came in January 1929 when seven Century sections traveled eastbound from Chicago to New York carrying 822 passenger.
Each Century section required a crew of nearly 70, which is a ratio of one crew member for every 1.75 passengers. Included among the staff were sleeping car porters, dining car waiters, stewards, cooks, maids, barbers, manicurists, valets, and secretaries.
Equipment-wise, the NYC kept 24 locomotives and 122 identical luxury sleeping cars on standby to meet demand and or mitigate negative situations impacting the Century's schedule. The schedule, by the way, was guaranteed to the point that the NYC refunded each passenger $1 for each hour the train was late. Also, the 122 standby sleepers, as with all cars assigned to the Century, had to be turned so that passenger room windows faced the waters of the Hudson River going into or coming out of New York. The NYC paid attention to the smallest detail so passengers would have the best travel experience.
No. 2 - The Wolverine and My Old School
Grab those stereophonic headphones, it's time for a trip back to 1973 …
I remember the 35 sweet goodbyes
When you put me on The Wolverine up to Annandale
It was still September
When your daddy was quite surprised
To find you with the working girls in the county jail
I was smoking with the boys upstairs when I
Heard about the whole affair, I said oh no
William and Mary won’t do …
Dial the volume down from 10 and let's interpret what you just heard. This is the first verse of the song My Old School from the group Steely Dan's 1973 album Countdown to Ecstasy. Band leaders Donald Fagen and Walter Becker tell the story of getting caught up in a pot bust at their old school - Bard College - in Annandale-on-Hudson, N.Y. The incident happened in May 1969, a time when "long hairs," like Fagen and Becker, were targeted for their behavior - real or imagined.
The Wolverine was a New York Central train running from New York to Chicago, and was popular transportation for Bard students. The train ran an interesting route, ducking into Canada after Buffalo, N.Y., and returning to the U.S. at Detroit, before a final sprint to the Windy City. It carried both sleeper and coach accommodations.
The time visited in My Old School was a time when The Wolverine, like many passenger trains, was in decline. Starting in 1957, the train lost its observation car. By December 1967, The Wolverine was no longer a named train, merely appearing as Nos. 17/8. During the Penn Central period, the train remained numbered only - westbound, Nos. 61/17. Eastbound, No. 14, was truncated at Buffalo, with passengers needing to change trains at 2:30 a.m. in order to reach New York.
Interestingly in all of this, The Wolverine never stopped in Annandale-on-Hudson. The closest NYC station to Bard College was 8 miles away in Rhinecliff, N.Y. Finally, today's Amtrak Wolverine, running between Chicago and Pontiac, Mich., via Detroit, is a direct descendant of the original NYC train … although neither of them would actually get you to my old school.
No. 3 - Would you like butter with that?
The 20th Century Limited epitomized the concept of a passenger train being akin to the finest hotel or steamship, but one that glides along steel rails rather than remaining perched on a foundation or cresting the next wave. During the Century's heyday, its two arch-competitors could be found in the Pennsylvania Railroad's Broadway Limited, and the Baltimore & Ohio Railroad's Capitol Limited , both of which also ran a New York-Chicago route. It has been reported, that all else being equal in the mind of the passenger, the choice of which train to take sometimes came down to the dining car the passenger preferred.
If a passenger liked butter, the 20th Century Limited was the way to go. Beginning with the Century's inauguration in 1902, Dr. William Seward Webb, a Vanderbilt relation and president of the Lake Shore & Michigan Southern Railroad over whose tracks the Century ran west of Buffalo, N.Y., became the official source for the train's butter provision. Webb owned a hobby agricultural venture - Shelburne Farm, located on the shores of Lake Champlain in Vermont. For a hobby farm, the operation was stunning, featuring well-maintained, prized herd of purebred Hereford cattle. From here, Webb produced the luscious creamy butter served aboard the Century.
For each passenger, the Century carried one pound of butter. The butter was served at table in the dining car and also used in the kitchen for cooking. However, whether traveling New York to Chicago or Chicago to New York, a pound of butter was allotted for each passenger. " … the diners of the Century may will have rolled on butter in their journal boxes," commented noted author and photographer Lucius Beebe in his book on the train.
No. 4 – Beer and NYC trains don't mix
New York's Grand Central Terminal stands as an icon in the railroad world. Since opening on Feb. 2, 1913, millions have passed through its spectacular halls, beginning, or ending a long-distance journey or merely as part of a daily commute. The Terminal's construction solved a significant neighborhood problem, by putting the trains underground and converting Park Avenue, running north of the station, into a boulevard befitting the posh uptown neighborhood the area was becoming.
Constructing Grand Central's two levels of track required massive excavations along Park Avenue. From 42nd to 50th street 1.6 million yards of rock were drilled and removed along with 1.2 million cubic yards of earth. More than 200 buildings were razed as the terminal site expanded from 23 to 48 acres.
One of the many construction challenges that arose appeared in the two-block area bounded by Lexington and Park avenues and 50th and 52nd streets. Roughly a third of these two blocks were occupied by two schools and church, another third by businesses and apartments. The last third, and the largest single landholder was the F.&M. Schaefer Brewing Co. The brewery fronted Park Avenue and for many years enjoyed having a street-level siding. With the tracks being moved below street level, the brewery's siding was gone. More disturbing, however, was that the drilling vibrations and the close proximity of the railroad excavations to the brewery threatened underground lagering cellars.
Despite the setbacks and danger, The F.&M. Schaefer Brewing Co. did not protest the planned expansion. On Nov. 22, 1902, according to the New York Times, former city controller Ashbel P. Fitch, representing the brewery, stated, " [F.&M. Schaefer Brewing Co. will] not stand in the way of any great public improvement, and that although they had already suffered by the closing of streets, they were willing to suffer more for the public good."
Ultimately, Schaefer Brewing closed the Manhattan plant, sold the incredibly valuable land, and built a new brewery in Brooklyn.
No. 5 - Late to its own funeral
Into the 1950s, the 20th Century Limited, like other passenger trains was in decline. The allure of faster jetliners and the freedom of the automobile had taken its toll. The last run of the Century was announced for Dec. 2, 1967. The announcement really wasn't a big announcement, but rather a whisper in an attempt to reduce the fanfare around the great train's demise.
Only half full, the Century departed from Grand Central Terminal track 34 at 6 p.m. The fanfare was minimal: a group of railfans gathered on the famous red carpet, taking photos of observation-lounge Wingate Brook, which brought up the rear of the train. Beyond that was the press, taking photos of the railfans. Aboard the train, despite the crew putting on a good face, the equipment was worn, and it was clearly the final trip.
In the dark of night, west of Harbor Creek, Pa., another train had derailed, blocking the Century's path. The train sat still for hours until it was detoured over the Norfolk & Western. In the end, the last run of the 20th Century Limited limped into Chicago around 6:45 p.m. - 9 hours, 50 minutes overdue - late to its own funeral.

As he flirts with .400, here are 7 Steven Kwan stats bound to blow your mind

Remember the last time Steven Kwan played an entire game and failed to record a base hit? Remember that, on May 2 in Houston, a mere seven weeks ago?
OK, sure, Kwan was sidelined for four weeks with a hamstring strain during that stretch, but all he’s done since he returned from the injured list — and, really, all he did before he landed on the injured list — is hit. And hit some more. And hit some more.
Advertisement
He’s flirting with the vaunted .400 mark, and not an eye-contact-from-across-the-bar type of flirting. There might be something here, something that can last. No big-leaguer has hit .400 since the ’40s. It’s not even fair to Kwan to surmise whether he can maintain this otherworldly pace for another few months. But he’s certainly positioning himself to have one of the more memorable hitting seasons in recent or semi-recent Cleveland history.
With a four-hit performance in Toronto on Sunday, Kwan boosted his average to .398. But the statistics that are difficult to wrap your head around extend far beyond his batting average. Let’s dig deeper.
His lead for a batting title is massive
Kwan is 70-for-176 this season, hitting .398, but he’s about 18 plate appearances short of qualifying for a batting title. He should make up enough ground in the next few weeks to join the leaderboards. And when he does, he’ll almost assuredly sit at the top in batting average.
Bobby Witt Jr. is the leader, at .327. For Kwan to fall below .327, he would have to go hitless in his next 39 at-bats. Thirty-nine!
And, well, that seems unlikely, considering …
Kwan’s longest hitless streak this season is seven plate appearances
The only time he’s had back-to-back hitless games: April 21 and 23 (with an off day in between). In the second game, he went 0-for-2 with a pair of walks.
Odds are, Kwan will cool off at some point. Can he hit .350 or better? Here’s the list of Cleveland players (minimum 400 plate appearances) to do that in the last 75 years:
Manny Ramirez, 2000: .351 Albert Belle, 1994: .357 Tito Francona, 1959: .363
The last Cleveland player to hit at least .365: Earl Averill, who hit .378 in 1936.
He’s the first player in 23 years with 70 hits in his first 43 games
Ichiro Suzuki is the only other player in the 21st century to tally that many base knocks in his first 43 games of a season. Kwan is one of seven players to accomplish the feat in the last 50 seasons.
The only player in franchise history, which dates to 1901, with more hits in his first 43 games of a season? Roy Weatherly, a 21-year-old rookie who totaled 73 hits in that span after being called up at the end of June during the 1936 season.
Kwan has more three-hit games than zero-hit games
Three-hit games: 11 Zero-hit games: seven
In one of those seven, he exited in the fourth inning with the aforementioned hamstring injury. Apparently, that injury gave him superpowers, because …
Since returning from the injured list, Kwan is batting .535 in 11 games
He’s 23-for-43, and he has six walks, four doubles and only three strikeouts.
In those 11 games:
Four-hit games: one Three-hit games: three Two-hit games: three One-hit games: four Zero-hit games: ha, yeah right
Kwan has three strikeouts in his last 125 plate appearances
Luis Arraez and Kwan boast the two best strikeout rates in the league, and there’s a huge gap between them and the rest of the field. They’re the only ones with a rate below than 10 percent.
To put Kwan’s recent stretch into perspective, Reds outfielder Will Benson, his close friend and former teammate, owns the league’s worst strikeout rate this season. Benson has 49 strikeouts in his last 125 plate appearances.
Overall, Kwan has 14 strikeouts in 196 plate appearances.
Kwan’s strikeout rate, per year:
2022: 9.4 percent 2023: 10.4 percent 2024: 7.1 percent
He has nearly as many three-hit games (11) as strikeouts (14) this season.
He’s on a record-setting-Ichiro-esque hit pace
Hits per game by Kwan in 2024: 1.6279 Hits per game by Ichiro in 2004: 1.6273
That’s the year Ichiro — one of Kwan’s idols — set a major-league record with 262 hits, surpassing George Sisler’s mark that stood for 84 years. Ichiro appeared in all but one game that season for the 63-99 Mariners and posted a .372/.414/.455 slash line, slapping singles across the diamond on a nightly basis.
He had only 37 extra-base hits.
His 225 singles smashed Willie Keeler’s record of 206, set in 1898. In fact, Ichiro owns three of the top six single-season singles totals, along with a pair of Keeler seasons, plus one from Lloyd Waner in 1927.
Another way to frame this: Singles accounted for 85.9 percent of Ichiro’s hits in 2004. Singles account for 77.1 percent of Kwan’s hits this season, which explains, in part, why his slugging percentage sits 90 points higher than Ichiro’s did 20 years ago.
Ichiro’s ability to sustain that hitting prowess across an entire season, without ever spending time on the injured list, was admirable. Kwan has another three and a half months to torment pitchers and then perhaps we can compare these seasons a bit more closely.
(Photo of Steven Kwan: Andy Lyons / Getty Images)
Get all-access to exclusive stories.
Subscribe to The Athletic for in-depth coverage of your favorite players, teams, leagues and clubs. Try a week on us.

Zack Meisel is a senior writer for The Athletic covering the Cleveland Guardians and Major League Baseball. Zack was named the 2021 Ohio Sportswriter of the Year by the National Sports Media Association and won first place for best sports coverage from the Society of Professional Journalists. He has been on the beat since 2011 and is the author of four books, including "Cleveland Rocked," the tale of the 1995 team. Follow Zack on Twitter @ ZackMeisel

An official website of the United States government
The .gov means it’s official. Federal government websites often end in .gov or .mil. Before sharing sensitive information, make sure you’re on a federal government site.
The site is secure. The https:// ensures that you are connecting to the official website and that any information you provide is encrypted and transmitted securely.
- Publications
- Account settings
Preview improvements coming to the PMC website in October 2024. Learn More or Try it out now .
- Advanced Search
- Journal List

Working memory capacity and (in)voluntary mind wandering
Alexander soemer.
Department of Psychology, University of Potsdam, Karl-Liebknecht-Str. 24-25, 14476 Potsdam, Germany
Ulrich Schiefele
Associated data.
According to influential accounts of mind wandering (MW), working memory capacity (WMC) plays a key role in controlling the amount of off-task thought during the execution of a demanding task. Whereas WMC has primarily been associated with reduced levels of involuntarily occurring MW episodes in prior research, here we demonstrate for the first time that high-WMC individuals exhibit lower levels of voluntary MW. One hundred and eighty participants carried out a demanding reading task and reported their attentional state in response to random thought probes. In addition, participants’ WMC was measured with two common complex span tasks (operation span and symmetry span). As a result, WMC was negatively related to both voluntary and involuntary MW, and the two forms of MW partially mediated the positive effect of WMC on reading performance. Furthermore, the negative relation between voluntary WM and reading remained significant after controlling for interest. Thus, in contrast to prior research suggesting that voluntary MW might be more closely related to motivation rather than WMC, the present results demonstrate that high-WMC individuals tend to limit both involuntary and voluntary MW more strictly than low-WMC individuals.
Electronic supplementary material
The online version of this article (10.3758/s13423-020-01737-4) contains supplementary material, which is available to authorized users.
Most of us have experienced situations in which our thoughts drifted off while carrying out potentially important tasks, such as proofreading a manuscript or making a tax declaration. Although content, emotional valence, as well as temporal focus of such task-unrelated thoughts can be quite diverse, two common characteristics are that these thoughts are not directly related to any given primary activity and that they originate from one’s internal stream of thoughts rather than being cued by external events (Smallwood & Schooler, 2015 ). 1
Task-unrelated thoughts have received a great deal of interest in recent psychological research, where they are also being referred to as mind wandering (MW). Part of this recent interest follows from the ubiquity of MW in daily life (e.g., Killingsworth & Gilbert, 2010 ; Unsworth, Brewer, & Spillers, 2012 ) and its potential impact on our actions. In particular, MW seems to have a negative impact on task performance (e.g., McVay & Kane, 2012 ; Soemer & Schiefele, 2019 ), and it is frequently accompanied by negative emotions (Killingsworth & Gilbert, 2010 ) and cognitive failures (Unsworth et al., 2012 ).
An important question for MW researchers is why certain individuals more than others frequently engage in MW while carrying out a given primary task. Related to this question, prior research has highlighted the role of working memory as a key predictor of MW (Kane & McVay, 2012 ). Working memory is thought to underlie a wide variety of cognitive skills, and individual differences in working memory capacity (WMC) are a major predictor for performance in a broad range of tasks (Kane, Bleckley, Conway, & Engle, 2001 ). According to an influential working memory theory, individual differences in WMC reflect variation in a domain-general executive control capability that is critical for maintaining one’s focus of attention on a primary task and inhibit distraction from both external and internal sources (Kane et al., 2001 ). Based on this account of working memory, some researchers have argued that the occurrence of MW reflects failures of executive control, whereby personal concerns of an individual overtake the focus of attention and disrupt execution of the primary task (Kane & McVay, 2012 ; McVay & Kane, 2012 ). In support of this view, it has been observed that high-WMC individuals report fewer MW episodes than low-WMC individuals while carrying out a demanding task, and this difference partially explains their better task performance (e.g., McVay & Kane, 2012 ; Robison & Unsworth, 2018 ).
The control failures account has successfully been used to explain the relations between WMC, MW, and task performance in demanding tasks (e.g., reading comprehension) based on the assumption that individuals normally seek to avoid and suppress MW episodes to successfully perform the tasks; that is, the control failures account considers MW to be something that happens despite one’s best effort to focus on the primary task (Kane & McVay, 2012 , pp. 348–349).
However, both personal experience and research suggest that there are situations in which an individual freely chooses to engage in MW instead of focusing on the primary task. This may happen, for example, because one does not expect MW to have a negative impact on task performance, because a task is too demanding or because of a lack of motivation to perform the task (Seli, Cheyne, Xu, Purdon, & Smilek, 2015 ; Seli, Risko, & Smilek, 2016 ). Taking into consideration such cases of MW, some researchers have argued for making a distinction between “voluntary” and “involuntary” MW (e.g., Seli et al., 2016 ). 2 Here, voluntary MW is defined as some kind of MW that an individual freely chooses to engage in, whereas involuntary MW occurs despite an individual’s best effort to focus on the primary task.
Highlighting the importance of making this distinction, Seli et al. ( 2015 ) reported a significant negative correlation between the motivation to perform well on a task and a measure of voluntary (but not involuntary) MW suggesting that one cause for voluntary MW could be low motivation. Robison and Unsworth ( 2018 ) replicated this negative association and, furthermore, found an inverse association between involuntary (but not voluntary) MW and a measure of alertness. Finally, Seli et al. ( 2016 ) found voluntary and involuntary MW to be differently affected by task difficulty manipulations: Whereas voluntary MW was more frequent when participants carried out an easy version of the Sustained Attention to Response (SART) task compared with a more difficult version, the opposite was true for involuntary MW.
Although prior research suggests that it may be useful to distinguish between voluntary and involuntary MW, it is still not clear how WMC may be related to these two forms of MW. On the one hand, studies on the relation between WMC and MW have so far rarely distinguished between voluntary and involuntary MW and instead implicitly or explicitly characterized MW as involuntary (e.g., Kane & McVay, 2012 , p. 348). On the other hand, in those cases where the distinction was made, the resulting evidence is inconclusive. For example, Soemer and Schiefele ( 2019 ) found a significant negative association between WMC and involuntary MW, but a nonsignificant association between WMC and voluntary MW with a reading task. The same pattern was found in a study by Robison and Unsworth ( 2018 ) with a number of attention-demanding laboratory tasks. Finally, Ju and Lien ( 2018 ) reported a significant negative relation between involuntary MW and WMC in a demanding version of a modified N -back task but a nonsignificant relation in a nondemanding version of this task. Importantly, there was no statistically significant relation between voluntary MW and WMC in both task conditions.
Although one might be tempted to regard such nonsignificant results as support for the absence of a (measurable) relation between voluntary MW and WMC, it must be noted that statistically nonsignificant results, even if repeatedly demonstrated, generally do not constitute evidence in favor of a null hypothesis (e.g., Nickerson, 2000 ). For this reason, none of the aforementioned experiments should be taken as evidence for the hypothesis that voluntary MW and WMC are completely unrelated. And also, on theoretical grounds, there are reasons to believe that voluntary MW and WMC might be more strongly related than current evidence suggests. First, low-WMC individuals are known to experience more difficulties while carrying out demanding tasks (e.g., Swanson, Zheng, & Jerman, 2009 ), and they should therefore be more inclined to skip (difficult) parts of the task, rest, or even give up completely at some point. As a consequence, low-WMC individuals should exhibit a stronger tendency to deliberately start thinking about something unrelated to the task or continue a MW episode that originally started as an involuntary MW episode (i.e., due to an executive control failure).
Second, low-WMC individuals may generally be less motivated to engage or persist in demanding tasks because they do not expect themselves to be good at it (e.g., Linnenbrink & Pintrich, 2003 ). As a consequence of such low self-efficacy beliefs, they may not invest much of their attentional capacity in a demanding task right from the beginning and instead allow for more voluntary MW.
Third, prior research suggests that low-WMC individuals are less accurate with regard to meta-cognitive judgments of their performance in demanding tasks (e.g., Komori, 2016 ). Based on such results, one may speculate that low-WMC individuals more easily misjudge the impact of MW on task performance, and may therefore tolerate more voluntary MW compared with high-WMC individuals. Put differently, high-WMC individuals may more strictly limit voluntary MW because they are more aware of the negative consequences on task performance.
These three considerations lead to predicting a negative relation between WMC and voluntary MW, given a suitable experimental paradigm and appropriate instruments for measuring both constructs. However, a number of factors might contribute to the failure to demonstrate such a relation. First, some of the aforementioned studies used single-task measures of WMC (Ju & Lien, 2018 ; Soemer & Schiefele, 2019 ), raising the possibility that variance related to individual differences in WMC was masked by a large amount of error variance, which, in turn, led to an underestimation of the correlations between WMC and voluntary MW. Second, some of the studies report very low rates of voluntary MW (e.g., between 2% and 4% in Robison & Unsworth, 2018 ), suggesting floor effects that, again, make it difficult to detect significant correlations between voluntary MW and WMC. Third, the studies have used various tasks with different cognitive demands, a variable that likely moderates the relation between WMC and voluntary MW. 3 In one study, for example, voluntary MW was measured during the execution of three different laboratory tasks that later showed correlations between voluntary MW and measures of WMC ranging from −.17 to .11 (Robison & Unsworth, 2018 ). In another case, task difficulty was manipulated within a single task (reading), and this manipulation led to low rates of voluntary MW in the easier conditions (Soemer & Schiefele, 2019 ) meaning, again, that it was difficult to demonstrate a significant correlation between voluntary MW and WMC.
The current study
This study set out to investigate the associations between WMC and voluntary and involuntary MW, avoiding the aforementioned pitfalls of prior research and hypothesizing that WMC would be negatively related to both forms of MW for the above outlined reasons. To gather support for this hypothesis, we first measured participants’ WMC with two common complex span tasks—operation span and symmetry span (Conway et al., 2005 ) and then estimated participants’ individual MW rates during the execution of a demanding primary task. For the present purpose, reading comprehension was our task of choice because of its high ecological validity, its moderate to high difficulty, its frequent use in MW research (e.g., McVay & Kane, 2012 ; Soemer & Schiefele, 2019 ; Unsworth & McMillan, 2013 ), and because comparisons could be made with prior research (Soemer & Schiefele, 2019 ). Probe-caught experience sampling was used to estimate individual MW rates: Participants were randomly interrupted during reading and asked to indicate whether they had been focusing on the text or engaging in voluntary or involuntary MW prior to the interruption. After reading each text, participants answered comprehension questions. Because MW rates during reading and comprehension usually depend on prior experience with and interest in the topic of a text (e.g., Soemer & Schiefele, 2019 ), we included measures of topic interest and topic familiarity to be used as control variables in later modeling.
Based on prior research (e.g., McVay & Kane, 2012 ; Soemer & Schiefele, 2019 ; Unsworth & McMillan, 2013 ), we predicted that WMC would support comprehension, that WMC would be negatively related to involuntary MW, and that involuntary MW would partially mediate the relation between WMC and comprehension (e.g., Soemer & Schiefele, 2019 ). The most important prediction, however, concerned the relation between WMC and voluntary MW. We expected this relation to be negative, (1) because we assumed that low-WMC individuals experience more difficulties during reading and therefore more easily skip parts of the text, rest, or give up completely; (2) because they may generally invest less in a task that they believe they are not good at (low self-efficacy beliefs); or (3) because they more easily misjudge the impact of MW on comprehension. Furthermore, we predicted voluntary MW to negatively affect comprehension (cf. Soemer & Schiefele, 2019 ) and a partial mediation of the effect of WMC on comprehension through voluntary MW.
Because reading was selected as the primary task in this study, we included the control variables interest and topic familiarity, and we predicted these variables to be positively related to comprehension (Robison & Unsworth, 2018 ; Soemer & Schiefele, 2019 ; Unsworth & McMillan, 2013 ). Furthermore, we predicted negative effects of interest on both forms of MW, in line with prior research (Soemer & Schiefele, 2019 ). As regards the association between interest and topic familiarity, we predicted a strong positive relation because one usually reads more often about topics that are perceived as interesting (e.g., Alexander, Kulikowich, & Jetton, 1994 ; Soemer & Schiefele, 2019 ) and because more familiar topics promote the perception of interest (Schraw, Flowerday, & Lehman, 2001 ). Finally, we predicted a negative relation between topic familiarity and both forms of MW, although prior research suggests that this relation can be small when interest is controlled for (Soemer & Schiefele, 2019 ). A visual summary of the predictions can be found in Fig. Fig.1 1 .

Structural equation model corresponding to our hypotheses. “+” and “–” indicate the to-be-expected signs of the coefficients
In the following, we will report how we determined our sample size, all data exclusions (if any), all manipulations, and all measures in the study (Simmons, Nelson, & Simonsohn, 2012 ). The experiment was not preregistered. However, the data and analysis scripts are openly available ( https://osf.io/3hdwq/ ).
All participants were tested individually in a soundproof booth at our lab. At the beginning of the experiment, they received brief instructions by a trained experimenter regarding the tasks that they carried out during the following 90-minutes session. Detailed instructions were given prior to each individual task on the computer screen. The instructions for the reading task not only emphasized that participants were going to take a comprehension test after having read each text but also contained a short paragraph explaining to them that they would be interrupted from time to time during reading and asked about their thoughts prior to the interruption (see the Appendix ). To ensure that the participants had understood the instructions, they were allowed to ask questions at the beginning of the session and between each task.
Participants
One hundred and eighty participants were recruited from the university’s student population ( M age = 23.35 years, SD = 3.93 years, 155 females, 25 males). This sample size was set a priori based on our experience with earlier studies and the fact that several measures were taken to improve estimation of the to-be-measured variables compared with our previous study (Soemer & Schiefele, 2019 ). There were no additional stopping rules for data collection, which continued over the course of two academic terms until the targeted sample size was reached. 4 None of the participants had previously participated in any reading or MW experiments at our department, and we therefore assume that all participants were naïve with regard to the purpose of this experiment. After completion of the experiment, they were rewarded with either 12€ or course credits.
Instruments
Working memory capacity.
Participants carried out two commonly used complex span tasks (Conway et al., 2005 ): operation span and symmetry span. Both tasks required participants to maintain a set of items while making true/false judgments on a separate set of items. The two parts of the tasks were interleaved such that participants first saw a to-be-stored item (900 ms on, 100 ms off), then saw a second item on which they made a true/false judgment via button press (2,000 ms time-out), then saw the next to-be-stored item, and so on. The alternation of storage and processing episodes ended after three to seven cycles. Participants were then requested to recall the stored items in their correct serial order. The recall score was derived by dividing the number of items recalled in the correct position of the presented sequence by sequence length (partial credit scoring; see Conway et al., 2005 ).
The major difference between the two span tasks concerned the nature of the items. In the operation-span task, the to-be-stored items consisted of single monosyllabic letters, whereas the processing episodes consisted of math equations taking the form (a ± b) × c = d . Participants had to remember the letters and verify the correctness of the equations in between letter presentation. At recall, both letters that were part of the sequence and letters that were not, but belonged to the item pool, appeared on the screen. Participants had to recall the letter sequence by clicking on the letters one after another. In the symmetry-span task, the to-be-stored items consisted of square locations in a 4 × 4 grid, whereas the processing task required them to judge the vertical symmetry of 32 × 32 matrix patterns. At recall, an empty grid appeared, and participants had to recall the sequence of locations by clicking on the corresponding cells of the grid.
Prior to each task, participants were instructed to perform the processing part as quickly and as accurately as possible. Furthermore, we measured baseline processing speed for the equation and symmetry verification tasks within a single-task condition prior to administering the main tasks. This was done to check whether individuals strategically slow down their processing speed in the later dual-task trials. Processing times deviating more than two standard deviations from the mean times in the single-task trials were taken as an implicit filter criterion for the dual-task trials. Whenever a participant exceeded this individual criterion in a trial, a message appeared on the screen after recall, reminding the participant to perform the processing task as quickly and as accurately as possible. In addition, trials with processing accuracies below 80% were not considered in the later analyses, because this suggests that participants did not sufficiently focus on the processing part of the task (Conway et al., 2005 ).
Prior to the main session, participants were given the opportunity to practice the memory and processing parts both separately and together. In the main session, all participants completed four trials of different sequence lengths, starting with the three items and progressing up to the seven items. Final span scores were calculated by averaging across trials.
Reading comprehension
After completing the complex span tasks, participants read three texts on a computer screen and answered comprehension questions after each text. The texts were taken from the study of Soemer and Schiefele ( 2019 ) and dealt with (1) the physics behind global airflows, (2) stock market bubbles, and (3) semantic knowledge representation. They were edited to be of moderate readability as measured with the readability index ‘LIX’ (Björnsson, 1968 ). The texts were between 913 and 1,281 words long. Each text was displayed page wise, around 150 to 250 words per page, and typed in a dark gray serif font on a white background. To make reading as natural as possible, participants were allowed to freely navigate through the pages by pressing the left-arrow and right-arrow keys on a keyboard. There was no time limit for reading a single text. However, all three texts had to be completed within the overall session limit of 90 minutes.
Each comprehension test consisted of nine 4-item multiple-choice questions per text and was administered after reading (and after rating interest and topic familiarity). Participants were allowed to take as much time as necessary to provide the answers. The test questions were constructed as to measure fact knowledge and required the participants to complete a sentence with one out of four highly similar response options. For example, one test question for the “stock market bubbles” text was “ A central bank can exacerbate speculation on credit by . . . , ” and the four response options were “lending money to private banks at a low interest rate” (correct option), “ lending money to private banks at a high interest rate,” “ lending money to private banks over a short period,” and “ lending money to private banks over a long period.” Each correct answer was assigned 1 point.
Mind wandering
Participants were randomly interrupted during reading after a random interval between 60 to 90 seconds and were required to respond with one of three options: “I was thinking about something related to the text ,” “I was voluntarily thinking about something unrelated to the text ,” and “I was involuntarily thinking about something unrelated to the text . ” Participants were instructed that they should make their response based on whether the thoughts they were engaging in prior to the interruption were aimed at promoting comprehension. The distinction between voluntary and involuntary MW was made clear to them prior to the experiment (see the Appendix ). Because the number of probes that an individual received during reading could vary across participants and texts, individual MW rates per individual and texts were calculated by dividing the responses to the probes by the number of probes.
Participants rated their level of interest on the items “I found this text interesting,” “I like to read about such topics,” and “I found the topics in this text very stimulating.” Ratings were to be provided on a 4-point scale ranging from 0 ( strongly disagree ) to 3 ( strongly agree ).
Topic familiarity
Participants rated their levels of topic familiarity on the items “I have read or heard about these things many times before,” “My prior knowledge helped a lot to understand this text,” and “I am familiar with the topics of this text.” Ratings were to be provided on a 4-point scale ranging from 0 ( strongly disagree ) to 3 ( strongly agree ).
The data of one participant had to be discarded because this participant’s reading times were shorter than 1 minute per text, suggesting that he or she had not properly read the texts. Descriptive and inferential statistics were computed using the data of the remaining 179 participants.
Descriptive statistics
Correlations between the measures were mostly weak to moderate and followed the expected direction (see Table Table1 1 for latent correlations and Table Table4 4 for manifest correlations). In particular, WMC, interest and topic familiarity were positively correlated with comprehension, whereas both forms of MW were negatively correlated with comprehension, interest, and topic familiarity. Most importantly, the correlations between WMC and voluntary/involuntary MW were both negative, as predicted.
Latent bivariate correlations and descriptive statistics for the investigated constructs
Note. WMC = working memory capacity; MW = mind wandering. * p < .05; *** p < .001
Bivariate correlations and descriptive statistics the manifest variables
INT = interest; FAM = topic familiarity; OSPAN = operation span; SSPAN = symmetry span; VOL = voluntary mind wandering; INV = involuntary mind wandering; COM = comprehension. Note that the items COM1-3 represent parcels that were created by grouping together each three items from the multiple-choice test
Structural equation modeling
A structural equation model was subsequently estimated to test the hypotheses shown in Fig. Fig.1. 1 . In this model, WMC, interest, topic familiarity, and comprehension were set up as latent variables. The latent variables referring to the former three constructs were represented at the level of individual items, whereas comprehension was indicated by three item parcels instead of treating each comprehension question as a single indicator (see Little, Cunningham, Shahar, & Widaman, 2002 , for a detailed discussion about the benefits of parceling when there are many indicators for a construct). Voluntary and involuntary MW were modeled as manifest variables. Because interest and topic familiarity were measured on ordinal scales, the corresponding variables were treated as categorical predictors. Finally, to account for the multilevel structure of the data—texts were nested in participants—intercepts were allowed to vary per participant.
The fit of the model was good according to common criteria (χ 2 = 129.718, p < .001, df = 52, Comparative Fit Index (CFI) = .990, Tucker-Lewis Index (TLI) = .986, Root Mean Error of Approximation (RMSEA) = .053), and the signs of the estimated path coefficients corresponded to our predictions (see Table Table2 2 and Fig. Fig.2). 2 ). Most importantly, WMC was positively related to reading comprehension and negatively related to both forms of MW. Furthermore, the positive effect of WMC on comprehension was partially mediated by both forms of MW (see Table Table3). 3 ). These results support the main hypothesis that low-WMC individuals were more likely to engage in voluntary MW and that this partly explains their lower task performance. Furthermore, we replicated prior research showing that high-WMC individuals tend to suppress involuntary MW episodes more strongly than low-WMC individuals, and this explains another part of the variance in task performance. As regards the remaining results, interest was negatively related to both voluntary and involuntary MW, and, furthermore, the effect of interest on comprehension was mediated by both forms of MW (see Table Table3). 3 ). Somewhat surprisingly, topic familiarity did not significantly predict MW or comprehension in this model, despite the earlier reported significant correlations. Taking into account that interest and topic familiarity highly correlated ( r = .55), both variables seemed to explain largely overlapping parts of the variance in MW and reading comprehension, although the two constructs were empirically separable. 5
Standardized coefficients, standard deviations, expected signs of the coefficients, and p values for each path of the estimated model
Note. WMC = working memory capacity; MW = mind wandering

Structural equation model with estimated standardized coefficients. Grey dashed lines indicate nonsignificant paths
Standardized coefficients, standard deviations, expected signs of the coefficients, and p values for the mediator paths in the estimated model
Note. WM = working memory; MW = mind wandering
The current study investigated the relations between WMC, voluntary/involuntary MW, and performance in a reading comprehension task. The most important and novel results in comparison to prior research (Ju & Lien, 2018 ; Robison & Unsworth, 2018 ; Soemer & Schiefele, 2019 ) are (1) that high-WMC individuals reported fewer voluntary MW episodes compared with low-WMC individuals, (2) that the negative relation between WMC and voluntary MW remained after controlling for interest, and (3) that voluntary MW partially mediated the effect of WMC on reading performance. Moreover, we also replicating previous findings showing that involuntary MW impairs reading comprehension and partially mediates the positive effect of WMC on comprehension (e.g., McVay & Kane, 2012 ; Soemer & Schiefele, 2019 ).
Our preferred interpretation of the inverse association between voluntary WM and WMC is that low-WMC individuals experience greater difficulties while extracting facts from a text and integrating them into a coherent mental model (Swanson et al., 2009 ), and that these difficulties may especially encourage low-WMC individuals to skip parts of the text, or give up completely, and deliberately pursue less stressful thoughts instead. Related to this explanation, prior research has demonstrated an inverse association between readability, a measure of objective text difficulty, and voluntary MW (Soemer & Schiefele, 2019 ), suggesting that the more difficult a text, the more likely it becomes that an individual engages in voluntary MW. Here, although our texts were of overall moderate readability, they may have nevertheless been relatively difficult for low-WMC individuals. As a consequence, these individuals may have been more inclined to deliberately start a MW episode or deliberately continue a MW episode that started as an involuntary episode.
An alternative explanation is related to self-efficacy beliefs—that is, an individual’s expectation of being successful in a given task. Prior research suggests that low-WMC individuals have lower self-efficacy beliefs (Linnenbrink & Pintrich, 2003 ), and it may therefore be argued that they are less willing to engage and persist in a task or refocus their attention on the text when they become aware of their (involuntary) MW. Finally, prior research suggests that low-WMC individuals are less accurate with regard to meta-cognitive judgments of their performance in demanding tasks, because they lack the capacity to perform the task and carry out the judgments at the same time (Komori, 2016 ). We suggest that low-WMC individuals may also more easily misjudge the impact of voluntary MW on reading comprehension, compared with high-WMC individuals, and therefore allow more voluntary MW to take place during task execution.
Whereas our results deviate from prior research insofar as we obtained, for the first time, significant effects of WMC on voluntary MW, they do not generally contradict the control failures account of MW (Kane & McVay, 2012 ). To reiterate, this account states that WMC reflects the ability to control the focus of attention and inhibit external and internal distraction. Accordingly, because high-WMC individuals are better at suppressing internal distraction, they report less (involuntary) MW, and this partially explains their better task performance. That being said, the negative relation between WMC and voluntary MW as well as the negative effect of voluntary MW on comprehension were not explicitly predicted by the control failures account, as this account is mainly concerned with involuntarily MW (Kane & McVay, 2012 , pp. 348–349). In line with more recent proposals regarding the distinction between voluntary and involuntary MW (Seli et al., 2015 ; Seli et al., 2016 ), we argue that high-WMC individuals use their better executive control capabilities not only for suppressing involuntary MW episodes but also for limiting their level of voluntary MW whenever this is required for successfully carrying out a given task (Rummel & Boywitt, 2014 ).
Finally, it is also particularly noteworthy that voluntary MW impaired comprehension even though our model controlled for interest, an important motivational variable. Thus, in contrast to prior research arguing that voluntary MW might be more closely related to motivational factors rather than to executive control (Soemer & Schiefele, 2019 ), interest and voluntary MW seemed to explain partially separate parts of variance in reading comprehension. In sum, the present results support the idea that executive control (i.e., WMC) is used to regulate thought content both by suppressing involuntary occurring MW and by limiting voluntary MW.
It may be argued that such conclusions should be drawn with great caution given the number of nonsignificant results in prior studies. Although we have argued earlier that these nonsignificant results do not tell us much about the sign and the strength of the relation between WMC and voluntary MW, it seems nevertheless meaningful to incorporate estimates from prior research into our analysis to address concerns of replicability. We therefore carried out a Bayesian reanalysis of our structural equation model (SEM) using parameter estimates from a similar previous study (Soemer & Schiefele, 2019 ) as priors for our model parameters, and provide the results of this reanalysis as a supplement . To foreshadow the results, we found the Bayesian reanalysis to be broadly consistent with the traditional analysis reported above. In particular, the negative relations between WMC and the two forms of MW shown in the traditional analysis remain statistically reliable in the Bayesian reanalysis.
(DOCX 33 kb)
Appendix: Mind-wandering instructions (approximate translation)
(Slide 1) “Do you recognize this situation? You are sitting in front of your desk reading a text. Your eyes move from left to right, scanning every word, sentence, and paragraph. After some time, however, you start thinking about something that has nothing to do with the text. Perhaps, you don’t notice it immediately; perhaps your eyes are still moving naturally across the text as if you were still focusing on reading. But at some point, you become aware that your thoughts have been drifting off and that you have to reread the previous paragraph.”
(Slide 2) “Every human being experiences such task-unrelated thoughts under certain circumstances while carrying out certain tasks. This is a natural phenomenon called ‘mind wandering’ in psychological research. In this study, we are interested in the role of intention in mind wandering.”
(Slide 3) “Sometimes, we unintentionally drift off, despite our best effort to focus on a task such as reading a text. We call this ‘involuntary mind wandering,’ because it happens although we actually don’t intend to mind wander. Sometimes we drift off deliberately because we don’t want to focus on the task in that specific moment. We call this ‘voluntary mind wandering.’”
(Slide 4) “In this study, we will ask you to read a number of texts dealing with different subject areas. From time to time during reading, we will interrupt you and ask you to indicate whether you were focusing on the text just prior to the interruption or, instead, voluntarily or involuntarily engaging in mind wandering.”
(Slide 5) “When we interrupt you, the following question will appear on the screen:
What were you just thinking about? “(1) About something related to the text”; “(2) About something unrelated to the text (voluntarily)”: “(3) About something unrelated to the text (involuntarily).”
Please answer this question with the keys 1–3. Of course, sometimes there is no clear relation between “related” and “unrelated” thoughts, but the general rule is as follows: If the thoughts you experienced aimed at promoting comprehension, please choose (1). Thoughts that did not aim at promoting text comprehension count as “unrelated to the text” (2, 3).
(Slide 6). “Please be totally honest with regard to the mind wandering reports. There really is no ‘right’ or ‘wrong’ answer.”
1 Of course, the internal stream of thought might itself be influenced by external events.
2 Alternative labels are “intentional” or “deliberate” for voluntary MW, and “spontaneous” or “unintentional” for involuntary MW. For convenience, here we only use the terms “voluntary” and “involuntary” MW.
3 For involuntary forms of MW, there is evidence suggesting that a negative relation with WMC only shows up if a task is sufficiently demanding (e.g., Rummel & Boywitt, 2014 ). This could likewise be the case with voluntary MW.
4 There were 10 instances of no-shows or equipment errors. Any partial data was discarded completely without analysis and a replacement was scheduled to reach the target sample size of 180 in these cases.
5 The fit of a two-factor model with separate factors for ‘interest’ and ‘familiarity’ was substantially better (χ 2 = 52.718, p < .001, df = 8, CFI = .995, TLI = .990, RMSEA = .102) than the fit of a one-factor model (χ 2 = 577.013, p < .001, df = 9, CFI =.933, TLI = .888, RMSEA = .343) or a two-factor model with the correlation between the two factors set to 1.0 (χ 2 = 801.362, p < .001, df = 9, CFI = .910, TLI = .849, RMSEA = .405.
Publisher’s note
Springer Nature remains neutral with regard to jurisdictional claims in published maps and institutional affiliations.
- Alexander PA, Kulikowich JM, Jetton TL. The role of subject-matter knowledge and interest in the processing of linear and nonlinear texts. Review of Educational Research. 1994; 64 (2):201–252. doi: 10.3102/00346543064002201. [ CrossRef ] [ Google Scholar ]
- Björnsson C-H. Läsbarhet [Readability] Stockholm, Sweden: Liber; 1968. [ Google Scholar ]
- Conway ARA, Kane MJ, Bunting MF, Hambrick DZ, Wilhelm O, Engle RW. Working memory span tasks: A methodological review and user’s guide. Psychonomic Bulletin & Review. 2005; 12 :769–786. doi: 10.3758/BF03196772. [ PubMed ] [ CrossRef ] [ Google Scholar ]
- Ju Y-J, Lien Y-W. Who is prone to wander and when? Examining an integrative effect of working memory capacity and mindfulness trait on mind wandering under different task loads. Consciousness & Cognition. 2018; 63 :1–10. doi: 10.1016/j.concog.2018.06.006. [ PubMed ] [ CrossRef ] [ Google Scholar ]
- Kane MJ, Bleckley MK, Conway ARA, Engle RW. A controlled-attention view of working-memory capacity. Journal of Experimental Psychology: General. 2001; 130 (2):169–183. doi: 10.1037//0096-3445.130.2.169. [ PubMed ] [ CrossRef ] [ Google Scholar ]
- Kane MJ, McVay JC. What mind wandering reveals about executive-control abilities and failures. Current Directions in Psychological Science. 2012; 21 (5):348–354. doi: 10.1177/0963721412454875. [ CrossRef ] [ Google Scholar ]
- Killingsworth MA, Gilbert DT. A wandering mind is an unhappy mind. Science. 2010; 330 (6006):330–332. doi: 10.1126/science.1192439. [ PubMed ] [ CrossRef ] [ Google Scholar ]
- Komori M. Effects of working memory capacity on metacognitive monitoring: A study of group differences using a listening span test. Frontiers in Psychology. 2016; 7 :285. doi: 10.3389/fpsyg.2016.00285. [ PMC free article ] [ PubMed ] [ CrossRef ] [ Google Scholar ]
- Linnenbrink EA, Pintrich PA. The role of self-efficacy beliefs in student engagement and learning in the classroom. Reading & Writing Quarterly. 2003; 19 (2):119–137. doi: 10.1080/10573560308223. [ CrossRef ] [ Google Scholar ]
- Little TD, Cunningham WA, Shahar G, Widaman KF. To parcel or not to parcel: Exploring the question, weighing the merits. Structural Equation Modeling. 2002; 9 (2):151–173. doi: 10.1207/S15328007SEM0902_1. [ CrossRef ] [ Google Scholar ]
- McVay JC, Kane MJ. Why does working memory capacity predict variation in reading comprehension? On the influence of mind wandering and executive attention. Journal of Experimental Psychology: General. 2012; 141 (2):302–320. doi: 10.1037/a0025250. [ PMC free article ] [ PubMed ] [ CrossRef ] [ Google Scholar ]
- Nickerson RS. Null hypothesis significance testing: A review of an old and continuing controversy. Psychological Methods. 2000; 5 (2):241–301. doi: 10.1037/1082-989x.5.2.241. [ PubMed ] [ CrossRef ] [ Google Scholar ]
- Robison MK, Unsworth N. Cognitive and contextual predictors of spontaneous and deliberate mind-wandering. Journal of Experimental Psychology: Learning, Memory, and Cognition. 2018; 44 (1):85–98. doi: 10.1037/xlm0000444. [ PubMed ] [ CrossRef ] [ Google Scholar ]
- Rummel J, Boywitt CD. Controlling the stream of thought: Working memory capacity predicts adjustment of mind-wandering to situational demands. Psychonomic Bulletin & Review. 2014; 21 (5):1309–1315. doi: 10.3758/s13423-013-0580-3. [ PubMed ] [ CrossRef ] [ Google Scholar ]
- Schraw G, Flowerday T, Lehman S. Increasing situational interest in the classroom. Educational Psychology Review. 2001; 13 :211–224. doi: 10.1023/A:1016619705184. [ CrossRef ] [ Google Scholar ]
- Seli P, Cheyne JA, Xu M, Purdon C, Smilek D. Motivation, intentionality, and mind wandering: Implications for assessments of task-unrelated thought. Journal of Experimental Psychology: Learning, Memory, and Cognition. 2015; 41 (5):1417–1425. doi: 10.1037/xlm0000116. [ PubMed ] [ CrossRef ] [ Google Scholar ]
- Seli P, Risko EF, Smilek D. On the necessity of distinguishing between unintentional and intentional mind wandering. Psychological Science. 2016; 27 (5):685–691. doi: 10.1177/0956797616634068. [ PubMed ] [ CrossRef ] [ Google Scholar ]
- Simmons, J. P., Nelson, L. D., & Simonsohn, U. (2012). A 21 word solution . Retrieved from https://ssrn.com/abstract=2160588
- Smallwood J, Schooler JW. The science of mind wandering: Empirically navigating the stream of consciousness. Annual Reviews of Psychology. 2015; 66 :487–518. doi: 10.1146/annurev-psych-010814-015331. [ PubMed ] [ CrossRef ] [ Google Scholar ]
- Soemer A, Schiefele U. Text difficulty, topic interest, and mind wandering during reading. Learning and Instruction. 2019; 61 :12–22. doi: 10.1016/j.learninstruc.2018.12.006. [ CrossRef ] [ Google Scholar ]
- Swanson HL, Zheng X, Jerman O. Working memory, short-term memory, and reading disabilities. Journal of Learning Disabilities. 2009; 42 (3):260–287. doi: 10.1177/0022219409331958. [ PubMed ] [ CrossRef ] [ Google Scholar ]
- Unsworth N, Brewer GA, Spillers GJ. Variation in cognitive failures: An individual differences investigation of everyday attention and memory failures. Journal of Memory and Language. 2012; 67 (1):1–16. doi: 10.1016/j.jml.2011.12.005. [ PubMed ] [ CrossRef ] [ Google Scholar ]
- Unsworth N, McMillan BD. Mind wandering and reading comprehension: Examining the roles of working memory capacity, interest, motivation, and topic experience. Journal of Experimental Psychology: Learning, Memory, and Cognition. 2013; 39 (3):832–842. doi: 10.1037/a002966. [ PubMed ] [ CrossRef ] [ Google Scholar ]

IMAGES
VIDEO
COMMENTS
The debate about mind-wandering. On the depressing side of the debate, Matt Killingsworth's Track Your Happiness project concluded that mind-wandering makes us unhappy. His data showed that our ...
Mind-wandering has been linked to crucial parts of our daily lives, including learning, affect, and job productivity. While a number of studies have examined mind-wandering rates in everyday life, an implicit assumption has been that the rate is constant over the course of the day. The current research provides the first evidence suggesting ...
Mind-wandering is thought to be ubiquitous, having been estimated to occur between 30% and 50% of our waking moments. Yet, it is unclear whether this frequency is similar within-task performance contexts and unknown whether mind-wandering systematically increases with time-on-task for a broad range of tasks. We conducted a systematic literature ...
Thus, mind-wandering might be expected to predict flow, but more research is needed to examine this potential relationship and its mediators. Mind-wandering occurs with high frequency, occupying about half of the individuals' waking hours, and is thus, a prevalent and important experience in daily life (Smallwood and Schooler, 2015).
A wandering mind is a forgetful mind: A systematic review on the influence of mind wandering on episodic memory encoding. Philippe Blondé, ... Pascale Piolino, in Neuroscience & Biobehavioral Reviews, 2022. 1.1 Foreword. Mind wandering is one of the fastest-growing cognitive science topics of the last few decades (Callard et al., 2013). The ...
In the literature on mind-wandering, 1 there is an intriguing finding that has been consistently observed: rates of mind-wandering decrease with increasing age (Jordão, Ferreira-Santos, Pinho, & St Jacques, 2019; Maillet & Schacter, 2016).This finding has perplexed researchers because (a) mind-wandering has been commonly construed as an executive-control failure (e.g., McVay & Kane, 2010 ...
But, still, mind-wandering is a constant. Overall, people mind-wander 47% of the time. Perhaps not such a good thing if it relates to unhappiness, To hear more about mind-wandering — and about the importance of studying happiness in general — watch Killingsworth's talk. And after the jump, read several more fascinating studies on the ...
Mind wandering (MW) and mindfulness have both been reported to be vital moderators of psychological wellbeing. Here, we aim to examine how closely associated these phenomena are and evaluate the ...
Mind-wandering refers to moments when this inward focus diverts attention away from the current task-at-hand. Mind-wandering is thought to be ubiquitous, having been estimated to occur between 30% and 50% of our waking moments. Yet, it is unclear whether this frequency is similar within-task performance contexts and unknown whether mind ...
Conscious experience is fluid; it rarely remains on one topic for an extended period without deviation. Its dynamic nature is illustrated by the experience of mind wandering, in which attention switches from a current task to unrelated thoughts and feelings. Studies exploring the phenomenology of mind wandering highlight the importance of its content and relation to meta-cognition in ...
Key points. Mind-wandering is common and almost everyone experiences it. A new meta-analysis integrated data on mind-wandering and emotions from more then 23,000 volunteers. Mind-wandering can ...
As the scientists themselves so succinctly concluded, "A human mind is a wandering mind, and a wandering mind is an unhappy mind." There are significant differences between individuals when it comes to how much time we spend daydreaming. We are all familiar with the image of a child staring out of a classroom window into the distance.
According to Jonathan Schooler of UC, Santa Barbara, your mind is wandering at least 30% of the time when you are doing your normal day-to-day tasks, and in some cases—for example, when driving ...
Minds wander. Some wander more than others, but human ones wander a lot. A much-cited estimate, due to Killingsworth and Gilbert (2010), has it that the awake human mind spends from a third to half its time wandering.That's a big range, a rough estimate, and there are good reasons to be suspicious of it (see Seli et al. 2018).The actual number will likely depend a bit upon the nature of mind ...
Mind wandering is believed to play an important role in generating new ideas, conclusions or insights (also known as "aha! moments"). This is because it can give your mind a break and free it ...
Mind-wandering (MW) as a research topic has received considerable attention over the last several decades. The recent differentiation between spontaneous and deliberate MW has suggested a particular effect of the former on psychopathology; in that increased spontaneous MW may precede mental illness. The present study sought to explore MW as a ...
Recent studies have shown that mind-wandering may play a crucial role in both autobiographical planning and creative problem solving, thus providing at least two possible adaptive functions of the phenomenon. This article reviews these observed costs and possible functions of mind-wandering and identifies important avenues of future inquiry.
The research, by psychologists Matthew A. Killingsworth and Daniel T. Gilbert of Harvard University, is described this week in the journal Science. "A human mind is a wandering mind, and a wandering mind is an unhappy mind," Killingsworth and Gilbert write. "The ability to think about what is not happening is a cognitive achievement that ...
Student's shift of attention away from a current learning task to task-unrelated thought, also called mind wandering, occurs about 30% of the time spent on education-related activities. Its frequent occurrence has a negative effect on learning outcomes across learning tasks. Automated detection of mind wandering might offer an opportunity to assess the attentional state continuously and non ...
Introduction. An extensive empirical literature has demonstrated that adults mind-wander as much as 30-50% of their waking lives, often at considerable expense to ongoing performance and quality of life (Kane et al., 2007; Killingsworth and Gilbert, 2010; Schooler et al., 2011).Mind-wandering is characteristically described as the interruption of task-focus by task-unrelated thought (TUT ...
Mind-wandering. Mind-wandering is loosely defined as thoughts that are not produced from the current task. Mind-wandering consists of thoughts that are task-unrelated and stimulus-independent. [1] [2] This can be in the form of three different subtypes: positive constructive daydreaming, guilty fear of failure, and poor attentional control.
Mind wandering (MW) is a common feature of the human experience occurring when our attention shifts from the task at hand to inner thoughts. MW seems to be often future-oriented and could be used to help people to carry out their planned actions (Prospective Memory PM). Here, we tested the link between MW and the ability to perform PM ...
Letting your mind wander can help you break free from this cycle and focus on more positive and calming thoughts. It's like taking a mental vacation to a peaceful place, where you can relax and ...
Enough denialism: Democrats (and their media enablers) need to face the facts on President Biden's cognitive decline. After The Post reported on Biden wandering away from the pack of G-7 leaders ...
Mind-blowing facts — New York Central passenger trains We are 50-plus years into the Amtrak era, which began on May 1, 1971. A few Amtrak trains still carry the identity of the conveyances they ...
Introduction. An extensive empirical literature has demonstrated that adults mind-wander as much as 30-50% of their waking lives, often at considerable expense to ongoing performance and quality of life (Kane et al., 2007; Killingsworth and Gilbert, 2010; Schooler et al., 2011).Mind-wandering is characteristically described as the interruption of task-focus by task-unrelated thought (TUT ...
Kwan has more three-hit games than zero-hit games. Three-hit games: 11 Zero-hit games: seven. In one of those seven, he exited in the fourth inning with the aforementioned hamstring injury.
Abstract. According to influential accounts of mind wandering (MW), working memory capacity (WMC) plays a key role in controlling the amount of off-task thought during the execution of a demanding task. Whereas WMC has primarily been associated with reduced levels of involuntarily occurring MW episodes in prior research, here we demonstrate for ...